Temporal Context in Speech Emotion Recognition.
Interspeech(2021)
摘要
We investigate the importance of temporal context for speech emotion recognition (SER). Two SER systems trained on traditional and learned features, respectively, are developed to predict categorical labels of emotion. For traditional acoustical features, we study the combination of filterbank features and prosodic features and the impact on SER when the temporal context of these features is expanded by learnable spectro-temporal receptive fields (STRFs). Experiments show that the system trained on learnable STRFs outperforms other reported systems evaluated with a similar setup. We also demonstrate that the wav2vec features, pretrained with long temporal context, are superior to traditional features. We then introduce a novel segment-based learning objective to constrain our classifier to extract local emotion features from the large temporal context. Combined with the learning objective and fine-tuning strategy, our top-line system using wav2vec features reaches state-of-the-art performance on the IEMOCAP dataset.
更多查看译文
关键词
speech emotion recognition,deep neural networks,prosodic features,wav2vec,learnable spectro-temporal receptive fields
AI 理解论文
溯源树
样例
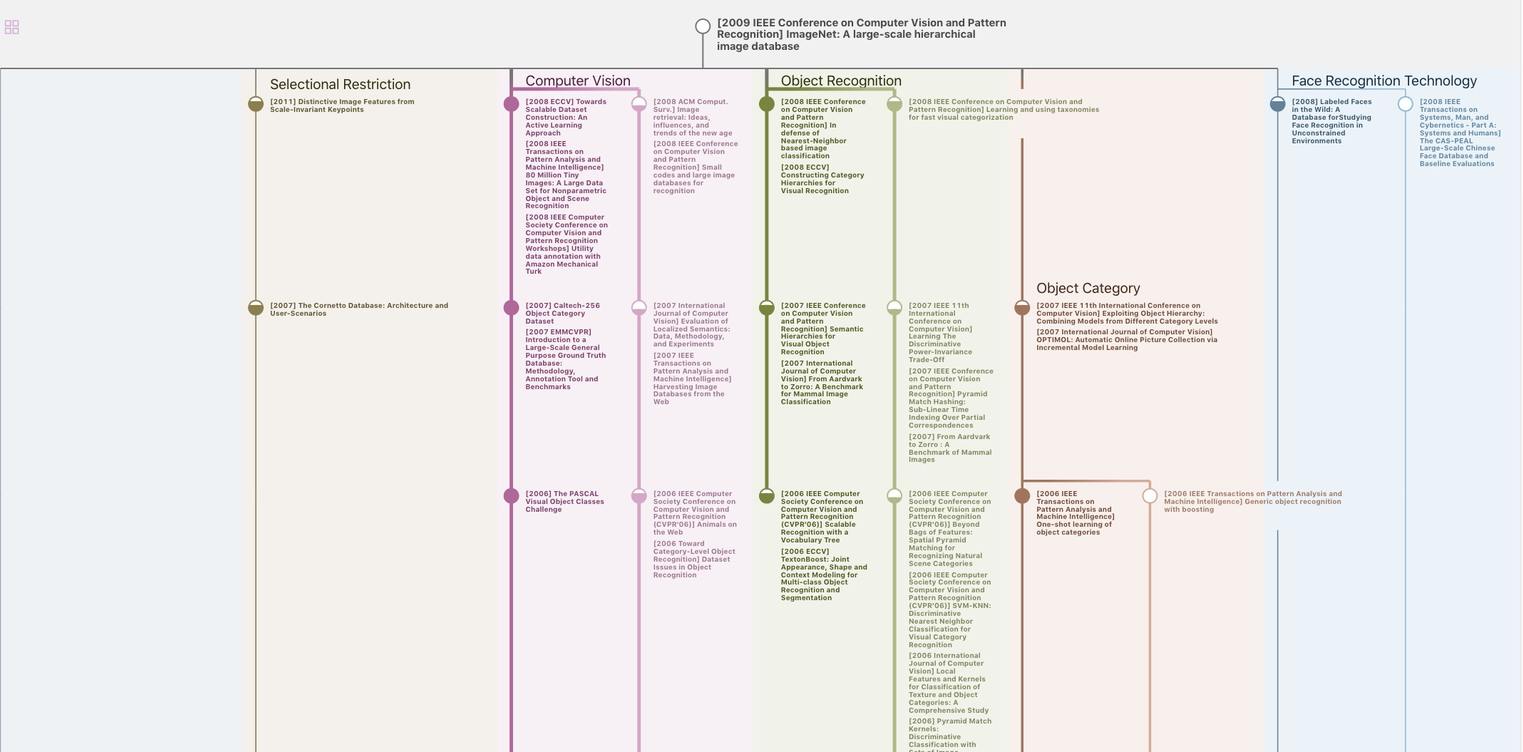
生成溯源树,研究论文发展脉络
Chat Paper
正在生成论文摘要