A Deep Q-Learning Bisection Approach for Power Allocation in Downlink NOMA Systems
IEEE Communications Letters(2022)
摘要
In this work, we study the weighted sum-rate maximization problem for a downlink non-orthogonal multiple access (NOMA) system. With power and data-rate constraints, this problem is generally non-convex. Therefore, a novel solution based on the deep reinforcement learning (DRL) framework is proposed for the power allocation problem. While previous work based on DRL restrict the solution to a limited set of possible power levels, the proposed DRL framework is specifically designed to find a solution with a much larger granularity, emulating a continuous power allocation. Simulation results show that the proposed power allocation method outperforms two baseline algorithms. Moreover, it achieves almost 85% of the weighted sum-rate obtained by a far more complex genetic algorithm that approaches exhaustive search in terms of performance.
更多查看译文
关键词
Non-orthogonal multiple access,deep reinforcement learning,weighted sum-rate maximization,successive interference cancellation stability
AI 理解论文
溯源树
样例
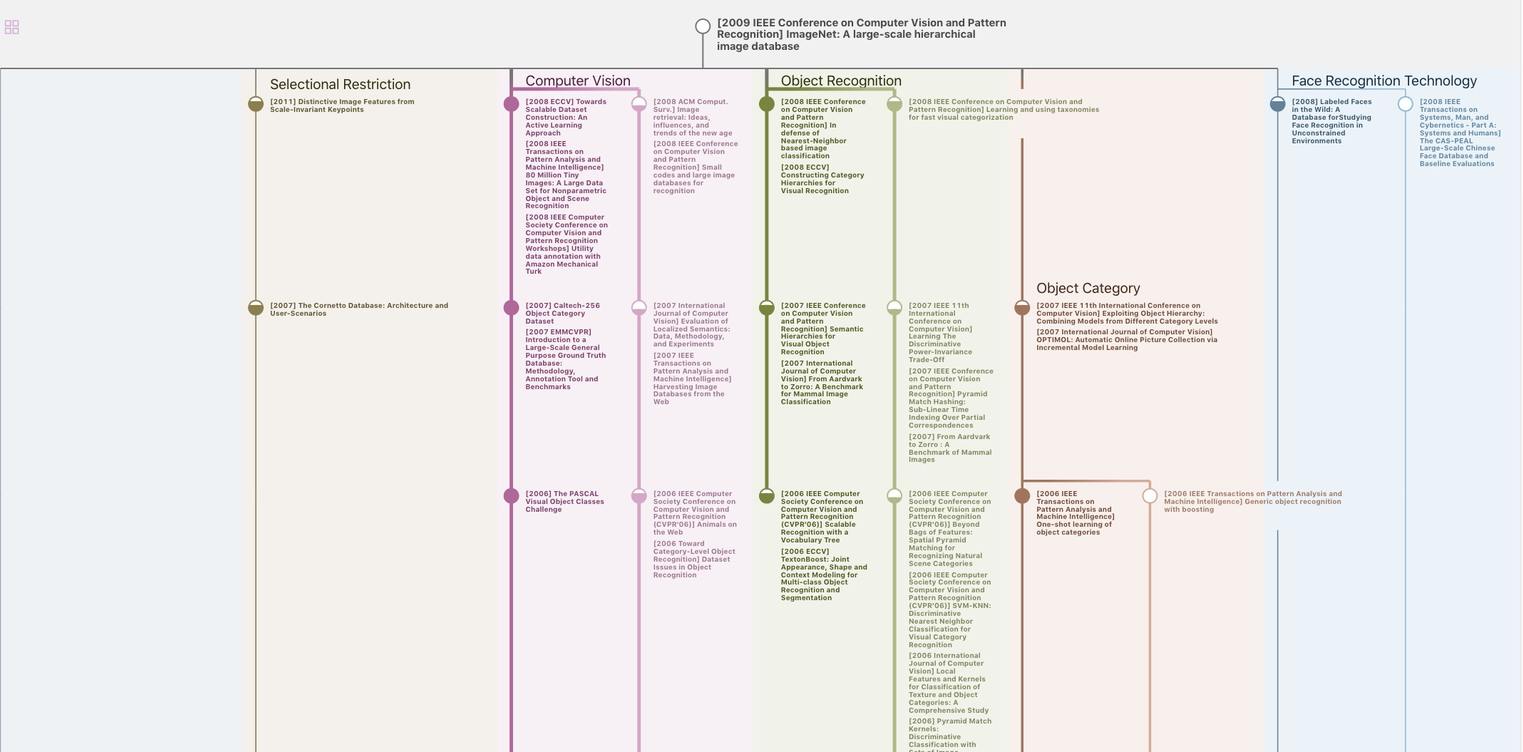
生成溯源树,研究论文发展脉络
Chat Paper
正在生成论文摘要