Graph Based Feature Selection for Reduction of Dimensionality in Next-Generation RNA Sequencing Datasets
ALGORITHMS(2022)
摘要
Analysis of high-dimensional data, with more features (p) than observations (N) (p > N), places significant demand in cost and memory computational usage attributes. Feature selection can be used to reduce the dimensionality of the data. We used a graph-based approach, principal component analysis (PCA) and recursive feature elimination to select features for classification from RNAseq datasets from two lung cancer datasets. The selected features were discretized for association rule mining where support and lift were used to generate informative rules. Our results show that the graph-based feature selection improved the performance of sequential minimal optimization (SMO) and multilayer perceptron classifiers (MLP) in both datasets. In association rule mining, features selected using the graph-based approach outperformed the other two feature-selection techniques at a support of 0.5 and lift of 2. The non-redundant rules reflect the inherent relationships between features. Biological features are usually related to functions in living systems, a relationship that cannot be deduced by feature selection and classification alone. Therefore, the graph-based feature-selection approach combined with rule mining is a suitable way of selecting and finding associations between features in high-dimensional RNAseq data.
更多查看译文
关键词
big data, feature selection, classification, discretization, association rule mining
AI 理解论文
溯源树
样例
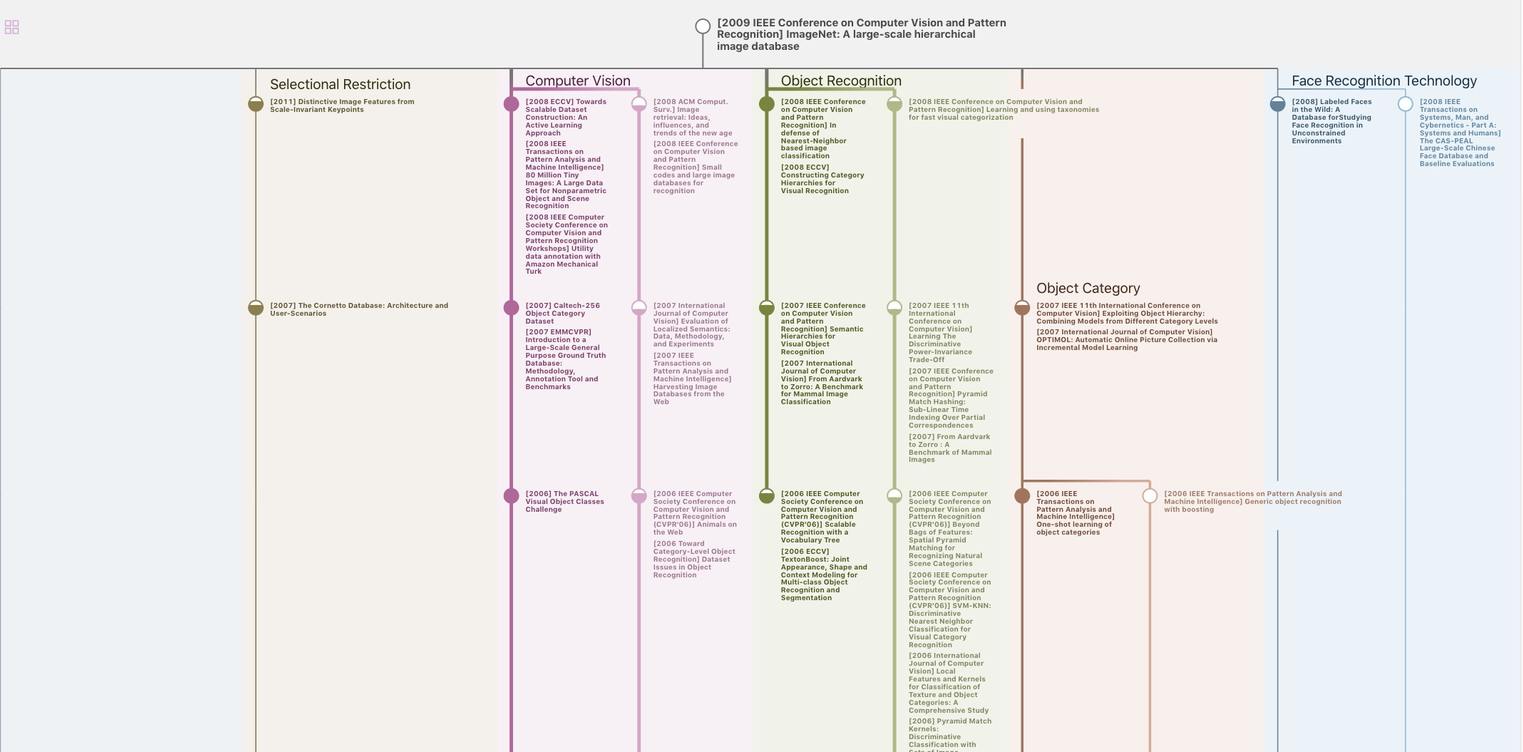
生成溯源树,研究论文发展脉络
Chat Paper
正在生成论文摘要