A deep learning framework for glaucoma detection based on robust optic disc segmentation and transfer learning
INTERNATIONAL JOURNAL OF IMAGING SYSTEMS AND TECHNOLOGY(2022)
摘要
Glaucoma is a common ocular disorder, inflicting blindness in millions of people, early detection of which can reduce adverse outcomes. This paper presents a two-stage deep learning framework UNet-SNet, for glaucoma detection. Initially, each fundus image is segmented into GMM super pixels and the Region of Interest (RoI) is separated by Cuckoo Search Optimization (CSO). In the first stage, a regularized UNet is trained with RoIs for OD segmentation. In the second stage, a SqueezeNet is fine-tuned with deep features of the ODs to discriminate fundus images into glaucomotousor Normal. The UNet is trained and tested with the RIGA and RIM-ONEv2 datasets, achieving 97.84% and 99.85% accuracies respectively. The classifier is trained with the ODs segmented from the RIM-ONEv2 dataset and tested with ACRIMA, Drishti-GS1 and RIM-ONEv1 datasets accomplishing 99.86%, 97.05% and 100% accuracies respectively. Performance evaluations and complexity analyses with state-of-the-art systems demonstrate the superiority of the proposed model.
更多查看译文
关键词
glaucoma, GMM super pixel, SqueezeNet, transfer learning, UNet
AI 理解论文
溯源树
样例
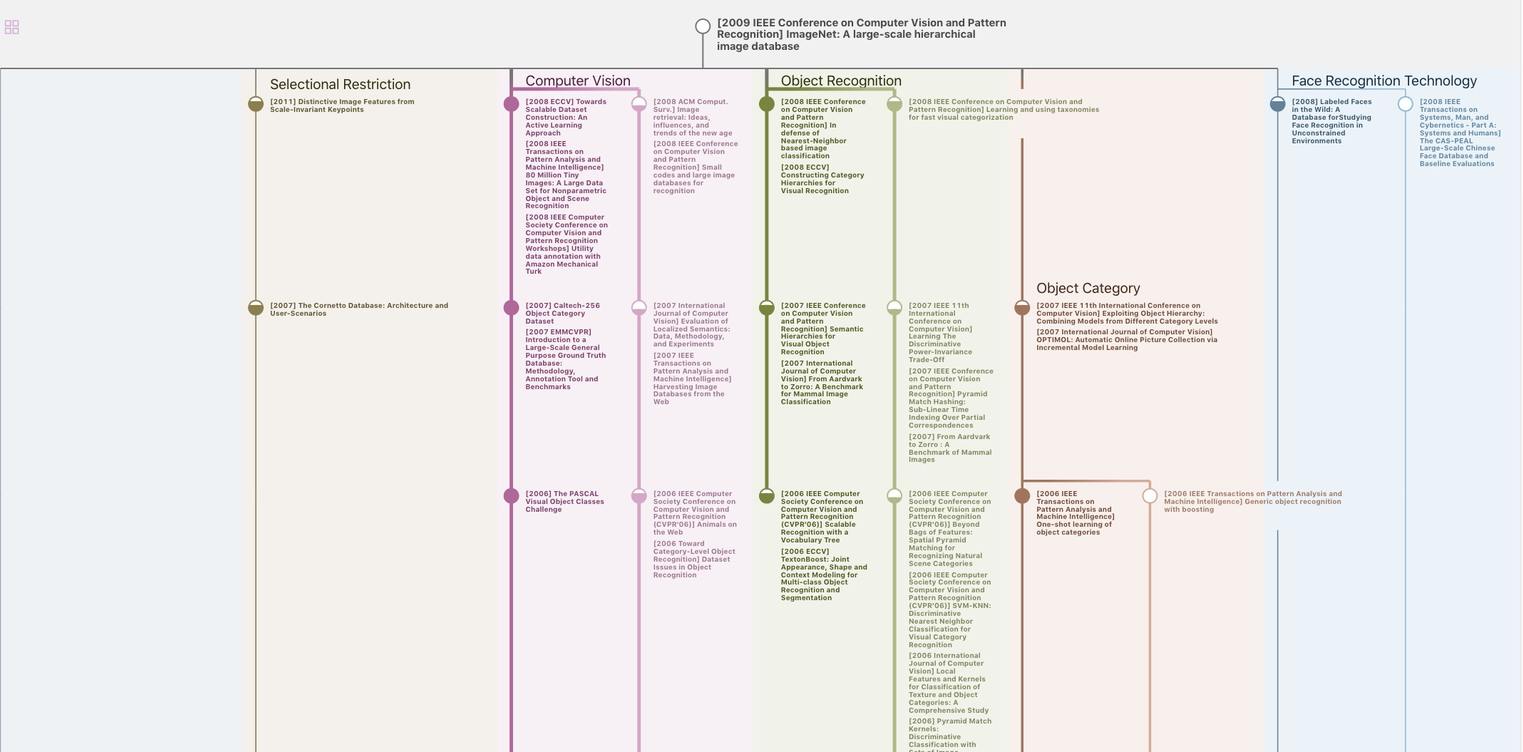
生成溯源树,研究论文发展脉络
Chat Paper
正在生成论文摘要