Predicting Temporal Performance Drop of Deployed Production Spoken Language Understanding Models.
Interspeech(2021)
摘要
In deployed real-world spoken language understanding (SLU) applications, data continuously flows into the system. This leads to distributional differences between training and application data that can deteriorate model performance. While regularly retraining the deployed model with new data helps mitigating this problem, it implies significant computational and human costs. In this paper, we develop a method, which can help guiding decisions on whether a model is safe to keep in production without notable performance loss or needs to be retrained. Towards this goal, we build a performance drop regression model for an SLU model that was trained offline to detect a potential model drift in the production phase. We present a wide range of experiments on multiple real-world datasets, indicating that our method is useful for guiding decisions in the SLU model development cycle and to reduce costs for model retraining.
更多查看译文
AI 理解论文
溯源树
样例
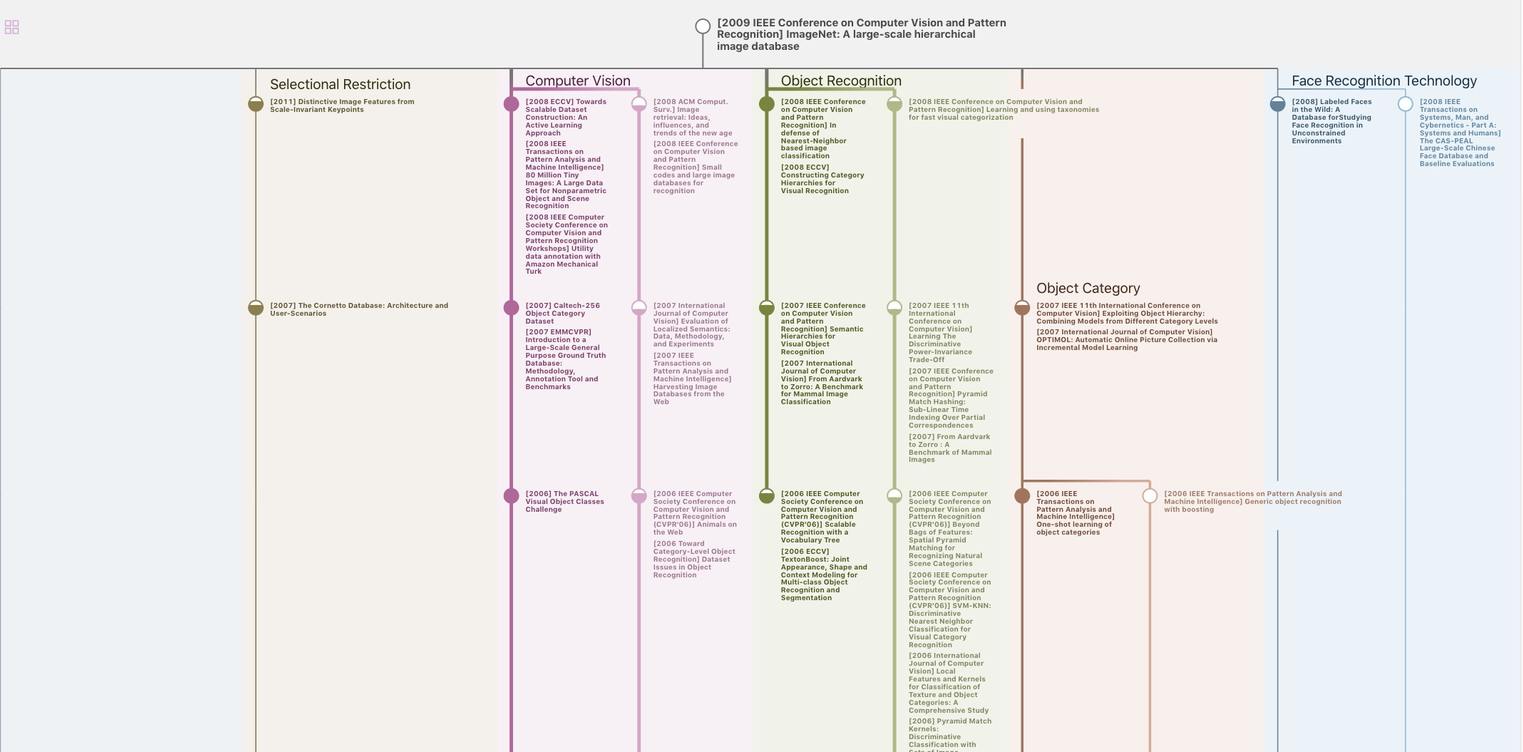
生成溯源树,研究论文发展脉络
Chat Paper
正在生成论文摘要