Bayesian nonparametric adjustment of confounding.
Biometrics(2023)
摘要
Analysis of observational studies increasingly confronts the challenge of determining which of a possibly high-dimensional set of available covariates are required to satisfy the assumption of ignorable treatment assignment for estimation of causal effects. We propose a Bayesian nonparametric approach that simultaneously (1) prioritizes inclusion of adjustment variables in accordance with existing principles of confounder selection; (2) estimates causal effects in a manner that permits complex relationships among confounders, exposures, and outcomes; and (3) provides causal estimates that account for uncertainty in the nature of confounding. The proposal relies on specification of multiple Bayesian additive regression trees models, linked together with a common prior distribution that accrues posterior selection probability to covariates on the basis of association with both the exposure and the outcome of interest. A set of extensive simulation studies demonstrates that the proposed method performs well relative to similarly-motivated methodologies in a variety of scenarios. We deploy the method to investigate the causal effect of emissions from coal-fired power plants on ambient air pollution concentrations, where the prospect of confounding due to local and regional meteorological factors introduces uncertainty around the confounding role of a high-dimensional set of measured variables. Ultimately, we show that the proposed method produces more efficient and more consistent results across adjacent years than alternative methods, lending strength to the evidence of the causal relationship between SO emissions and ambient particulate pollution.
更多查看译文
关键词
BART,Bayesian nonparametrics,air pollution,causal inference,confounder selection
AI 理解论文
溯源树
样例
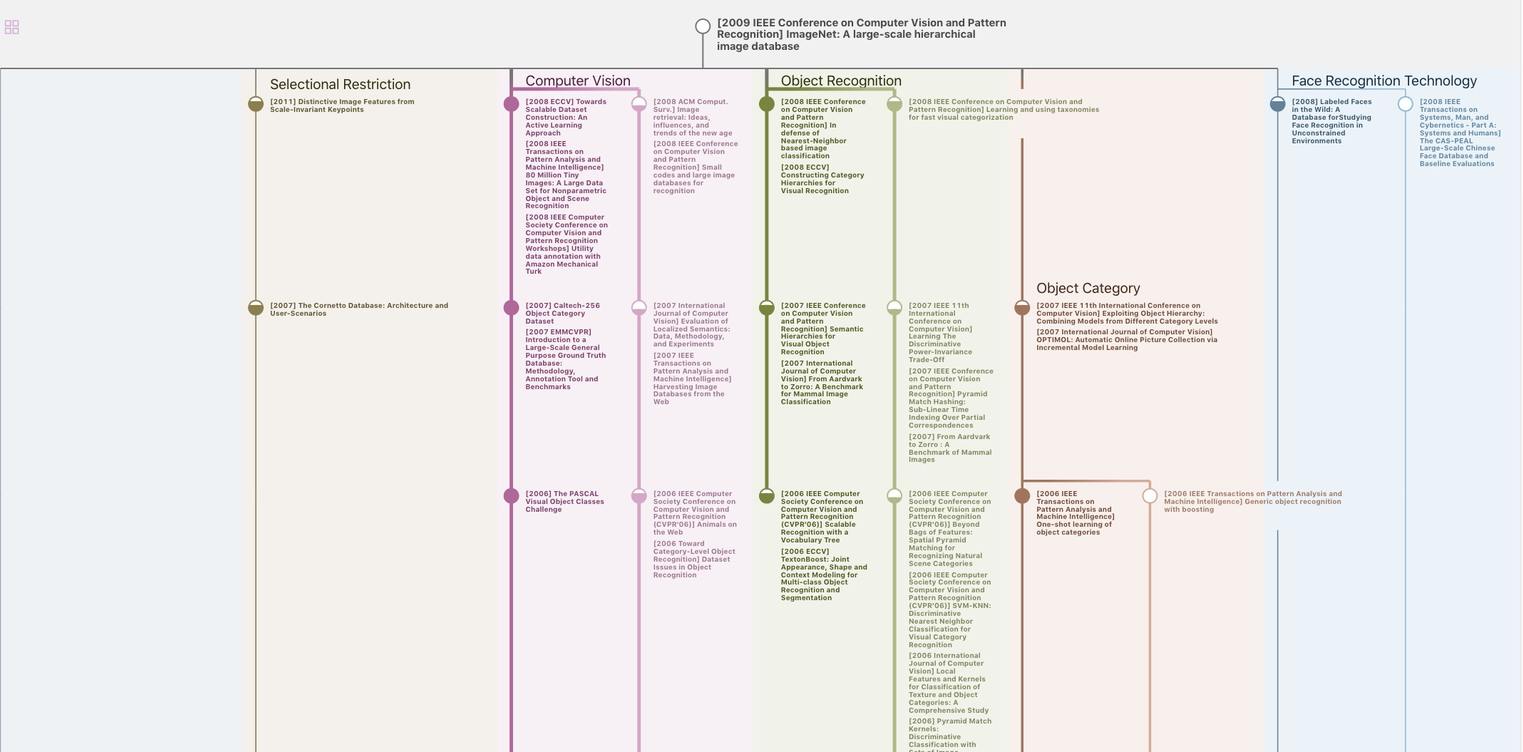
生成溯源树,研究论文发展脉络
Chat Paper
正在生成论文摘要