Phoneme-to-Audio Alignment with Recurrent Neural Networks for Speaking and Singing Voice.
Interspeech(2021)
摘要
Phoneme-to-audio alignment is the task of synchronizing voice recordings and their related phonetic transcripts. In this work, we introduce a new system to forced phonetic alignment with Recurrent Neural Networks (RNN). With the Connectionist Temporal Classification (CTC) loss as training objective, and an additional reconstruction cost, we learn to infer relevant per-frame phoneme probabilities from which alignment is derived. The core of the neural architecture is a context-aware attention mechanism between mel-spectrograms and side information. We investigate two contexts given by either phoneme sequences (model PHATT) or spectrograms themselves (model SPATT). Evaluations show that these models produce precise alignments for both speaking and singing voice. Best results are obtained with the model PHATT, which outperforms baseline reference with an average imprecision of 16.3ms and 29.8ms on speech and singing, respectively. The model SPATT also appears as an interesting alternative, capable of aligning longer audio files without requiring phoneme sequences on small audio segments.
更多查看译文
关键词
phoneme-to-audio alignment,recurrent neural network,Connectionist Temporal Classification,voice analysis
AI 理解论文
溯源树
样例
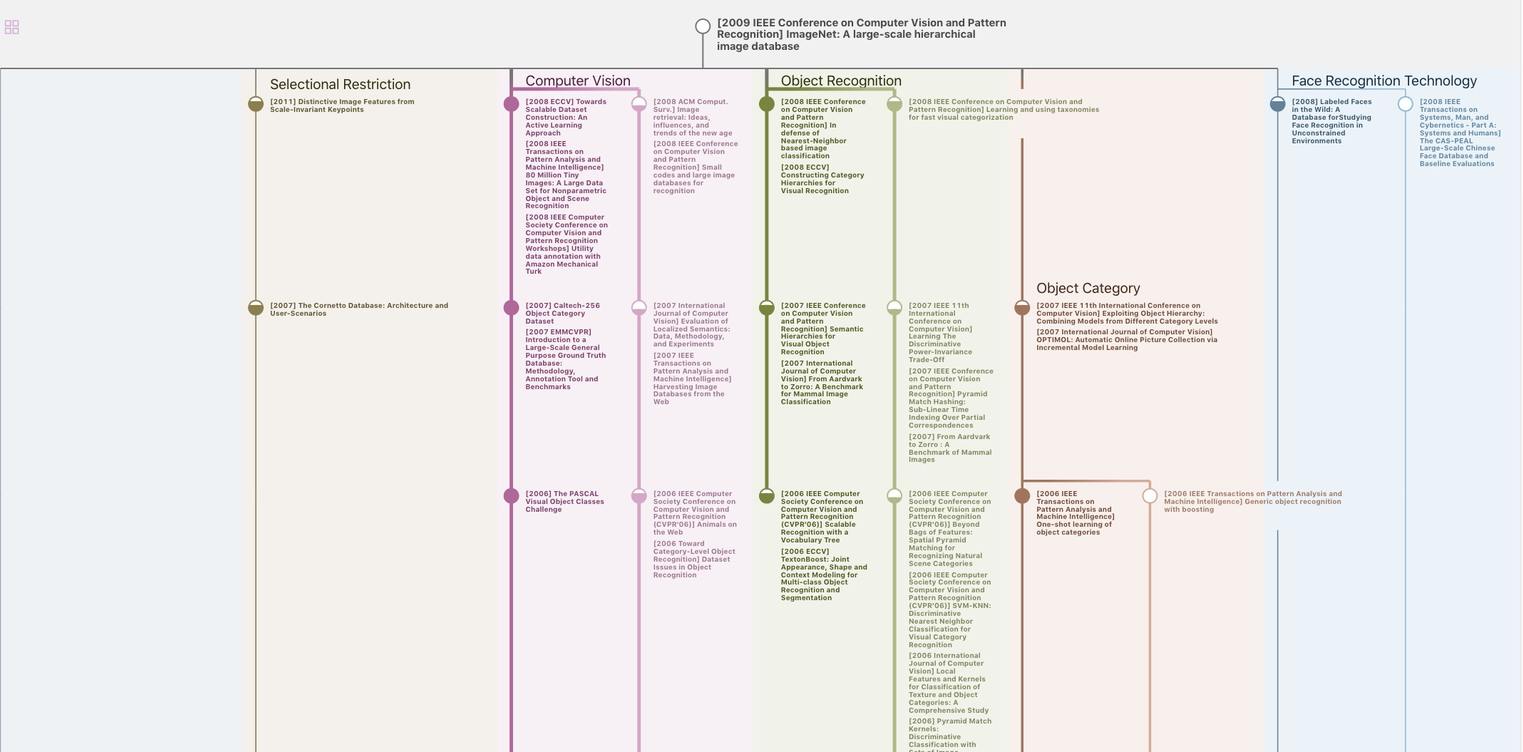
生成溯源树,研究论文发展脉络
Chat Paper
正在生成论文摘要