AutoSDF: Shape Priors for 3D Completion, Reconstruction and Generation
2022 IEEE/CVF Conference on Computer Vision and Pattern Recognition (CVPR)(2022)
摘要
Powerful priors allow us to perform inference with in-sufficient information. In this paper, we propose an au-toregressive prior for 3D shapes to solve multimodal 3D tasks such as shape completion, reconstruction, and gener-ation. We model the distribution over 3D shapes as a non-sequential autoregressive distribution over a discretized, low-dimensional, symbolic grid-like latent representation of 3D shapes. This enables us to represent distributions over 3D shapes conditioned on information from an arbitrary set of spatially anchored query locations and thus perform shape completion in such arbitrary settings (e.g. generating a complete chair given only a view of the back leg). We also show that the learned autoregressive prior can be leveraged for conditional tasks such as single-view reconstruction and language-based generation. This is achieved by learning task-specific ‘naive’ conditionals which can be approxi-mated by light-weight models trained on minimal paired data. We validate the effectiveness of the proposed method using both quantitative and qualitative evaluation and show that the proposed method outperforms the specialized state-of-the-art methods trained for individual tasks. The project page with code and video visualizations can be found at https://yccyenchicheng.github.io/AutoSDF/.
更多查看译文
关键词
Machine learning, 3D from single images, Representation learning, Vision + language
AI 理解论文
溯源树
样例
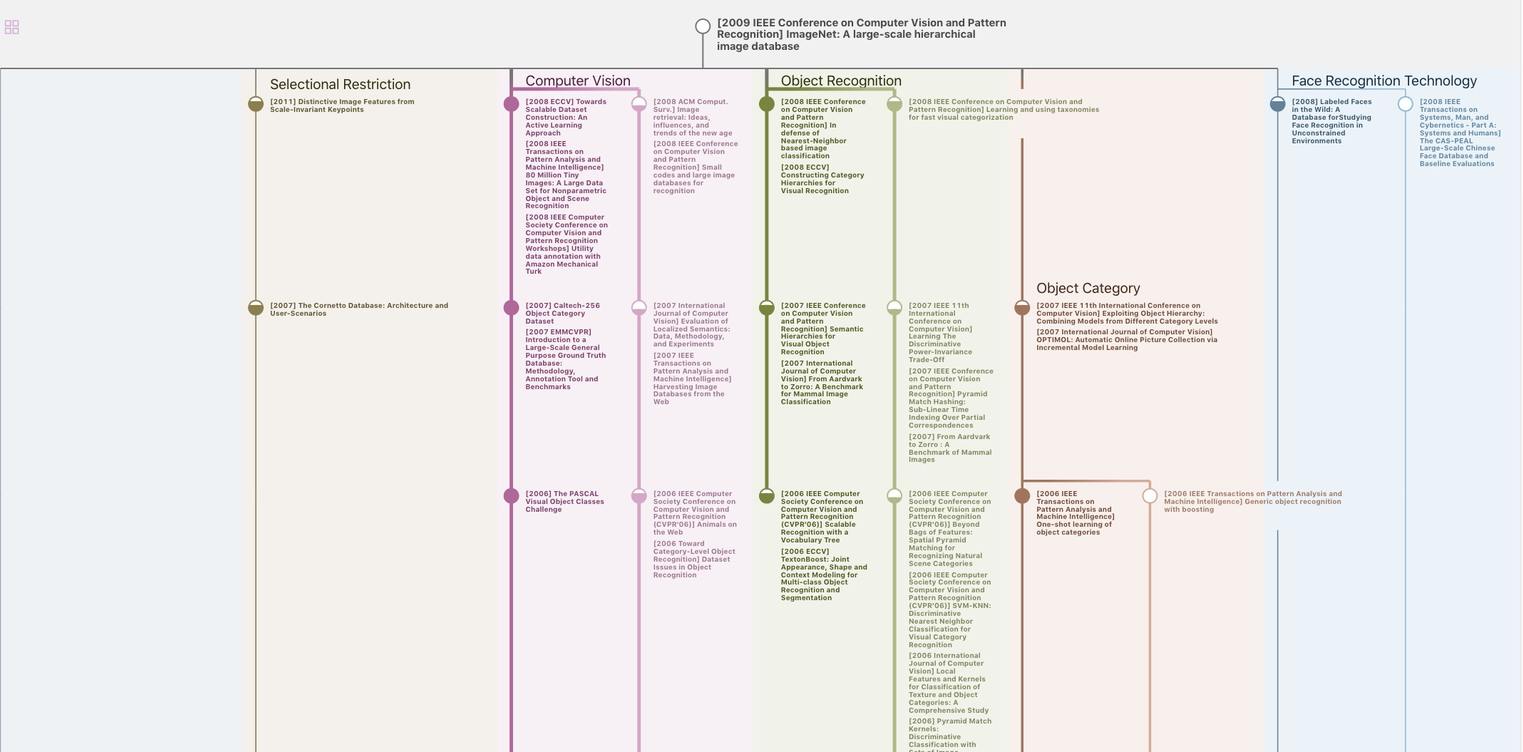
生成溯源树,研究论文发展脉络
Chat Paper
正在生成论文摘要