Learning from All Vehicles
IEEE Conference on Computer Vision and Pattern Recognition(2022)
摘要
In this paper, we present a system to train driving policies from experiences collected not just from the ego-vehicle, but all vehicles that it observes. This system uses the behaviors of other agents to create more diverse driving scenarios without collecting additional data. The main difficulty in learning from other vehicles is that there is no sensor information. We use a set of supervisory tasks to learn an intermediate representation that is invariant to the viewpoint of the controlling vehicle. This not only provides a richer signal at training time but also allows more complex reasoning during inference. Learning how all vehicles drive helps predict their behavior at test time and can avoid collisions. We evaluate this system in closed-loop driving simulations. Our system outperforms all prior methods on the public CARLA Leaderboard by a wide margin, improving driving score by 25 and route completion rate by 24 points.
更多查看译文
关键词
Navigation and autonomous driving
AI 理解论文
溯源树
样例
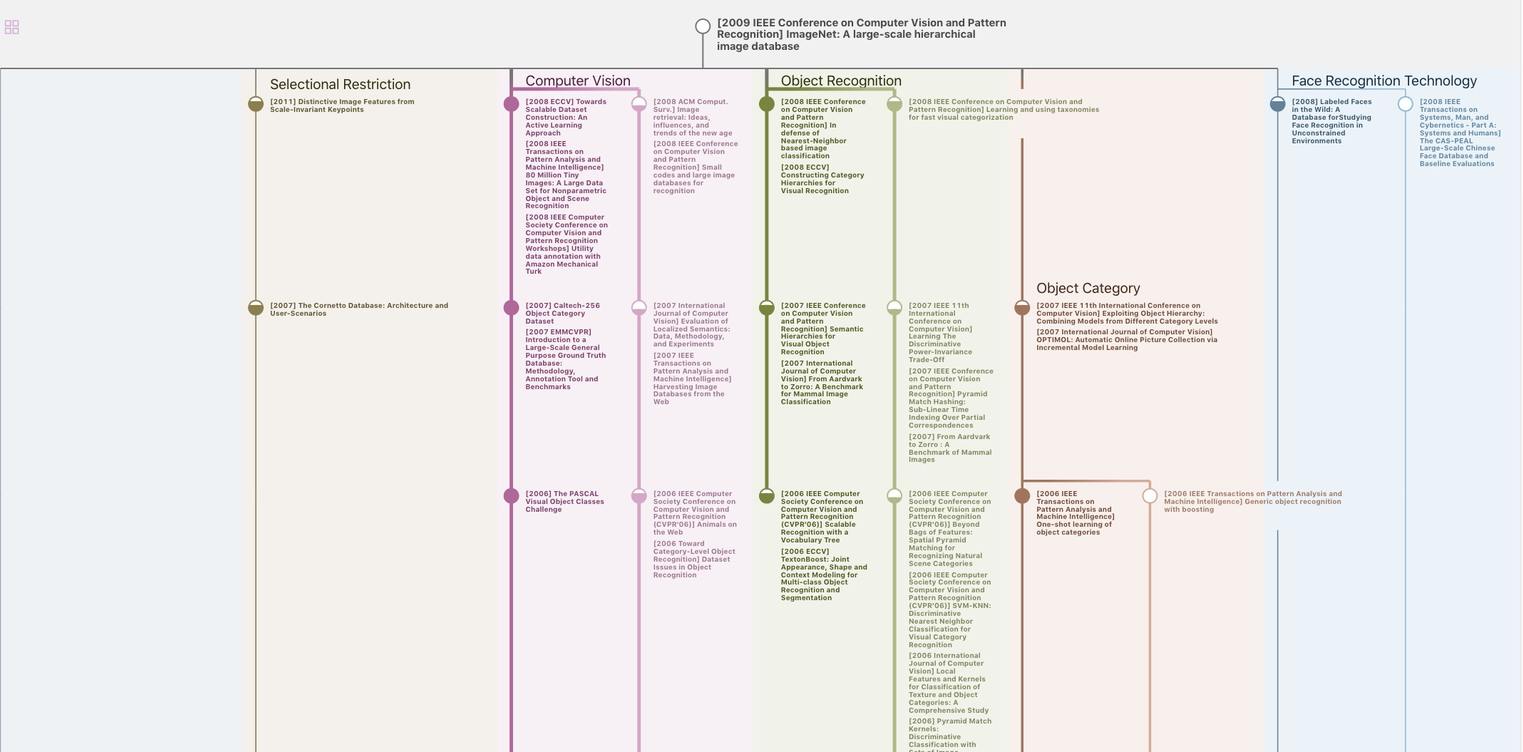
生成溯源树,研究论文发展脉络
Chat Paper
正在生成论文摘要