FANet: Feature aggregation network for RGBD saliency detection
Signal Processing: Image Communication(2022)
摘要
The crucial issue in RGBD saliency detection is how to adequately mine and fuse the geometric information and the appearance information contained in depth maps and RGB images, respectively. In this paper, we propose a novel feature aggregation network FANet including a feature extraction module and an aggregation module for RGBD saliency detection. The premier characteristic of FANet is the feature aggregation module consisting of a designed region enhanced module (REM) and a series of deployed hierarchical fusion module (HFM). Specifically, on one hand, the REM provides the powerful capability in differentiating salient objects and background. On the other hand, the HFM is used to gradually integrate high-level semantic information and low-level spatial details, where the K-nearest neighbor graph neural networks (KGNNs) and the non-local module (NLM) are embedded into HFM to dig the geometric information and enhance high-level appearance features, respectively. Extensive experiments on five RGBD datasets show that our model achieves compelling performance against the current 11 state-of-the-art RGBD saliency models.
更多查看译文
关键词
RGBD saliency,Feature aggregation,Graph neural networks,Hierarchical fusion
AI 理解论文
溯源树
样例
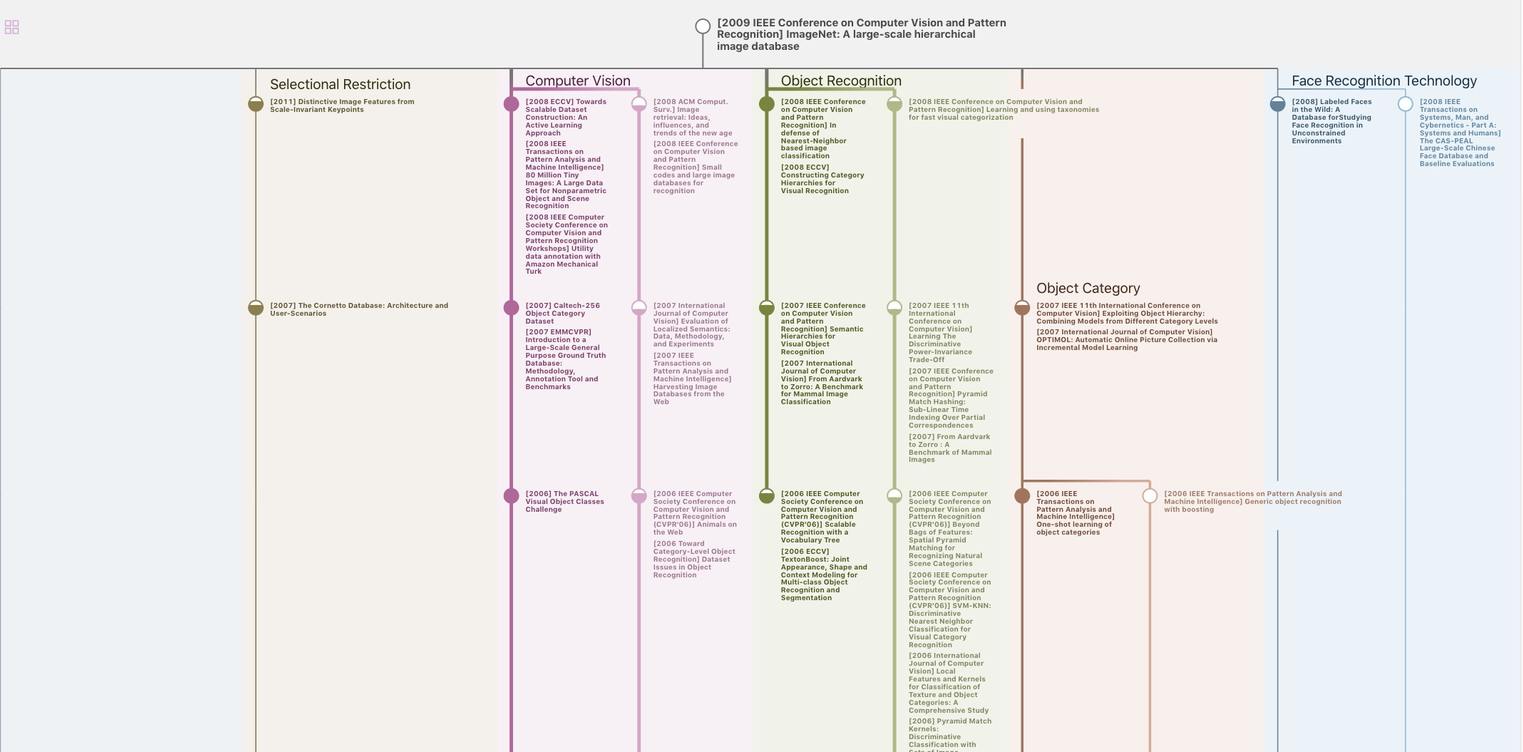
生成溯源树,研究论文发展脉络
Chat Paper
正在生成论文摘要