Universal Conditional Masked Language Pre-training for Neural Machine Translation
PROCEEDINGS OF THE 60TH ANNUAL MEETING OF THE ASSOCIATION FOR COMPUTATIONAL LINGUISTICS (ACL 2022), VOL 1: (LONG PAPERS)(2022)
摘要
Pre-trained sequence-to-sequence models have significantly improved Neural Machine Translation (NMT). Different from prior works where pre-trained models usually adopt an unidirectional decoder, this paper demonstrates that pre-training a sequence-to-sequence model but with a bidirectional decoder can produce notable performance gains for both Autoregressive and Non-autoregressive NMT. Specifically, we propose CeMAT, a conditional masked language model pre-trained on large-scale bilingual and monolingual corpora in many languages.(1) We also introduce two simple but effective methods to enhance the CeMAT, aligned code-switching & masking and dynamic dual-masking. We conduct extensive experiments and show that our CeMAT can achieve significant performance improvement for all scenarios from low- to extremely high-resource languages, i.e., up to +14.4 BLEU on low-resource and +7.9 BLEU on average for Autoregressive NMT. For Non-autoregressive NMT, we demonstrate it can also produce consistent performance gains, i.e., up to +5.3 BLEU. To the best of our knowledge, this is the first work to pre-train a unified model for fine-tuning on both NMT tasks.
更多查看译文
关键词
translation,language,neural machine
AI 理解论文
溯源树
样例
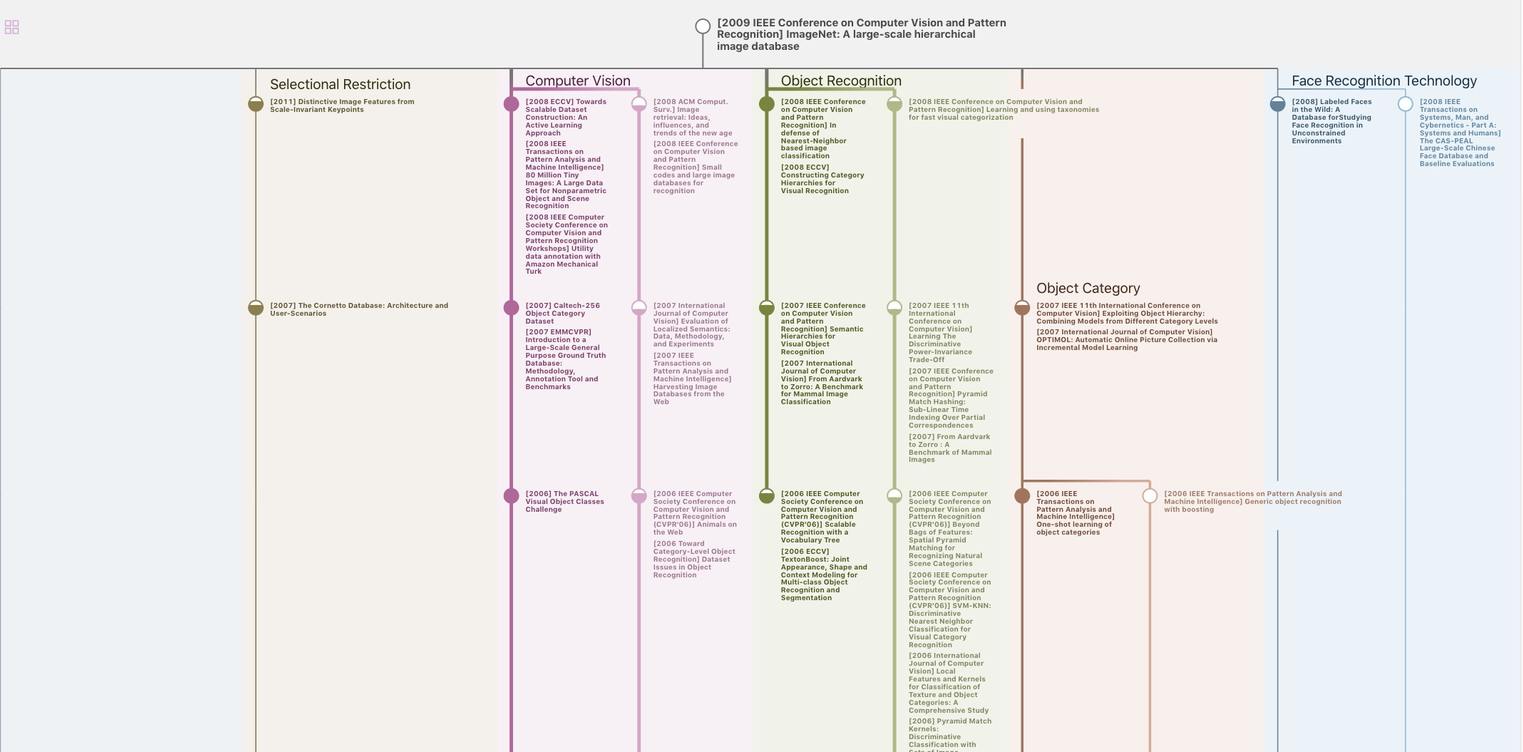
生成溯源树,研究论文发展脉络
Chat Paper
正在生成论文摘要