Integrating Prior Translation Knowledge Into Neural Machine Translation
IEEE/ACM Transactions on Audio, Speech and Language Processing(2022)
摘要
AbstractNeural machine translation (NMT), which is an encoder-decoder joint neural language model with an attention mechanism, has achieved impressive results on various machine translation tasks in the past several years. However, the language model attribute of NMT tends to produce fluent yet sometimes unfaithful translations, which hinders the improvement of translation capacity. In response to this problem, we propose a simple and efficient method to integrate prior translation knowledge into NMT in a universal manner that is compatible with neural networks. Meanwhile, it enables NMT to consider the crossing language translation knowledge from the source-side of the training pipeline of NMT, thereby making full use of the prior translation knowledge to enhance the performance of NMT. The experimental results on two large-scale benchmark translation tasks demonstrated that our approach achieved a significant improvement over a strong baseline.
更多查看译文
关键词
Machine translation, Knowledge representation, Training, Transformers, Speech processing, Decoding, Task analysis, Bilingual lexicon knowledge, prior knowledge representation, self-attention networks, machine translation
AI 理解论文
溯源树
样例
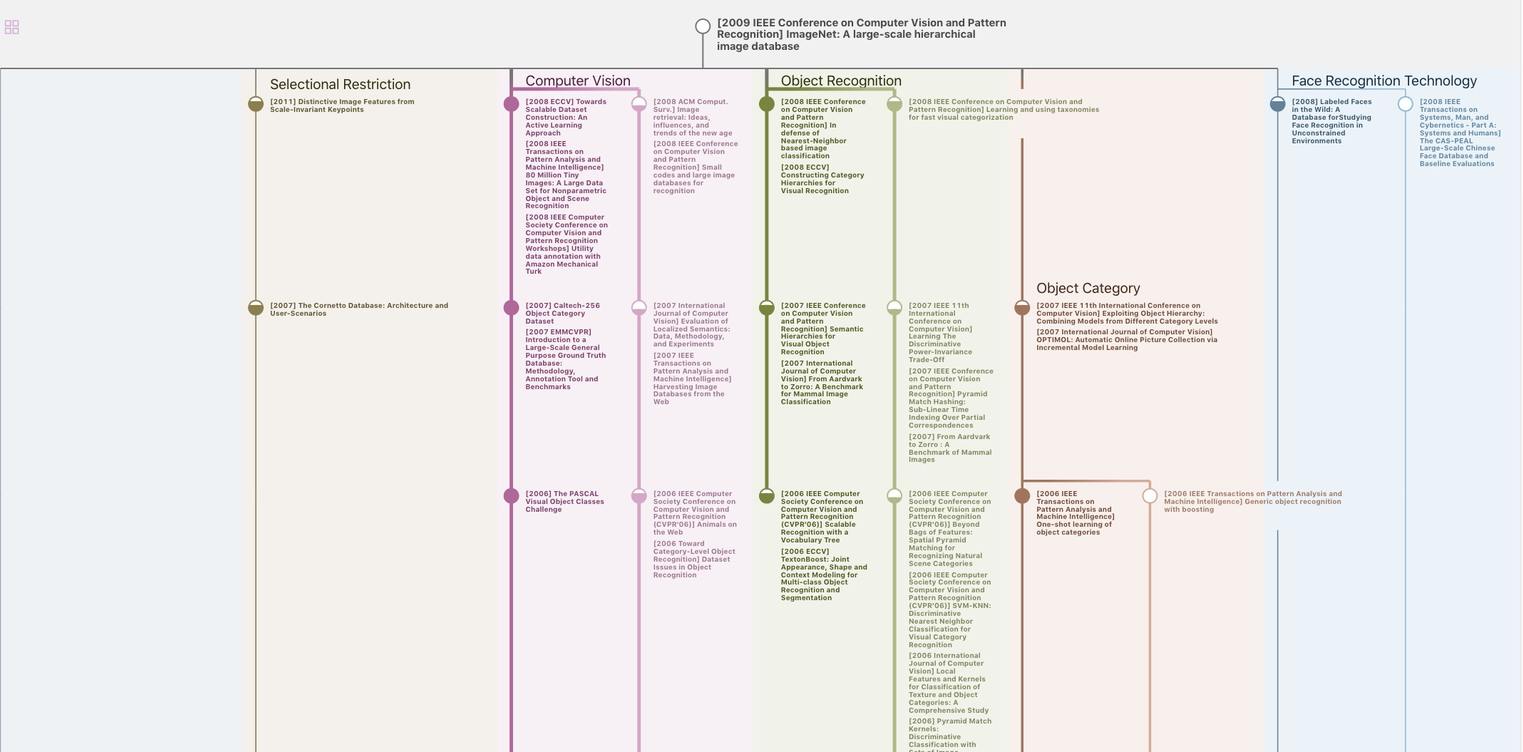
生成溯源树,研究论文发展脉络
Chat Paper
正在生成论文摘要