Improved Sampling-to-Counting Reductions in High-Dimensional Expanders and Faster Parallel Determinantal Sampling
arxiv(2022)
摘要
We study parallel sampling algorithms for classes of distributions defined via determinants: symmetric, nonsymmetric, and partition-constrained determinantal point processes. For these distributions, counting, a.k.a. computing the partition function, can be reduced to a simple determinant computation which is highly parallelizable; Csanky proved it is in NC. However, parallel counting does not automatically translate to parallel sampling, as the classic reductions between sampling and counting are inherently sequential. Despite this, we show that for all the aforementioned determinant-based distributions, a roughly quadratic parallel speedup over sequential sampling can be achieved. If the distribution is supported on subsets of size $k$ of a ground set, we show how to approximately produce a sample in $\widetilde{O}(k^{\frac{1}{2} + c})$ time with polynomially many processors for any $c>0$. In the special case of symmetric determinantal point processes, our bound improves to $\widetilde{O}(\sqrt k)$ and we show how to sample exactly in this case. We obtain our results via a generic sampling-to-counting reduction that uses approximate rejection sampling. As our main technical contribution, we show that whenever a distribution satisfies a certain form of high-dimensional expansion called entropic independence, approximate rejection sampling can achieve a roughly quadratic speedup in sampling via counting. Various forms of high-dimensional expansion, including the notion of entropic independence we use in this work, have been the source of major breakthroughs in sampling algorithms in recent years; thus we expect our framework to prove useful in the future for distributions beyond those defined by determinants.
更多查看译文
AI 理解论文
溯源树
样例
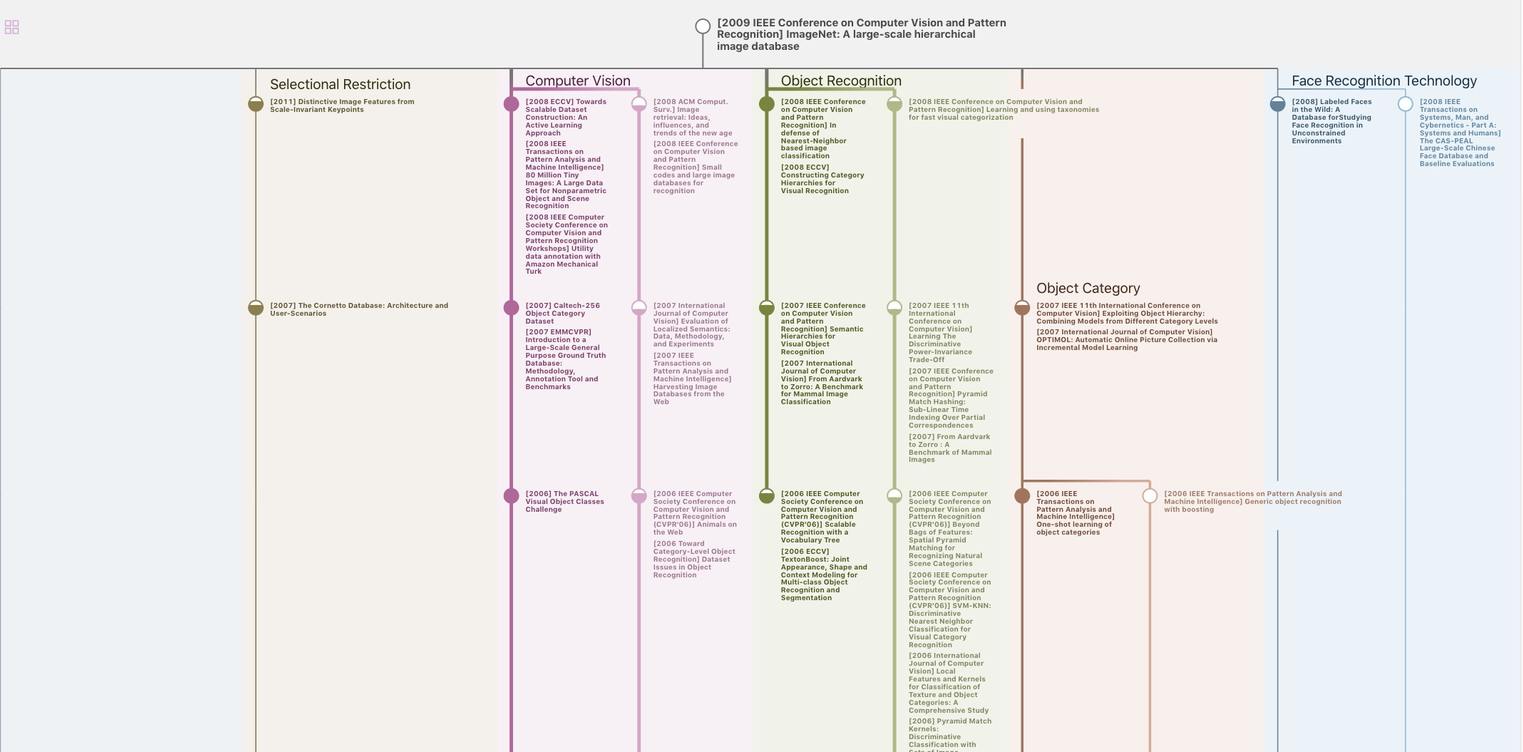
生成溯源树,研究论文发展脉络
Chat Paper
正在生成论文摘要