Making recommender systems forget: Learning and unlearning for erasable recommendation
KNOWLEDGE-BASED SYSTEMS(2024)
摘要
Regulations now mandate data-driven systems, e.g., recommender systems, to empower users to delete private individual data. This prompts the crucial unlearning of data from machine learning models, spotlighting the understudied machine unlearning problem. Despite the widespread usage of machine learning models in modern-day recommender systems, unlearning in this context lacks attention. Existing unlearning methods fall short in the preservation of collaborative information across users and items. To bridge this gap, we propose LASER: a model-agnostic erasable recommendation framework. LASER partitions data into disjoint and balanced shards using hypergraph-based embeddings. Sequential training on these shards, augmented by curriculum learning, LASER sufficiently preserves collaborative information and refines model utility. To address the inefficiency of sequential training, we integrate early stopping and parameter manipulation. Our theoretical analyses and real-world dataset experiments validate LASER's effectiveness. It enables efficient unlearning while outperforming state-of-the-art models in preserving model utility.
更多查看译文
关键词
Recommender systems,Collaborative filtering,Machine unlearning
AI 理解论文
溯源树
样例
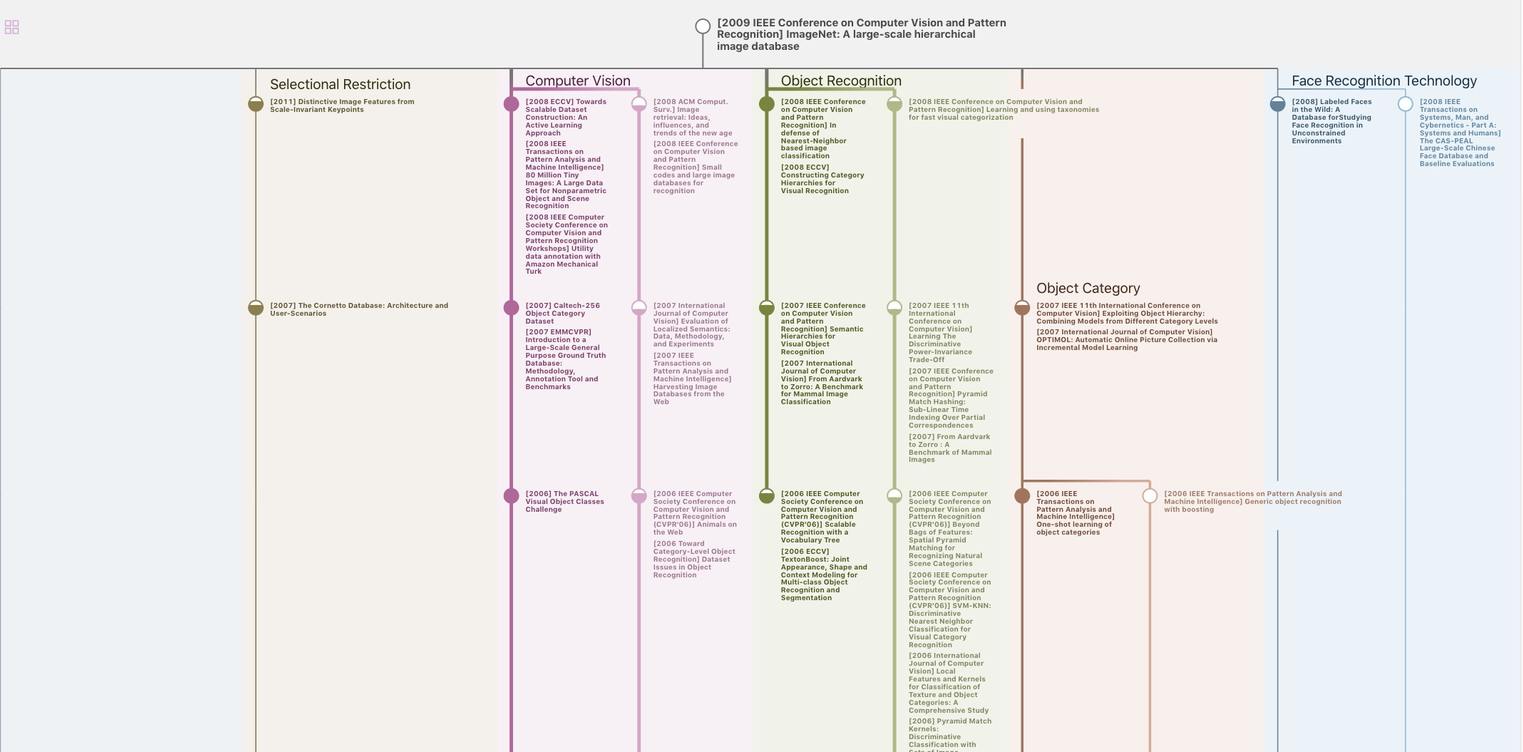
生成溯源树,研究论文发展脉络
Chat Paper
正在生成论文摘要