Domain Generalization by Mutual-Information Regularization with Pre-trained Models.
European Conference on Computer Vision(2022)
摘要
Domain generalization (DG) aims to learn a generalized model to an unseen target domain using only limited source domains. Previous attempts to DG fail to learn domain-invariant representations only from the source domains due to the significant domain shifts between training and test domains. Instead, we re-formulate the DG objective using mutual information with the oracle model, a model generalized to any possible domain. We derive a tractable variational lower bound via approximating the oracle model by a pre-trained model, called Mutual Information Regularization with Oracle (MIRO). Our extensive experiments show that MIRO significantly improves the out-of-distribution performance. Furthermore, our scaling experiments show that the larger the scale of the pre-trained model, the greater the performance improvement of MIRO. Code is available at https://github.com/kakaobrain/miro.
更多查看译文
关键词
generalization,mutual-information,pre-trained
AI 理解论文
溯源树
样例
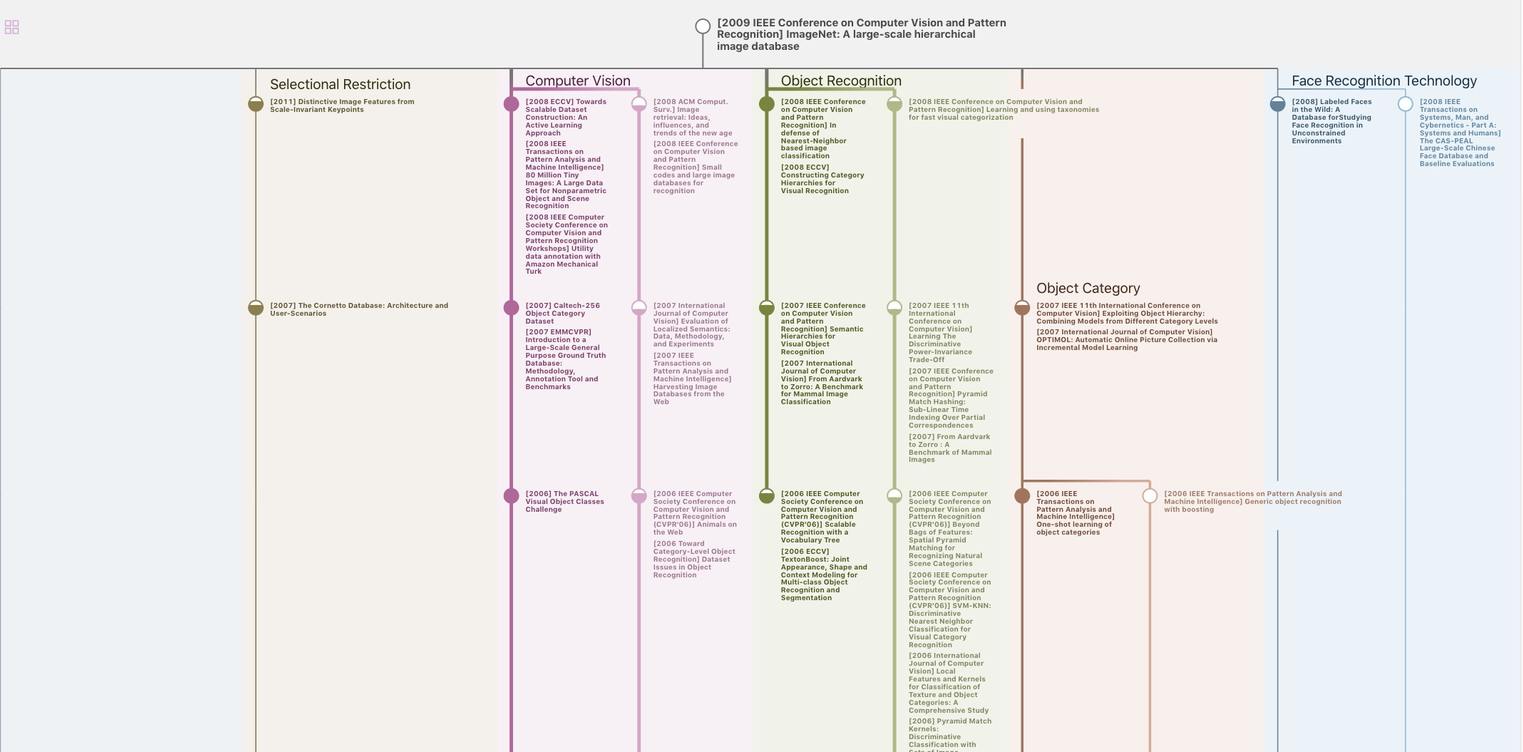
生成溯源树,研究论文发展脉络
Chat Paper
正在生成论文摘要