A gradient deconvolutional network for side-channel attacks
COMPUTERS & ELECTRICAL ENGINEERING(2022)
摘要
Recently, deep learning has been introduced as an interesting alternative to perform sidechannel attacks, which are nowadays well-known threats to secure systems in the electronic equipment. However, these end-to-end devices can limit their adoption in the complex power traces due to lack of transparency. In this work, we propose a new deconvolution architecture to evaluate the security of secure systems, which combines gradient calculation and deconvolutional operation. First, we use the U-Net-like structure to classify to verify the positive impact of the deconvolution on classification. Next, we propose a gradient deconvolution network (GDN), which combines gradient calculation and model training firstly. The result of gradient calculation locates the leakage and transfers the information to the model to get a better training effect. Finally, we evaluate our methodology with the public datasets and provide visualization of Points of Interest (POIs).
更多查看译文
关键词
Side-channel attacks, Electronic safety, Leakage characterization, Deep learning
AI 理解论文
溯源树
样例
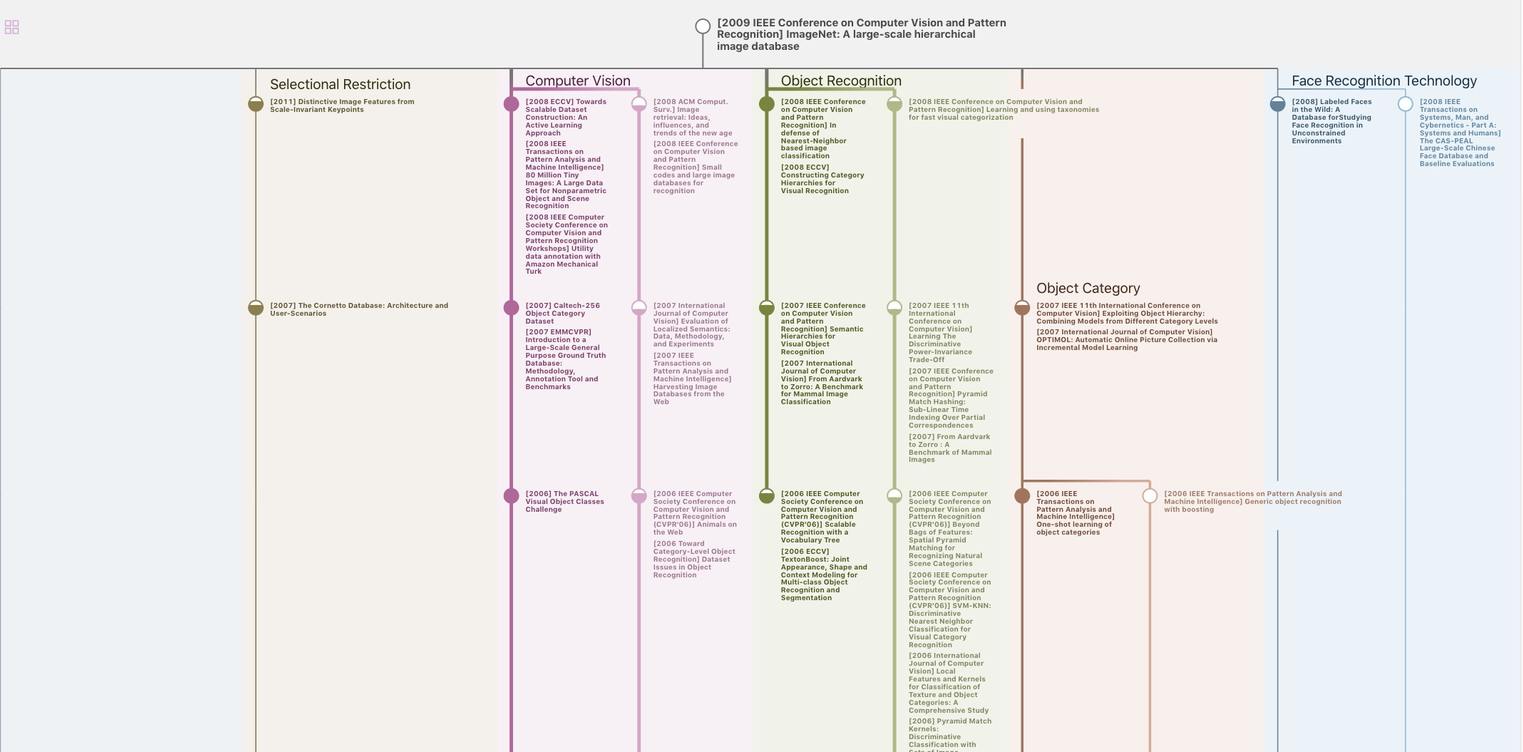
生成溯源树,研究论文发展脉络
Chat Paper
正在生成论文摘要