Data-based groundwater quality estimation and uncertainty analysis for irrigation agriculture
Agricultural Water Management(2022)
摘要
Accurate groundwater quality estimation is of great significance for effective irrigation management in agricultural areas. However, due to lack of data, the parameterization of the physical models is restricted for large areas with diverse underlying surface conditions. To obtain effective and convenient estimations of groundwater quality with the most accessible data is urgently needed. In this study, the validity of the data-based models in irrigation water quality index estimation was investigated by only using physical groundwater parameters as inputs. 15 combination scenarios of the physical parameters including temperature, pH, electrical conductivity, and dissolved oxygen were examined by support vector machine (SVM), random forests (RF), artificial neural networks (ANN) and extreme learning machine (ELM) models for total dissolved solids (TDS), potential salinity (PS), and sodium adsorption ratio (SAR) estimation for the Zhangye Basin, northwest China. Performance of the artificial intelligence (AI) models was evaluated according to the coefficient of correlation (R), root mean squared error (RMSE), mean absolute percentage error (MAPE), and Nash-Sutcliffe efficiency (NS). The Monte Carlo (MC) approach was performed to assess the uncertainty of the physical groundwater parameters and the sensitivity of the AI models. The results revealed an input pattern, which emphasized the important role of EC and the improving function of pH, in revealing the nature of data-based irrigation water quality index estimation. Results of the uncertainty analysis also confirmed the prominent superiority and robustness of the SVM, RF and ELM models in producing excellent estimations with only physical parameters as inputs. The developed AI models were valuable in estimating irrigation water quality indexes, thus could help decision makers manage irrigation strategies. By using physical groundwater parameters as input variables, the AI models showed prospects in convenient and cost-effective irrigation water quality index estimating.
更多查看译文
关键词
Groundwater quality,Data-based model,Uncertainty,Irrigated agriculture
AI 理解论文
溯源树
样例
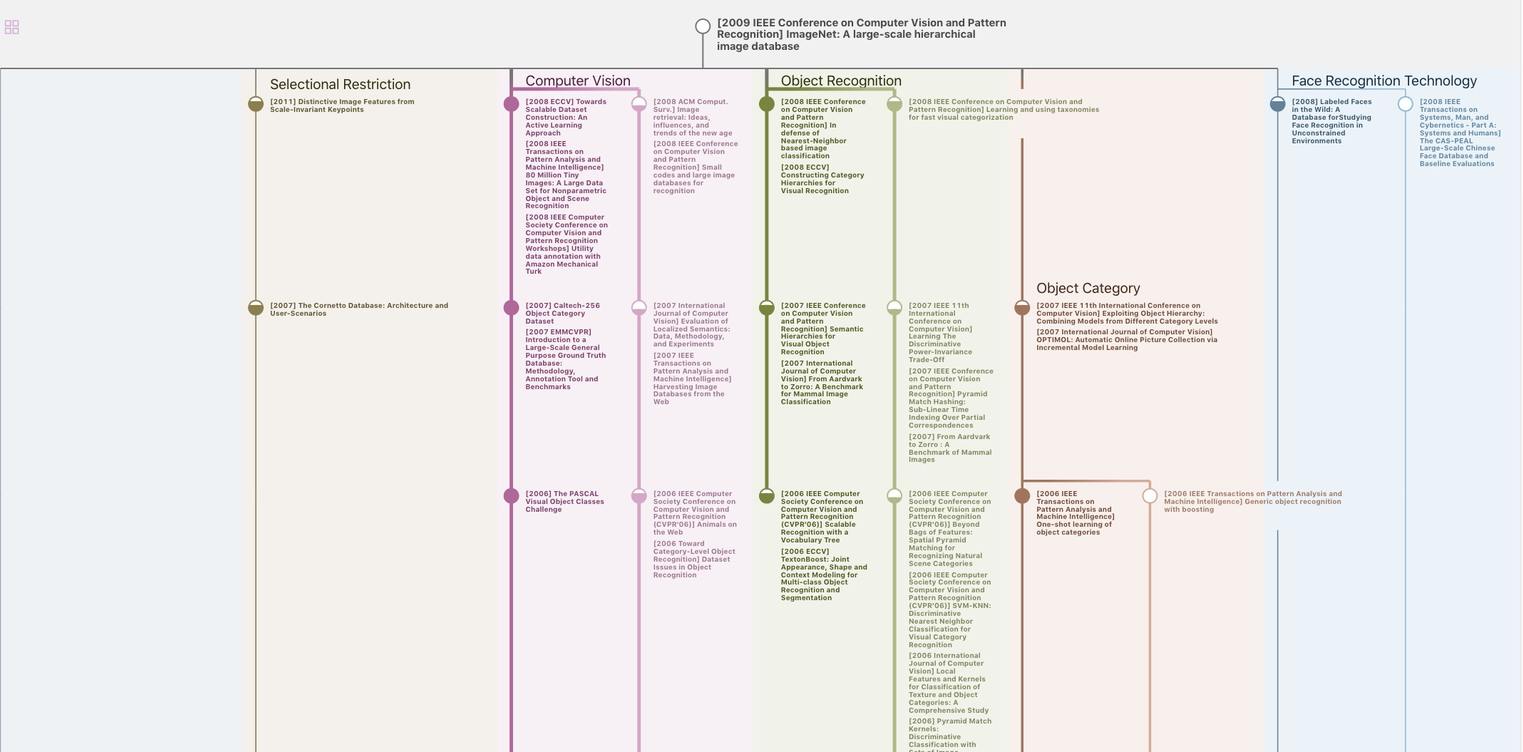
生成溯源树,研究论文发展脉络
Chat Paper
正在生成论文摘要