Mutation-Attention (MuAt): deep representation learning of somatic mutations for tumour typing and subtyping
biorxiv(2023)
摘要
Background Cancer genome sequencing enables accurate classification of tumours and tumour subtypes. However, prediction performance is still limited using exome-only sequencing and for tumour types with low somatic mutation burden such as many paediatric tumours. Moreover, the ability to leverage deep representation learning in discovery of tumour entities remains unknown. Methods We introduce here Mutation-Attention (MuAt), a deep neural network to learn representations of simple and complex somatic alterations for prediction of tumour types and subtypes. In contrast to many previous methods, MuAt utilizes the attention mechanism on individual mutations instead of aggregated mutation counts. Results We trained MuAt models on 2587 whole cancer genomes (24 tumour types) from the Pan-Cancer Analysis of Whole Genomes (PCAWG) and 7352 cancer exomes (20 types) from the Cancer Genome Atlas (TCGA). MuAt achieved prediction accuracy of 89% for whole genomes and 64% for whole exomes, and a top-5 accuracy of 97% and 90%, respectively. MuAt models were found to be well-calibrated and perform well in three independent whole cancer genome cohorts with 10,361 tumours in total. We show MuAt to be able to learn clinically and biologically relevant tumour entities including acral melanoma, SHH-activated medulloblastoma, SPOP -associated prostate cancer, microsatellite instability, POLE proofreading deficiency, and MUTYH -associated pancreatic endocrine tumours without these tumour subtypes and subgroups being provided as training labels. Finally, scrunity of MuAt attention matrices revealed both ubiquitous and tumour-type specific patterns of simple and complex somatic mutations. Conclusions Integrated representations of somatic alterations learnt by MuAt were able to accurately identify histological tumour types and identify tumour entities, with potential to impact precision cancer medicine.
更多查看译文
关键词
Cancer genomics,Precision cancer medicine,Machine learning,Deep learning,Tumour type prediction,Molecular tumour subtyping,Somatic mutations,Deep neural networks,Whole genome sequencing,Whole exome sequencing
AI 理解论文
溯源树
样例
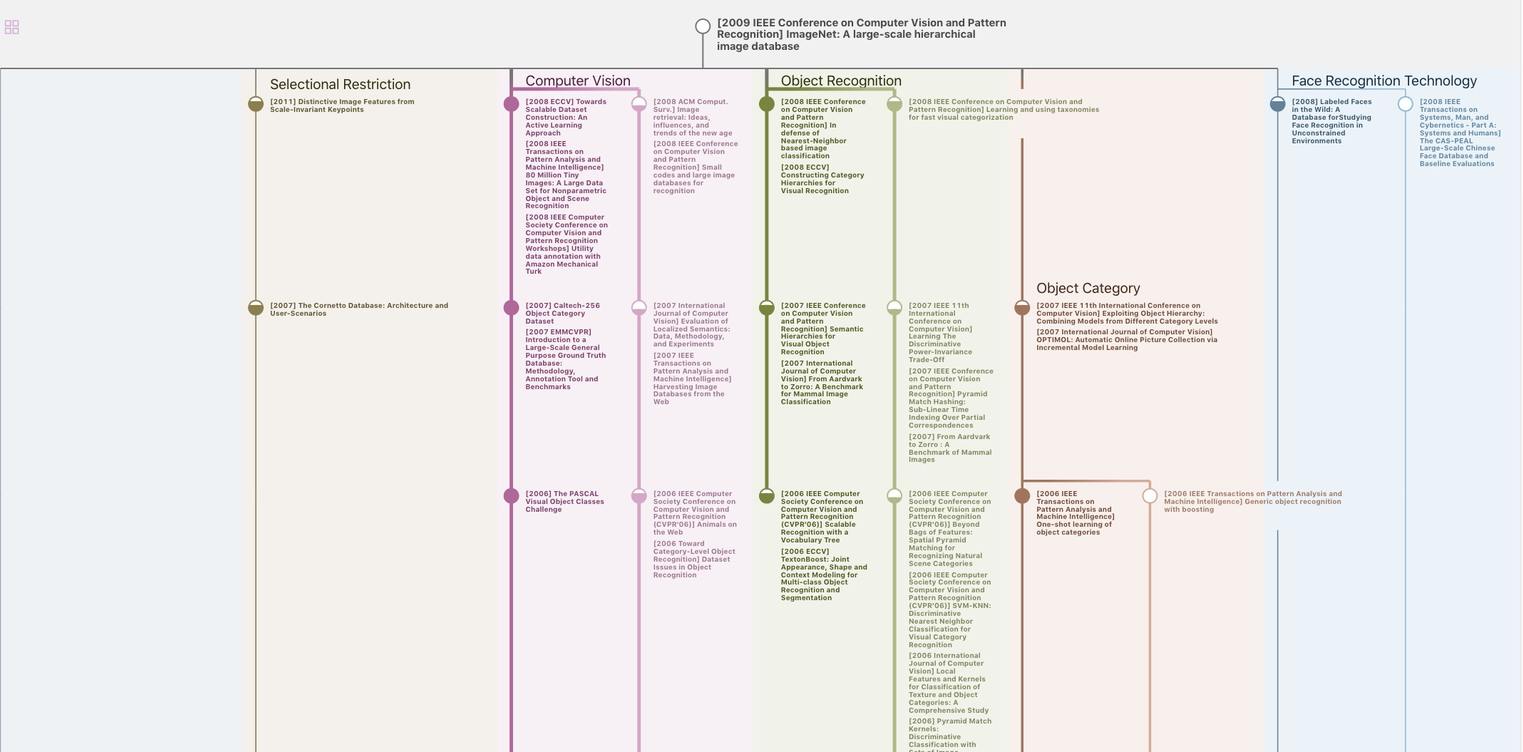
生成溯源树,研究论文发展脉络
Chat Paper
正在生成论文摘要