4D U-Nets for Multi-Temporal Remote Sensing Data Classification
REMOTE SENSING(2022)
摘要
Multispectral sensors constitute a core earth observation imaging technology generating massive high-dimensional observations acquired across multiple time instances. The collected multi-temporal remote sensed data contain rich information for Earth monitoring applications, from flood detection to crop classification. To easily classify such naturally multidimensional data, conventional low-order deep learning models unavoidably toss away valuable information residing across the available dimensions. In this work, we extend state-of-the-art convolutional network models based on the U-Net architecture to their high-dimensional analogs, which can naturally capture multi-dimensional dependencies and correlations. We introduce several model architectures, both of low as well as of high order, and we quantify the achieved classification performance vis-a-vis the latest state-of-the-art methods. The experimental analysis on observations from Landsat-8 reveals that approaches based on low-order U-Net models exhibit poor classification performance and are outperformed by our proposed high-dimensional U-Net scheme.
更多查看译文
关键词
remote sensing, u-nets, higher-order convolutional neural networks, multi-temporal data classification
AI 理解论文
溯源树
样例
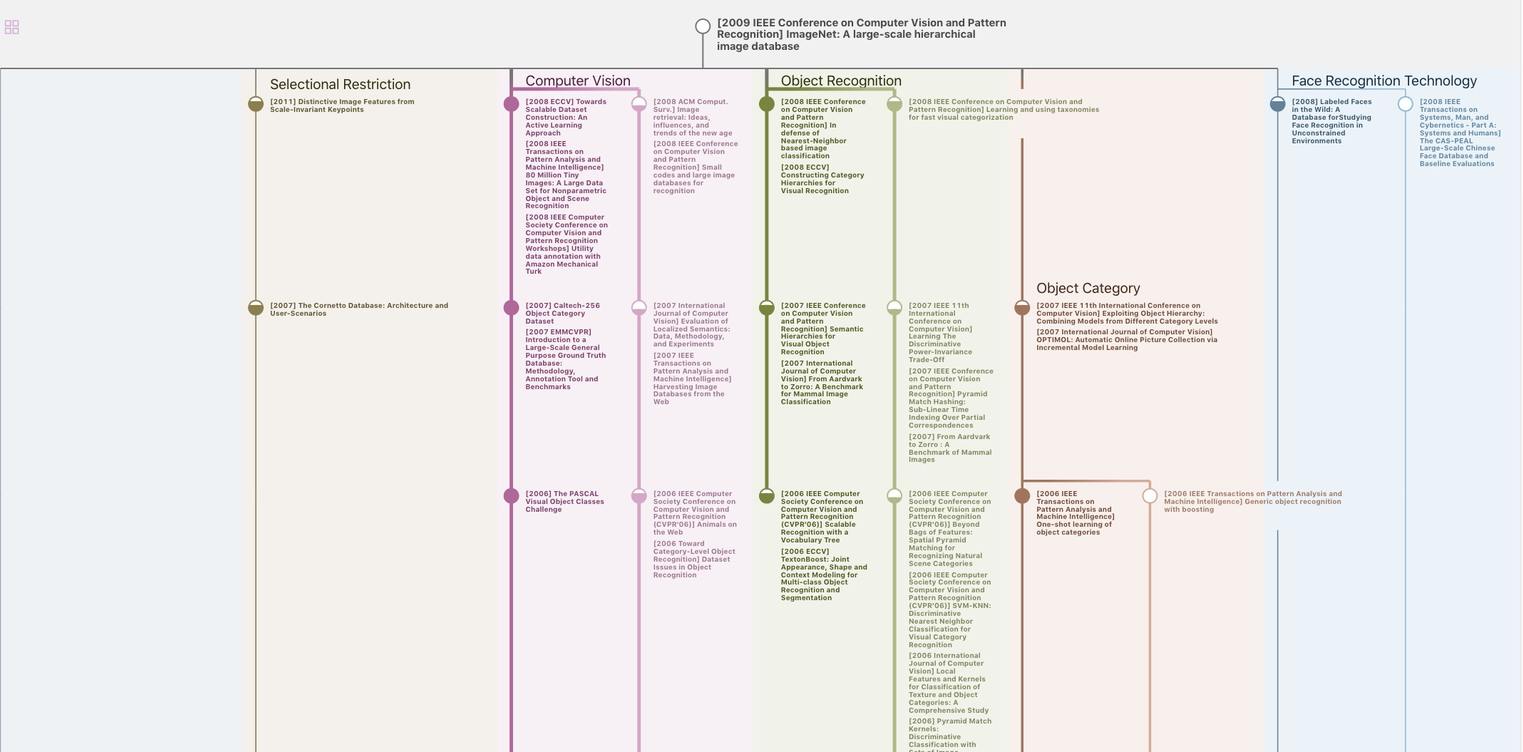
生成溯源树,研究论文发展脉络
Chat Paper
正在生成论文摘要