Leveraging Expert Guided Adversarial Augmentation For Improving Generalization in Named Entity Recognition
FINDINGS OF THE ASSOCIATION FOR COMPUTATIONAL LINGUISTICS (ACL 2022)(2022)
摘要
Named Entity Recognition (NER) systems often demonstrate great performance on in-distribution data, but perform poorly on examples drawn from a shifted distribution. One way to evaluate the generalization ability of NER models is to use adversarial examples, on which the specific variations associated with named entities are rarely considered. To this end, we propose leveraging expert-guided heuristics to change the entity tokens and their surrounding contexts thereby altering their entity types as adversarial attacks. Using expert-guided heuristics, we augmented the CoNLL 2003 test set and manually annotated it to construct a high-quality challenging set. We found that state-of-the-art NER systems trained on CoNLL 2003 training data drop performance dramatically on our challenging set. By training on adversarial augmented training examples and using mixup for regularization, we were able to significantly improve the performance on the challenging set as well as improve out-of-domain generalization which we evaluated by using OntoNotes data.
更多查看译文
关键词
expert guided adversarial augmentation,generalization
AI 理解论文
溯源树
样例
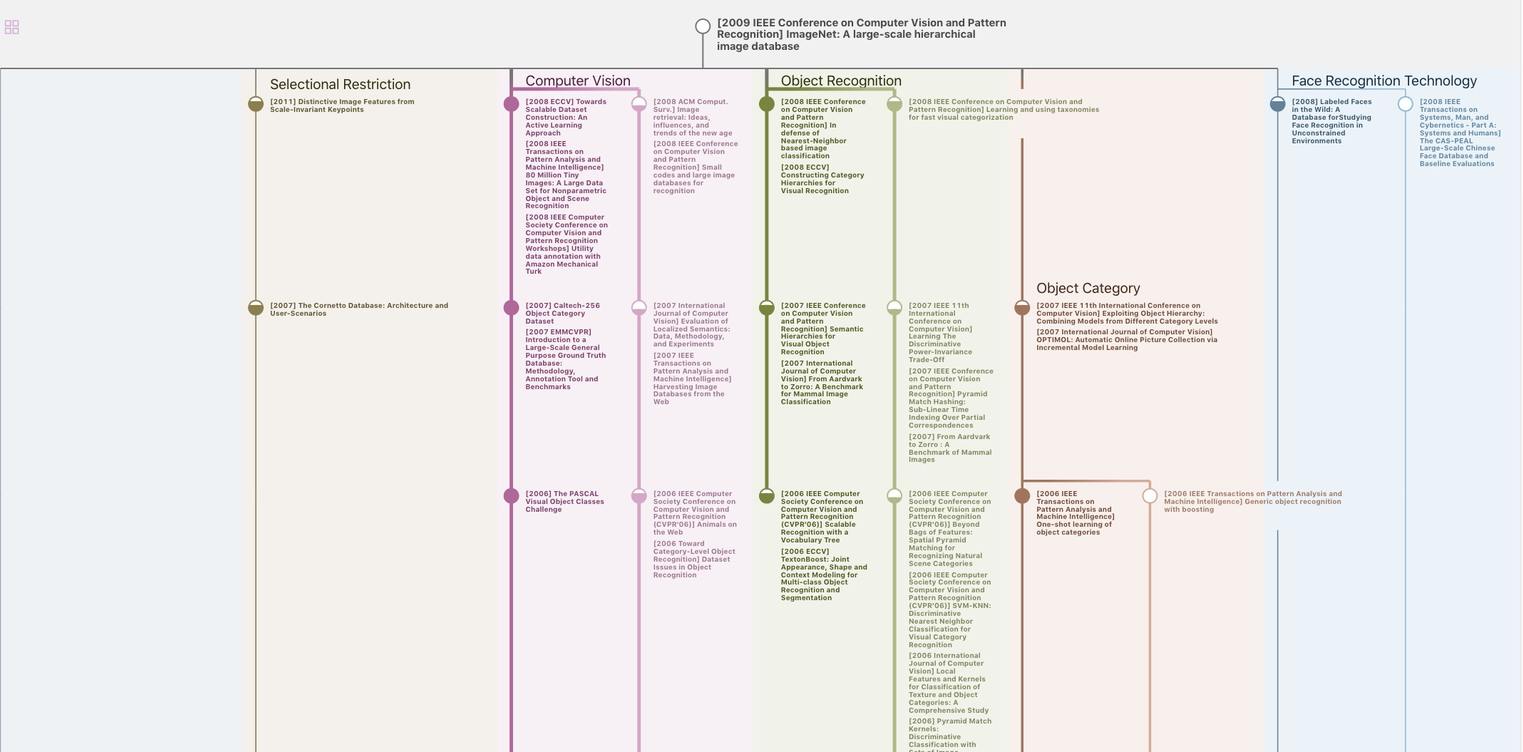
生成溯源树,研究论文发展脉络
Chat Paper
正在生成论文摘要