Live Streaming Speech Recognition Using Deep Bidirectional LSTM Acoustic Models and Interpolated Language Models
IEEE/ACM Transactions on Audio, Speech and Language Processing(2022)
摘要
AbstractAlthough Long-Short Term Memory (LSTM) networks and deep Transformers are now extensively used in offline ASR, it is unclear how best offline systems can be adapted to work with them under the streaming setup. After gaining considerable experience on this regard in recent years, in this paper we show how an optimized, low-latency streaming decoder can be built in which bidirectional LSTM acoustic models, together with general interpolated language models, can be nicely integrated with minimal perfomance degradation. In brief, our streaming decoder consists of a one-pass, real-time search engine relying on a limited-duration window sliding over time and a number of ad hoc acoustic and language model pruning techniques. Extensive empirical assessment is provided on truly streaming tasks derived from the well-known LibriSpeech and TED talks datasets, as well as from TV shows on a main Spanish broadcasting station.
更多查看译文
关键词
Acoustics, Decoding, Adaptation models, Hidden Markov models, Task analysis, Speech recognition, Computational modeling, Automatic speech recognition, streaming, decoding, acoustic modeling, language modeling, neural networks
AI 理解论文
溯源树
样例
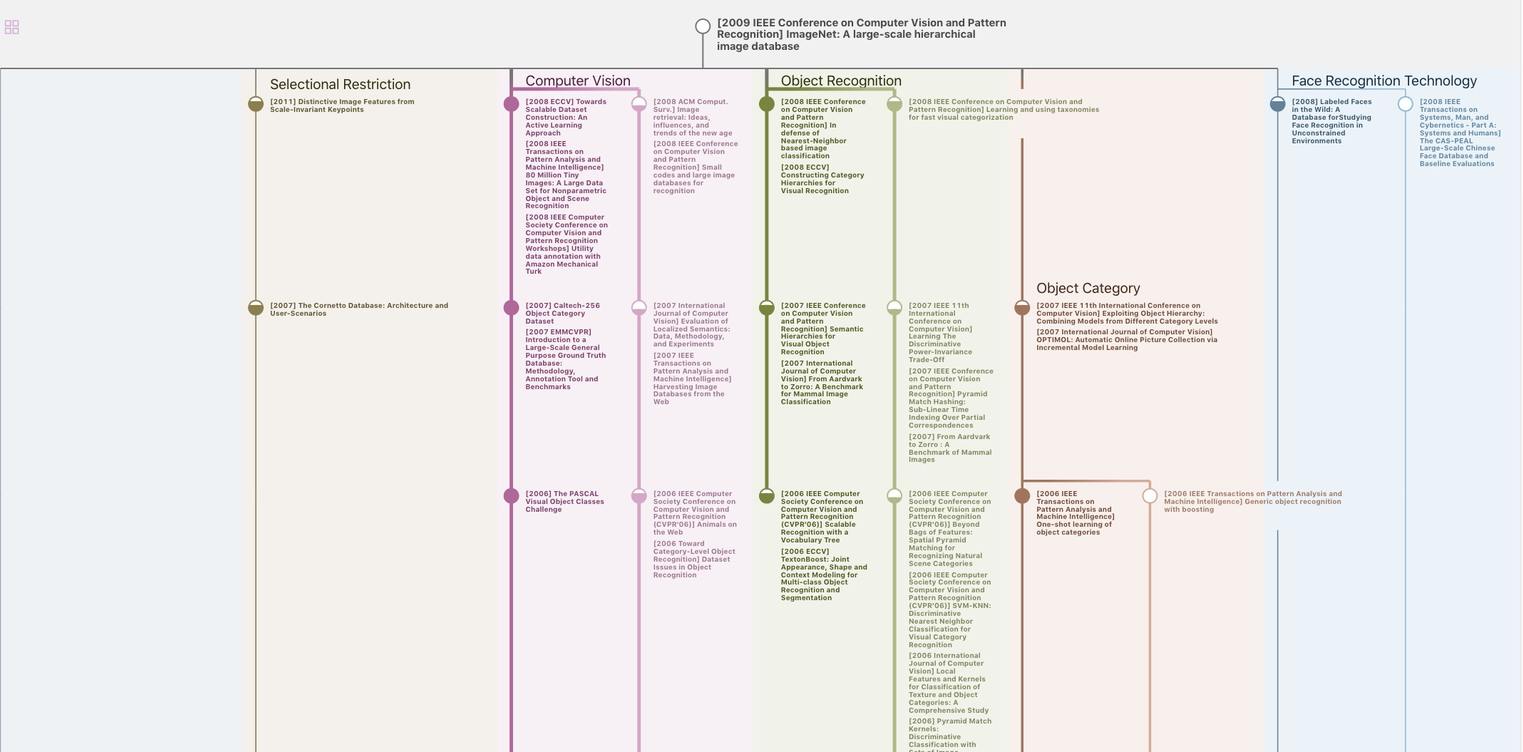
生成溯源树,研究论文发展脉络
Chat Paper
正在生成论文摘要