Bayesian Nonparametric Derivation of Spawning in Multi-Object Tracking - from Association to Tracking.
ACSCC(2021)
摘要
In tracking multiple objects, it is often assumed that each observation (measurement) is originated from one and only one object. However, we may encounter a situation that each measurement may or may not be associated with multiple objects at each time step –spawning. Therefore, the association of each measurement to multiple objects is a crucial task to perform in order to track multiple objects with birth and death. In this paper, we introduce a novel Bayesian nonparametric approach that models a scenario where each observation may be drawn from an unknown number of objects for which it provides a tractable Markov chain Monte Carlo (MCMC) approach to sample from the posterior distribution. Number of objects at each time step, itself, is also assumed to be unknown. We, then, show through experiments the advantage of nonparametric modeling to scenarios with spawning events. Our experiment results also demonstrate the advantages of our framework over the existing methods.
更多查看译文
关键词
Dependent Dirichlet Process,Indian Buffet Process,Spawning
AI 理解论文
溯源树
样例
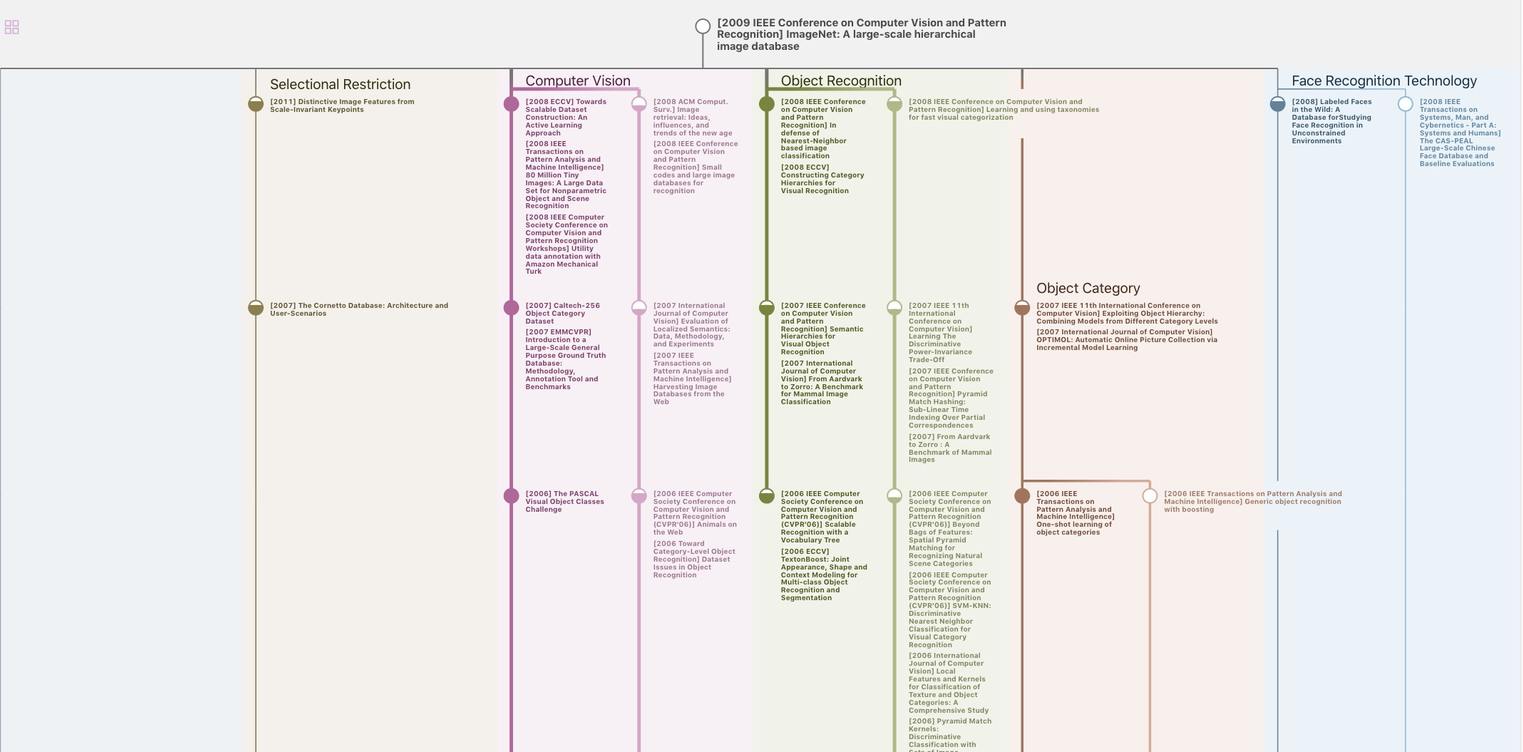
生成溯源树,研究论文发展脉络
Chat Paper
正在生成论文摘要