Method to enhance deep learning fault diagnosis by generating adversarial samples
APPLIED SOFT COMPUTING(2022)
摘要
Modern industrial fields utilize complex mechanical equipment and machinery, which are closely linked, and equipment faults are difficult to express. Therefore, fault diagnosis is important to ensure the safety of complex mechanical equipment in modern industries. Deep learning has achieved excellent results with recent fault diagnosis methods. At present, three common deep learning models (MLP, CNN, and RNN models) can achieve diagnosis rates close to 100% with original fault diagnosis data and a signal-to-noise ratio above 10 dB. However, we found that the diagnostic rate of these three models was completely incorrect when an adversarial sample with a signal-to-noise ratio noise greater than 10 dB was added to the original sample. We propose a GAN-based adversarial signal generative adversarial network (AdvSGAN) in this paper. We conduct experiments on the CWRU dataset and conclude that we can easily obtain adversarial noise and generate training samples through AdvSGAN. With the addition of adversarial data training, the diagnostic rate of the model on these adversarial samples increased from less than 5% to 98.69%, 97.38% and 96.94%. Hence, this method increases the reliability of our deep learning model. (C) 2021 Elsevier B.V. All rights reserved.
更多查看译文
关键词
Fault diagnosis, Adversarial-signal generative adversarial, network, Multilayer perceptron, Recurrent neural network, Convolutional neural network
AI 理解论文
溯源树
样例
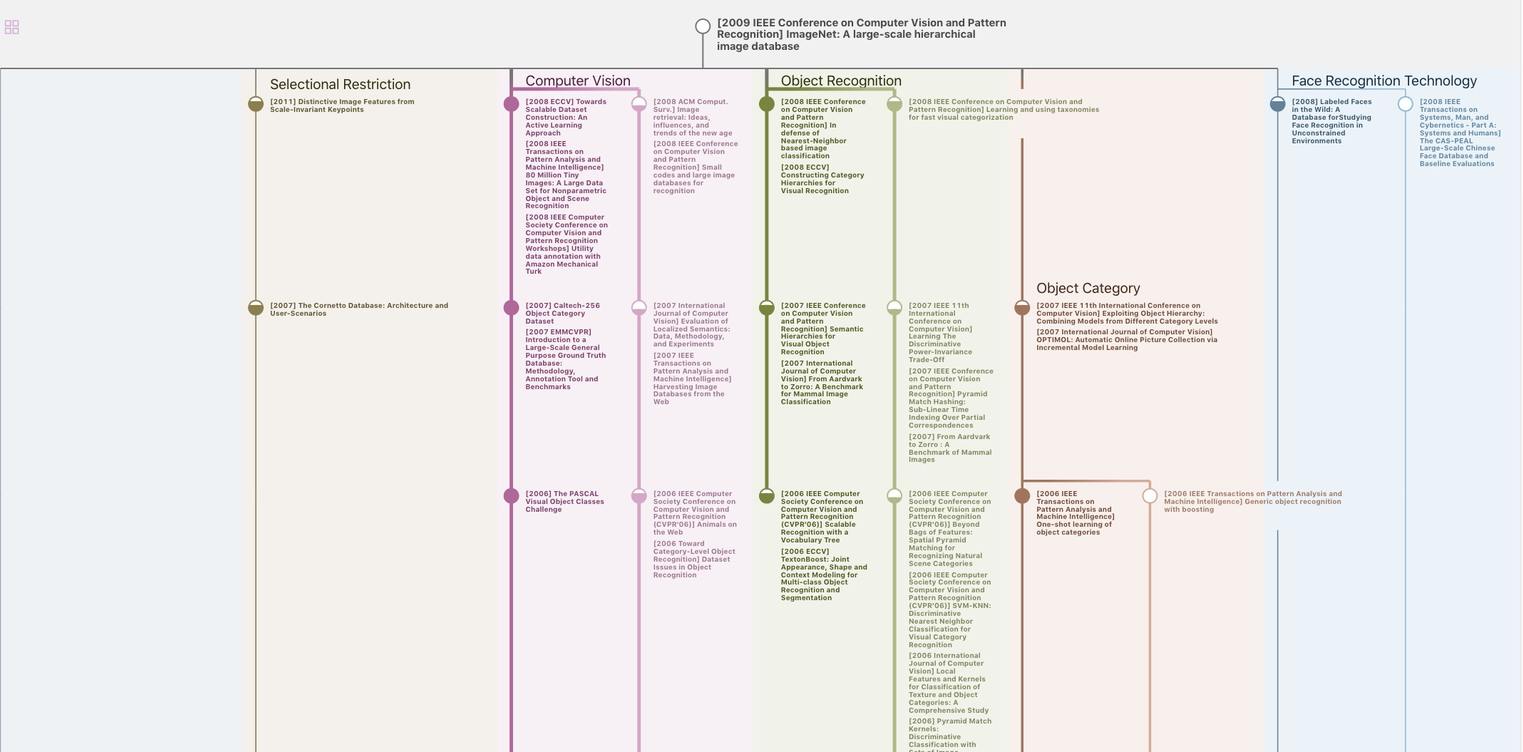
生成溯源树,研究论文发展脉络
Chat Paper
正在生成论文摘要