HGEN: Learning Hierarchical Heterogeneous Graph Encoding for Math Word Problem Solving
IEEE/ACM Transactions on Audio, Speech, and Language Processing(2022)
摘要
Designing algorithms to solve math word problems (MWPs) is an important research topic in natural language processing and smart education domains. The task of solving MWPs involves transforming math problem texts into math equations. Although recent Graph2Tree-based models, which adopt homogeneous graph encoders to learn quantity representations, have obtained very promising results in generating math equations, they do not consider the heterogeneous issue and the long-distance dependencies of heterogeneous nodes. In this paper, we propose a novel hierarchical heterogeneous graph encoding called HGEN for MWPs. Specifically, HGEN first introduces a heterogeneous graph consisting of a node-level attention layer and a type-aware attention layer to learn the heterogeneous node embedding. HGEN then captures the long-distance dependent information by propagating the multi-hop nodes in a hierarchical manner. We conduct extensive experiments on two popular MWP datasets. Our empirical results show that HGEN significantly outperforms the state-of-the-art Graph2Tree-based models in the literature.
更多查看译文
关键词
Math word problem,natural language processing,text mining,representation learning
AI 理解论文
溯源树
样例
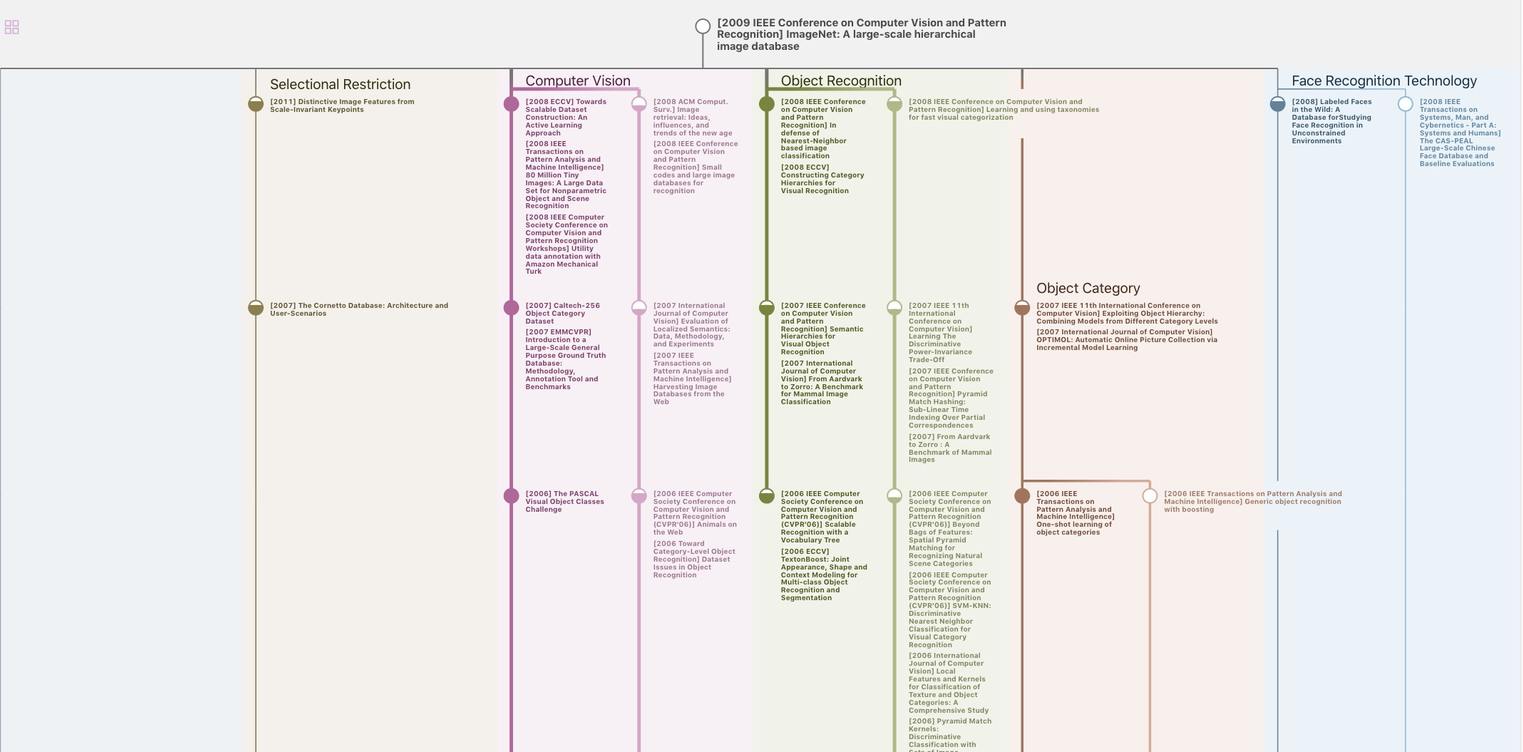
生成溯源树,研究论文发展脉络
Chat Paper
正在生成论文摘要