Crack free metal printing using physics informed machine learning
Acta Materialia(2022)
摘要
Process parameters and thermophysical and mechanical properties of alloys affect cracking which remains a major challenge in metal printing. Cracks occur because of multiple mechanisms and currently, there is no unified mitigation strategy. Here we evaluate the effects of variables related to the physics of cracking computed by a mechanistic model and independent experimental data using machine learning to prevent cracking. The computed solidification stress, the ratio of the vulnerable and relaxation times, ratio of the temperature gradient to solidification growth rate, and cooling rates and experimental data are used to generate a cracking susceptibility index that predicts crack formation before printing. Computed values of these four variables when used in a decision tree, support vector machines, and logistic regression can predict crack formation with exceptional accuracy. Information gain, information gain ratio, and Gini index-based feature selection calculations provide the same comparative influence of these four variables. Results are presented as easy-to-use cracking susceptibility maps. Our approach can help in process optimization, designing new alloys, and solving problems in manufacturing beyond metal printing.
更多查看译文
关键词
Metal printing,Aluminum alloys,Powder bed fusion,Hot tearing,Heat transfer and fluid flow
AI 理解论文
溯源树
样例
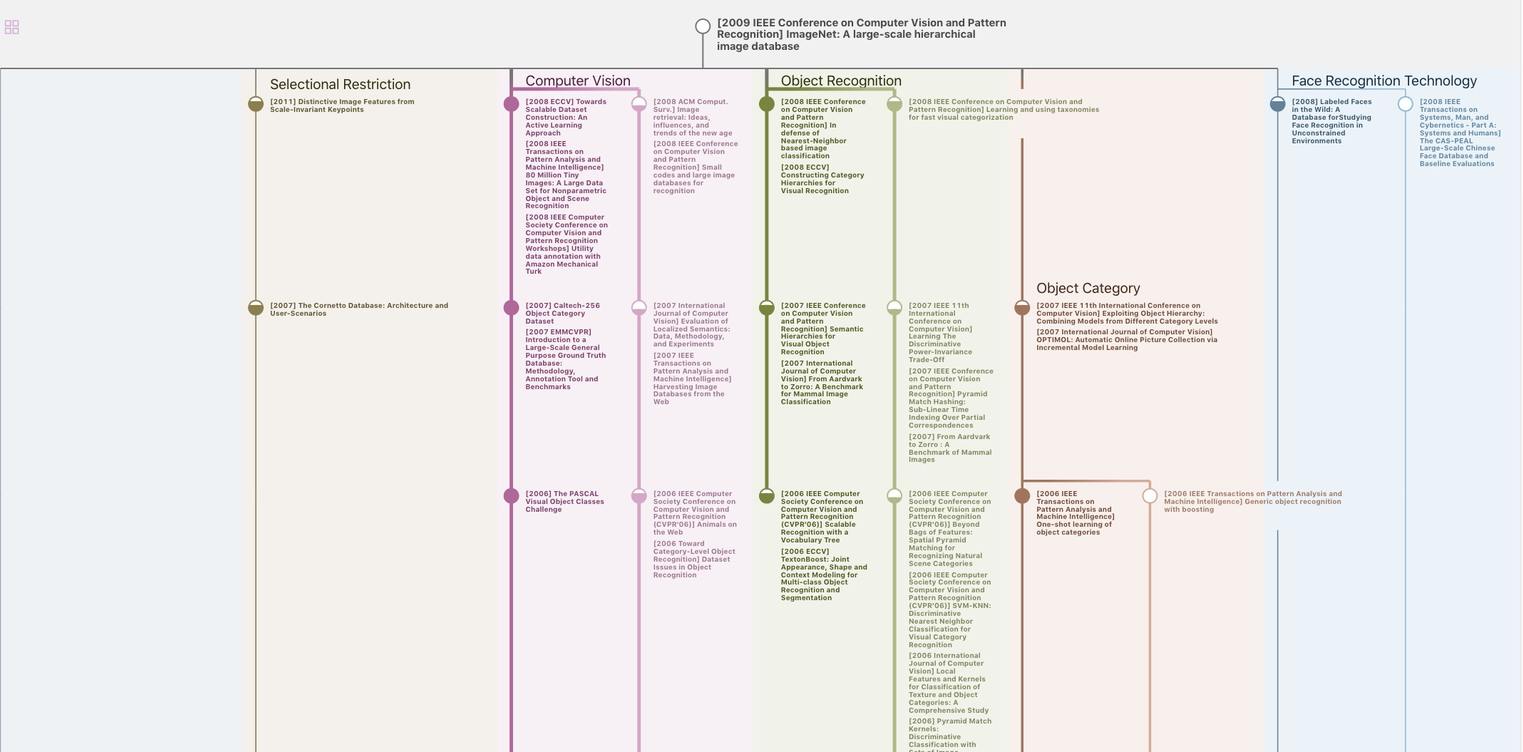
生成溯源树,研究论文发展脉络
Chat Paper
正在生成论文摘要