CodedVTR: Codebook-based Sparse Voxel Transformer with Geometric Guidance
IEEE Conference on Computer Vision and Pattern Recognition(2022)
摘要
Transformers have gained much attention by outperforming convolutional neural networks in many 2D vision tasks. However, they are known to have generalization problems and rely on massive-scale pre-training and sophisticated training techniques. When applying to 3D tasks, the irregular data structure and limited data scale add to the difficulty of transformer's application. We propose CodedVTR (Codebook-based Voxel TRansformer), which improves data efficiency and generalization ability for 3D sparse voxel transformers. On the one hand, we propose the codebook-based attention that projects an attention space into its subspace represented by the combination of “prototypes” in a learnable codebook. It regularizes attention learning and improves generalization. On the other hand, we propose geometry-aware self-attention that utilizes geometric information (geometric pattern, density) to guide attention learning. CodedVTR could be embedded into existing sparse convolution-based methods, and bring consistent performance improvements for indoor and outdoor 3D semantic segmentation tasks.
更多查看译文
关键词
Segmentation,grouping and shape analysis, Scene analysis and understanding
AI 理解论文
溯源树
样例
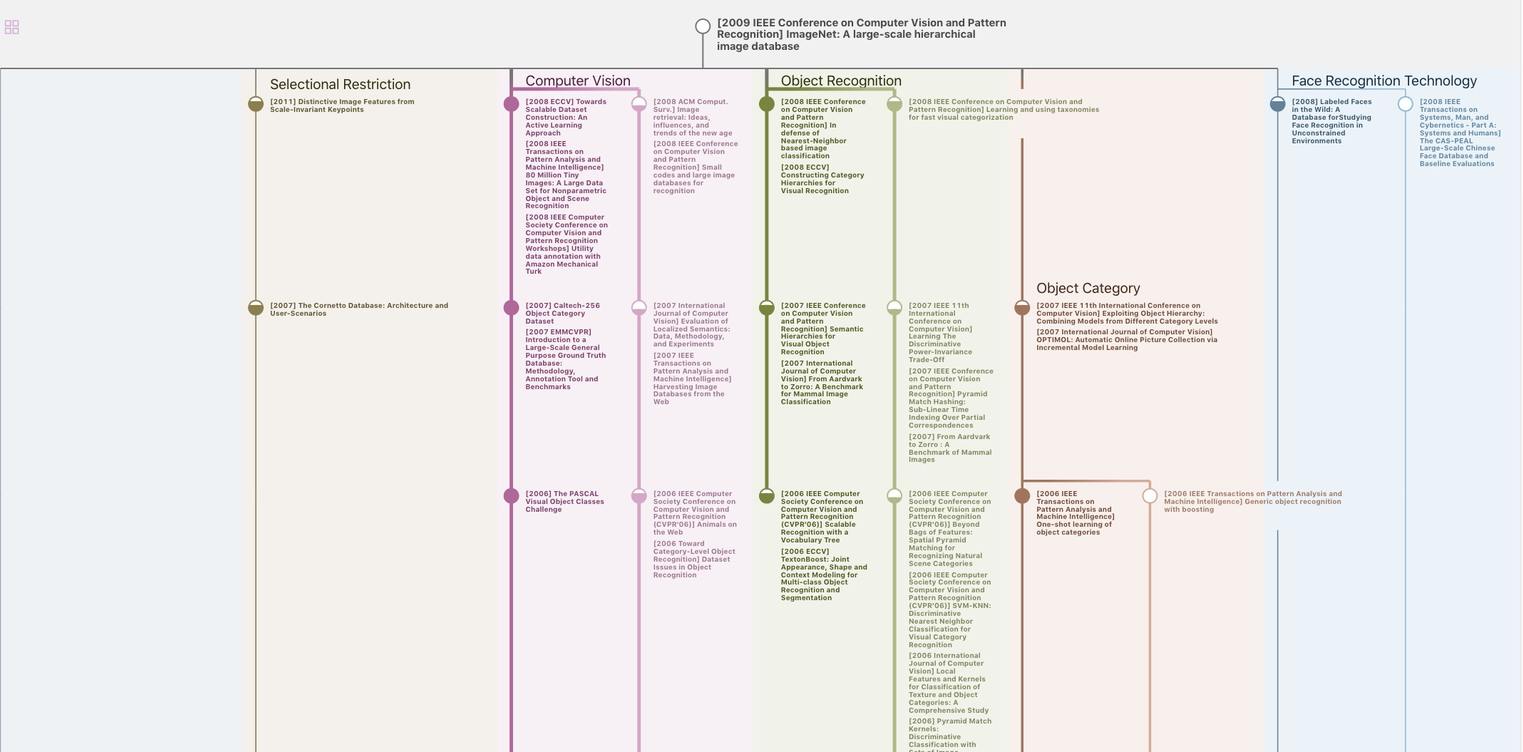
生成溯源树,研究论文发展脉络
Chat Paper
正在生成论文摘要