Video super-resolution using a hierarchical recurrent multireceptive-field integration network
DIGITAL SIGNAL PROCESSING(2022)
摘要
Video super-resolution methods always suffer from being computationally expensive and time-consuming because of sequentially performing the temporal frame alignment and spatial feature fusion. To address the issue, a novel video super-resolution method based on a hierarchical recurrent multireceptive-field integration network is proposed. Specifically, a hierarchical recurrent structure, which can fully model the temporal correlation of sequential low-resolution frames, is proposed. Then, a residual multireceptivefield integration module is introduced to obtain the hidden state and rich context features. Finally, an adaptive fusion strategy is employed to further boost video-reconstruction quality using fewer parameters. Moreover, the proposed method is capable of improving the performance and speed of video super-resolution. Extensive experiments on benchmark datasets demonstrate that the proposed method achieves a better balance between the reconstruction performance and speed than existing video super resolution methods. (C)& nbsp;2021 Elsevier Inc. All rights reserved.
更多查看译文
关键词
Video super-resolution, Deep learning, Recurrent convolutional network, Multireceptive-field feature representation
AI 理解论文
溯源树
样例
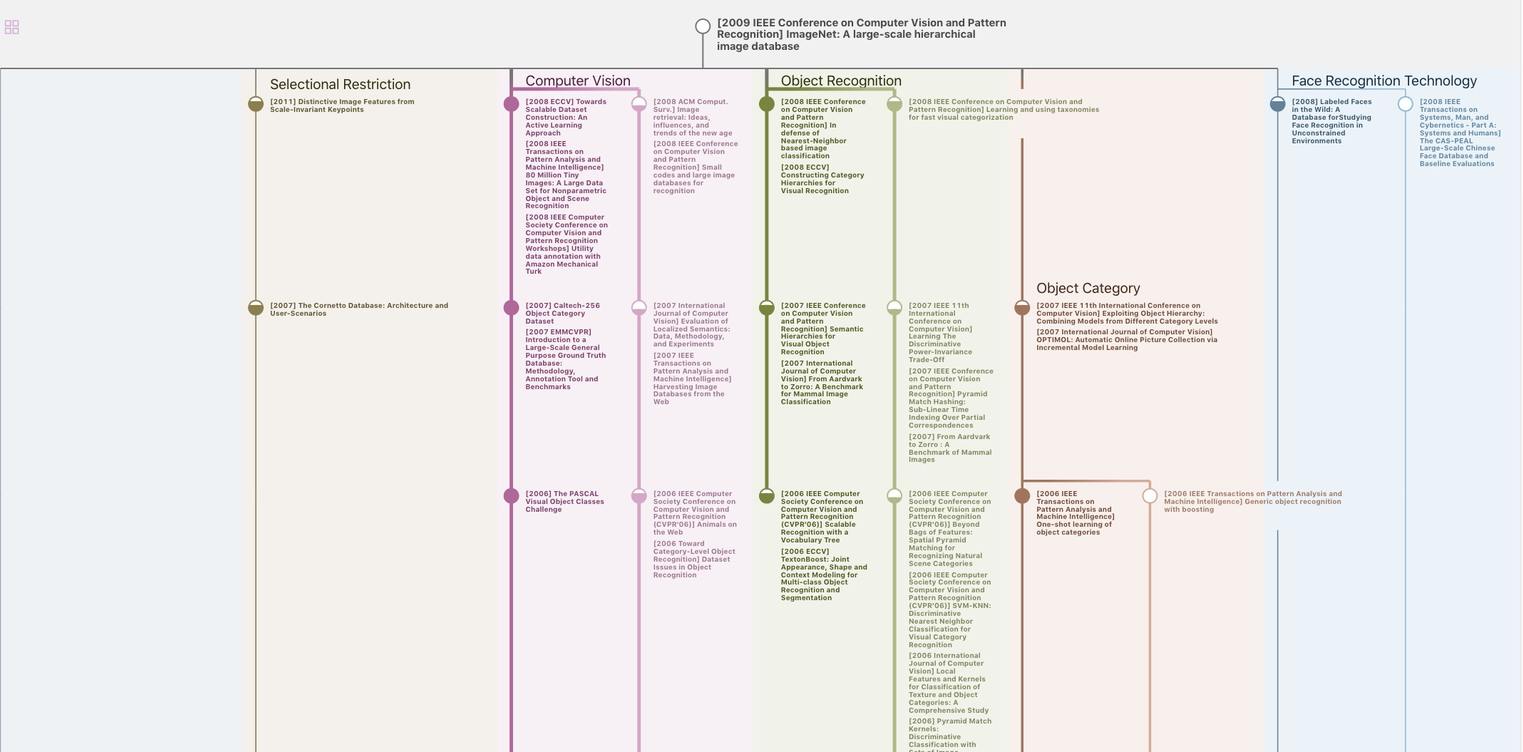
生成溯源树,研究论文发展脉络
Chat Paper
正在生成论文摘要