Data Smells: Categories, Causes and Consequences, and Detection of Suspicious Data in AI-based Systems
2022 IEEE/ACM 1st International Conference on AI Engineering – Software Engineering for AI (CAIN)(2022)
摘要
High data quality is fundamental for today’s AI-based systems. However, although data quality has been an object of research for decades, there is a clear lack of research on potential data quality issues (e.g., ambiguous, extraneous values). These kinds of issues are latent in nature and thus often not obvious. Nevertheless, they can be associated with an increased risk of future problems in AI-based systems (e.g., technical debt, data-induced faults). As a counterpart to code smells in software engineering, we refer to such issues as Data Smells. This article conceptualizes data smells and elaborates on their causes, consequences, detection, and use in the context of AI-based systems. In addition, a catalogue of 36 data smells divided into three categories (i.e., Believability Smells, Understandability Smells, Consistency Smells) is presented. Moreover, the article outlines tool support for detecting data smells and presents the result of an initial smell detection on more than 240 real-world datasets.
更多查看译文
关键词
data smells,artificial intelligence,data engineering,software engineering,data quality
AI 理解论文
溯源树
样例
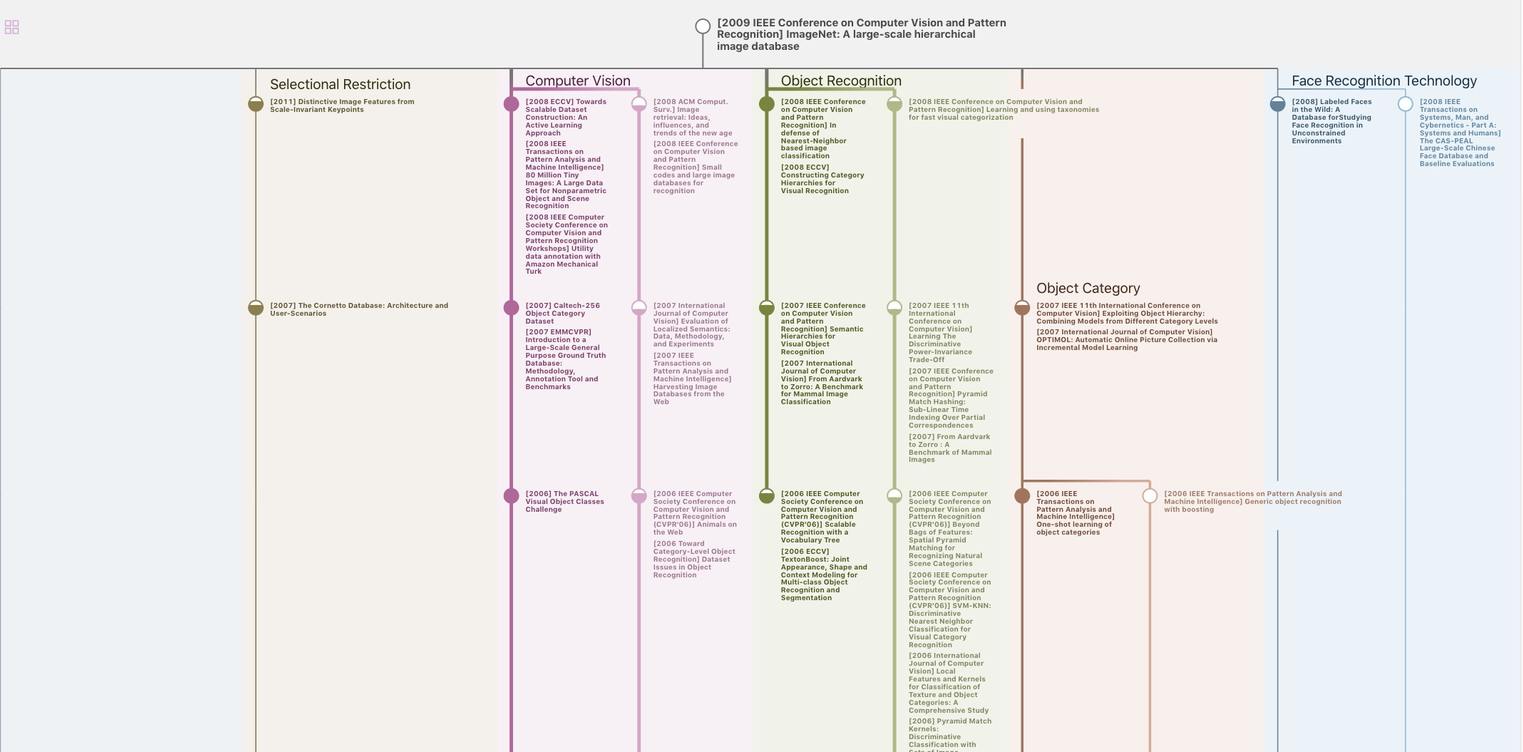
生成溯源树,研究论文发展脉络
Chat Paper
正在生成论文摘要