Impact of Gaussian Noise for Optimized Support Vector Machine Algorithm Applied to Medicare Payment on Raspberry Pi
INFORMATICA-AN INTERNATIONAL JOURNAL OF COMPUTING AND INFORMATICS(2021)
摘要
A relatively large dataset coupled with efficient but computationally slow machine learning algorithm poses a great deal of challenge for Internet of Things (IoT). On the contrary, Deep Learning Neural Networks (DLANNs) are known for good performances in terms of accuracy, but by nature are computationally intensive. Based on this argument, the purpose of this article is to apply a pipelined Support Vector Machine (SVM)) learning algorithm for benchmarking public health data using Internet of Things (IoT). Support Vector Machine (SVM) a very good performing machine learning algorithm but has constraints in terms of huge training time and its performance is also susceptible to noise. The applied software pipelined architecture to SVM was to minimize its computational time under a resource constrained device like raspberry pi. It was tested with a medicare dataset with Gaussian noise to assess the impact of noise. The classification results of Total Medicare Standardized Payment Amount obtained indicated that the proposed pipelined SVM model was optimal in performance compared to DLANN model by 79.74% in terms of computational time. Also the performance of SVM in terms of area under curve (AUC) was better compared to other models and outscored Logistic Regression by 7.2%, and DLANN model by 22.65%.
更多查看译文
关键词
medicare analysis, internet of things, data, statistical feature optimization techniques, support vector machine, pipelined models
AI 理解论文
溯源树
样例
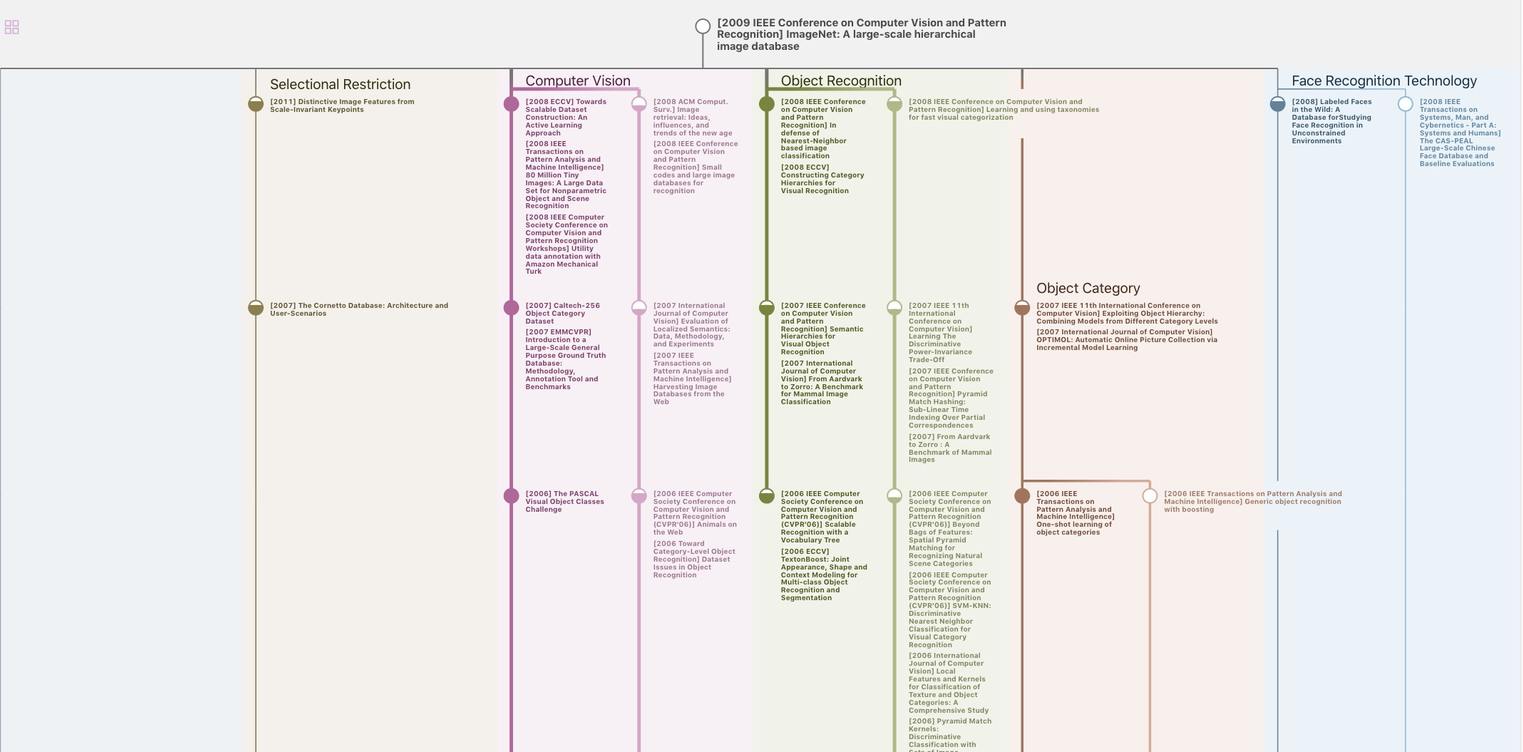
生成溯源树,研究论文发展脉络
Chat Paper
正在生成论文摘要