Crossfire Conditional Generative Adversarial Networks for Singing Voice Extraction.
Interspeech(2021)
摘要
Generative adversarial networks (GANs) and Conditional GANs (cGANs) have recently been applied for singing voice extraction (SVE), since they can accurately model the vocal distributions and effectively utilize a large amount of unlabelled datasets. However, current GANs/cGANs based SVE frameworks have no explicit mechanism to eliminate the mutual interferences between different sources. In this work, we introduce a novel 'crossfire' criterion into GANs to complement its standard adversarial training, which forms a dual-objective GANs, namely Crossfire GANs (Cr-GANs). In addition, we design a Generalized Projection Method (GPM) for cGANs based frameworks to extract more effective conditional information for SVE. Using the proposed GPM, we extend our Cr-GANs to conditional version, i.e., Crossfire Conditional GANs (Cr-cGANs). The proposed methods were evaluated on the DSD100 and CCMixter datasets. The numerical results have shown that the 'crossfire' criterion and GPM are beneficial to each other and considerably improve the separation performance of existing GANs/cGANs based SVE methods.
更多查看译文
关键词
generative adversarial networks,crossfire criterion,generalized projection method,singing voice extraction
AI 理解论文
溯源树
样例
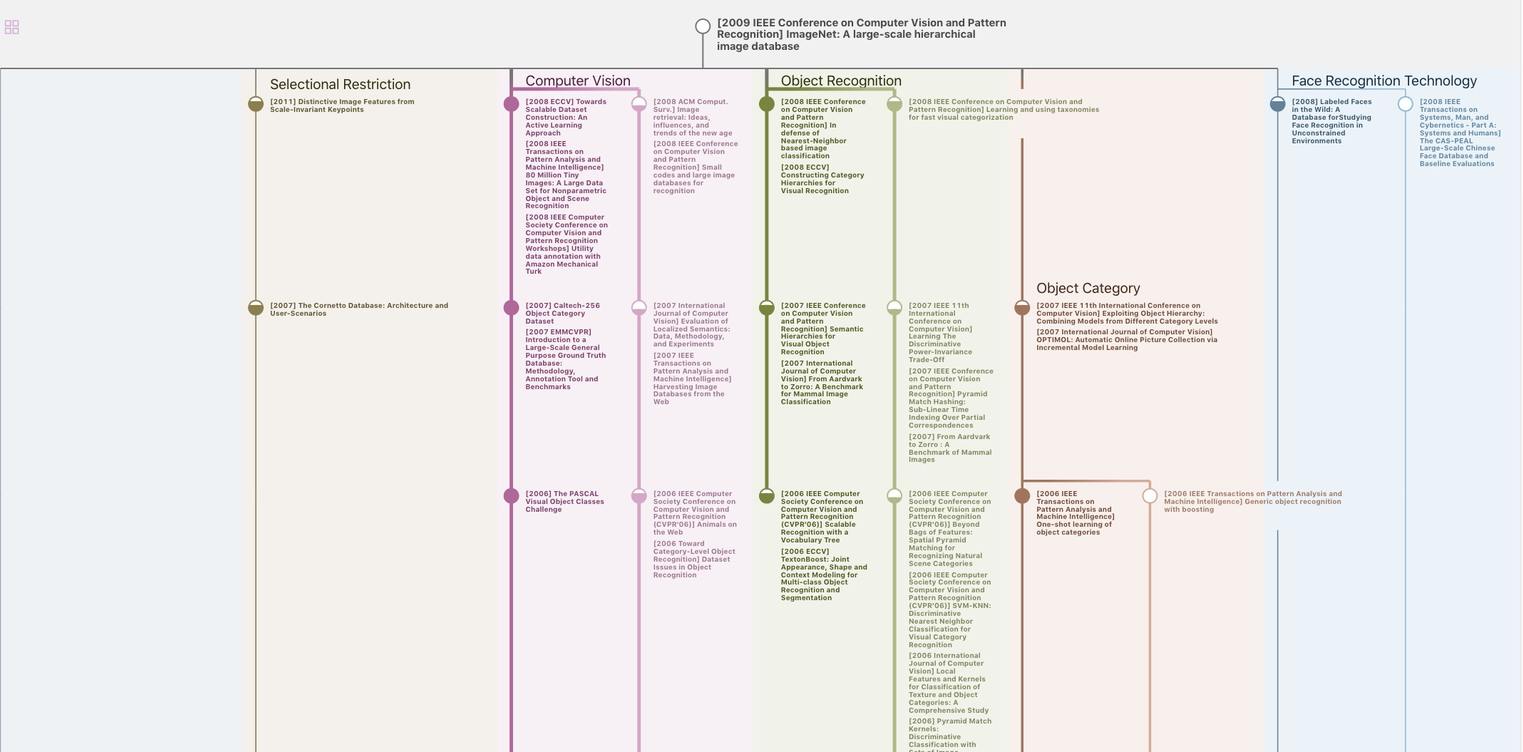
生成溯源树,研究论文发展脉络
Chat Paper
正在生成论文摘要