Perturbations in the Wild: Leveraging Human-Written Text Perturbations for Realistic Adversarial Attack and Defense
FINDINGS OF THE ASSOCIATION FOR COMPUTATIONAL LINGUISTICS (ACL 2022)(2022)
摘要
We proposes a novel algorithm, ANTHRO, that inductively extracts over 600K human-written text perturbations in the wild and leverages them for realistic adversarial attack. Unlike existing character-based attacks which often deductively hypothesize a set of manipulation strategies, our work is grounded on actual observations from real-world texts. We find that adversarial texts generated by ANTHRO achieve the best trade-off between (1) attack success rate, (2) semantic preservation of the original text, and (3) stealthiness-i.e. indistinguishable from human writings hence harder to be flagged as suspicious. Specifically, our attacks accomplished around 83% and 91% attack success rates on BERT and RoBERTa, respectively. Moreover, it outperformed the TextBugger baseline with an increase of 50% and 40% in terms of semantic preservation and stealthiness when evaluated by both layperson and professional human workers. ANTHRO can further enhance a BERT classifier's performance in understanding different variations of human-written toxic texts via adversarial training when compared to the Perspective API. Source code will be published at github.com/lethaiq/perturbations-in-the- wild.
更多查看译文
关键词
realistic adversarial attack,text perturbations,human-written
AI 理解论文
溯源树
样例
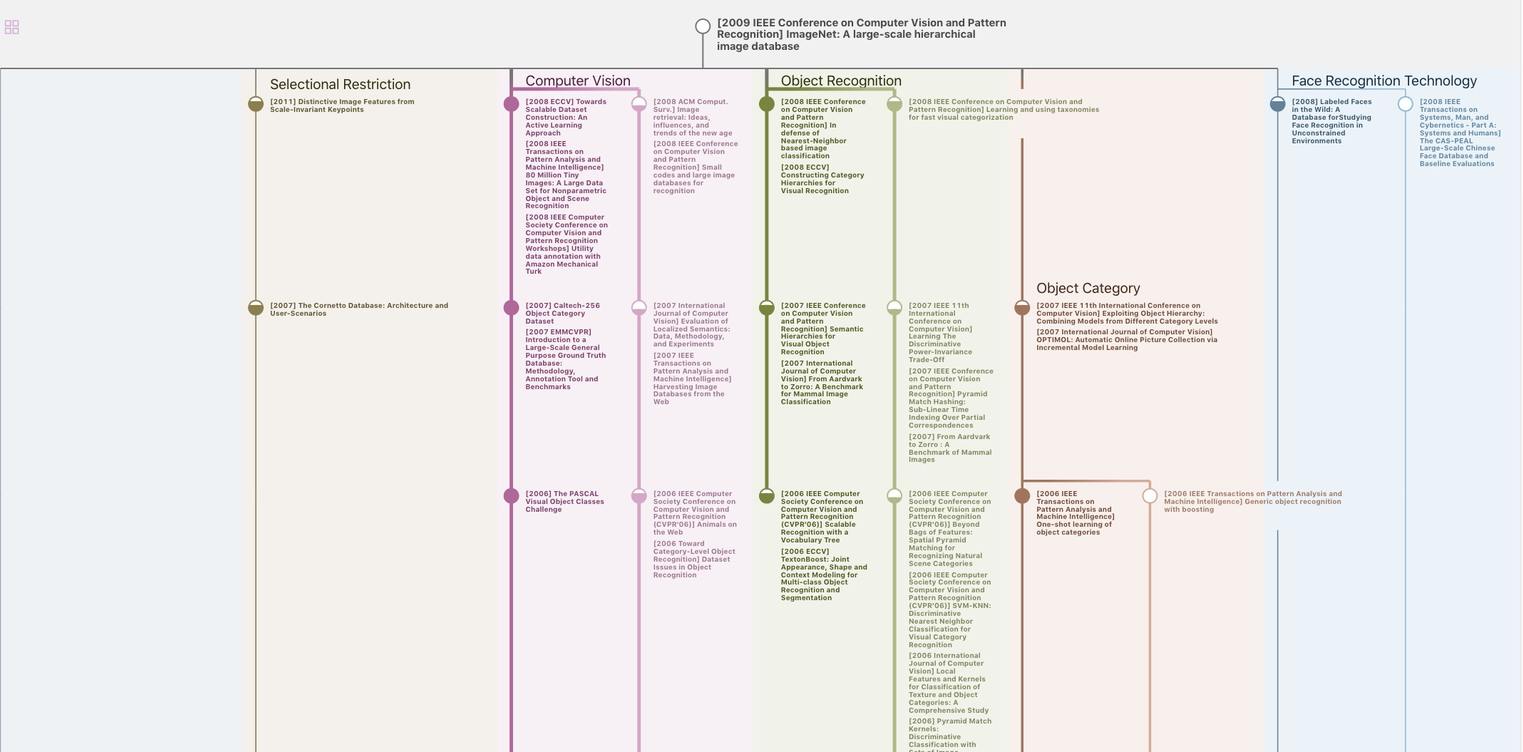
生成溯源树,研究论文发展脉络
Chat Paper
正在生成论文摘要