MoDANet: Multi-Task Deep Network for Joint Automatic Modulation Classification and Direction of Arrival Estimation
IEEE Communications Letters(2022)
摘要
In this letter, a multi-task deep convolutional neural network, namely MoDANet, is proposed to perform modulation classification and DOA estimation simultaneously. In particular, the network architecture is designed with multiple residual modules, which tackle the vanishing gradient problem. The multi-task learning (MTL) efficiency of MoDANet was evaluated with different variants of Y-shaped connection and fine-tuning some hyper-parameters of the deep network. As a result, MoDANet with one shared residual module using more filters, larger filter size, and longer signal length can achieve better performance of modulation classification and DOA estimation, but those might result in higher computational complexity. Therefore, choosing these parameters to attain a good trade-off between accuracy and computational cost is important, especially for resource-constrained devices. The network is investigated with two typical propagation channel models, including Pedestrian A and Vehicular A, to show the effect of those channels on the efficiency of the network. Remarkably, our work is the first DL-based MTL model to handle two unrelated tasks of modulation classification and DOA estimation.
更多查看译文
关键词
Automatic modulation classification,direction of arrival estimation,deep neural network,MIMO system
AI 理解论文
溯源树
样例
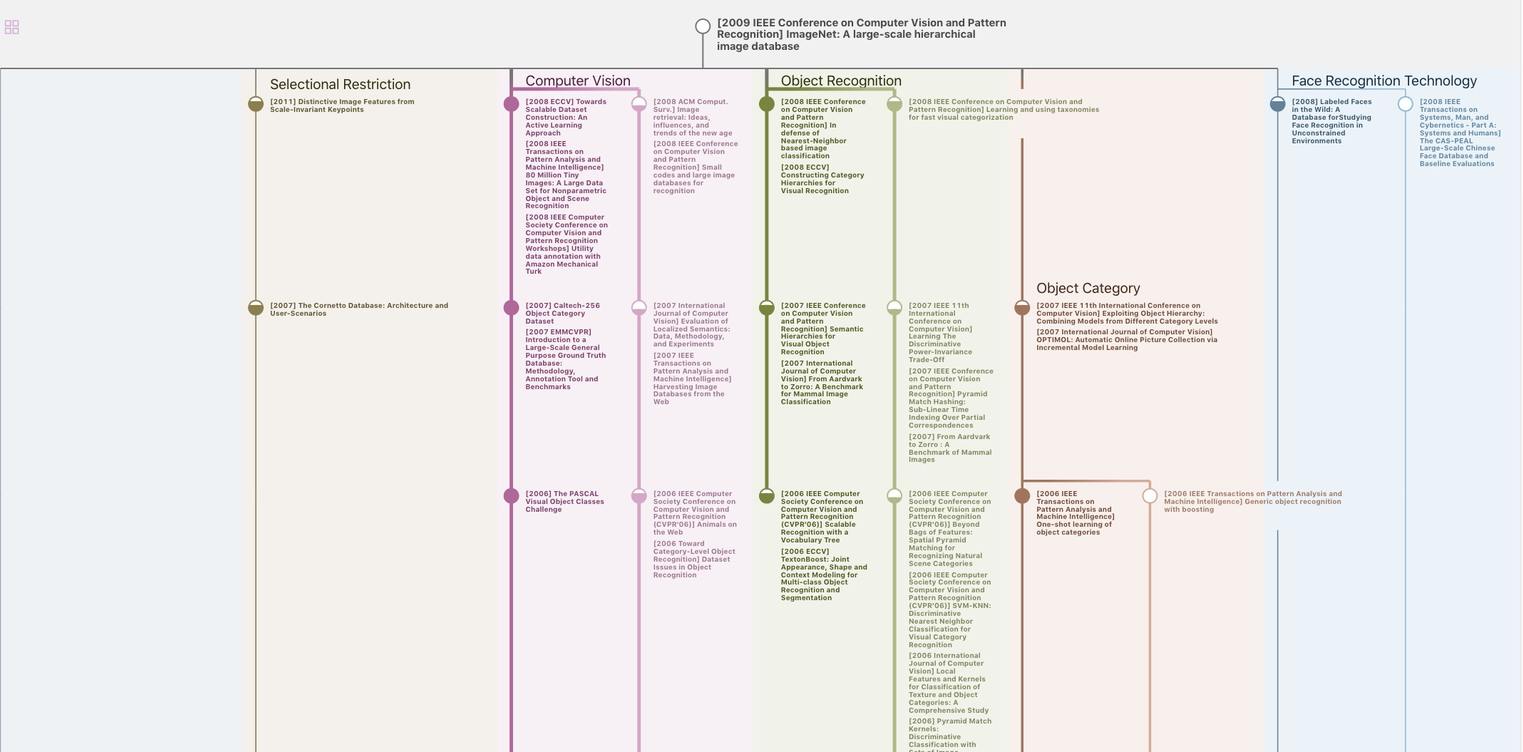
生成溯源树,研究论文发展脉络
Chat Paper
正在生成论文摘要