Neural Compression-Based Feature Learning for Video Restoration
IEEE Conference on Computer Vision and Pattern Recognition(2022)
摘要
How to efficiently utilize the temporal features is crucial, yet challenging, for video restoration. The temporal features usually contain various noisy and uncorrelated information, and they may interfere with the restoration of the current frame. This paper proposes learning noiserobust feature representations to help video restoration. We are inspired by that the neural codec is a natural denoiser: In neural codec, the noisy and uncorrelated contents which are hard to predict but cost lots of bits are more inclined to be discarded for bitrate saving. Therefore, we design a neural compression module to filter the noise and keep the most useful information in features for video restoration. To achieve robustness to noise, our compression module adopts a spatial-channel-wise quantization mechanism to adaptively determine the quantization step size for each position in the latent. Experiments show that our method can significantly boost the performance on video denoising, where we obtain 0.13 dB improvement over BasicVSR++ with only 0.23x FLOPs. Meanwhile, our method also obtains SOTA results on video deraining and dehazing.
更多查看译文
关键词
Low-level vision
AI 理解论文
溯源树
样例
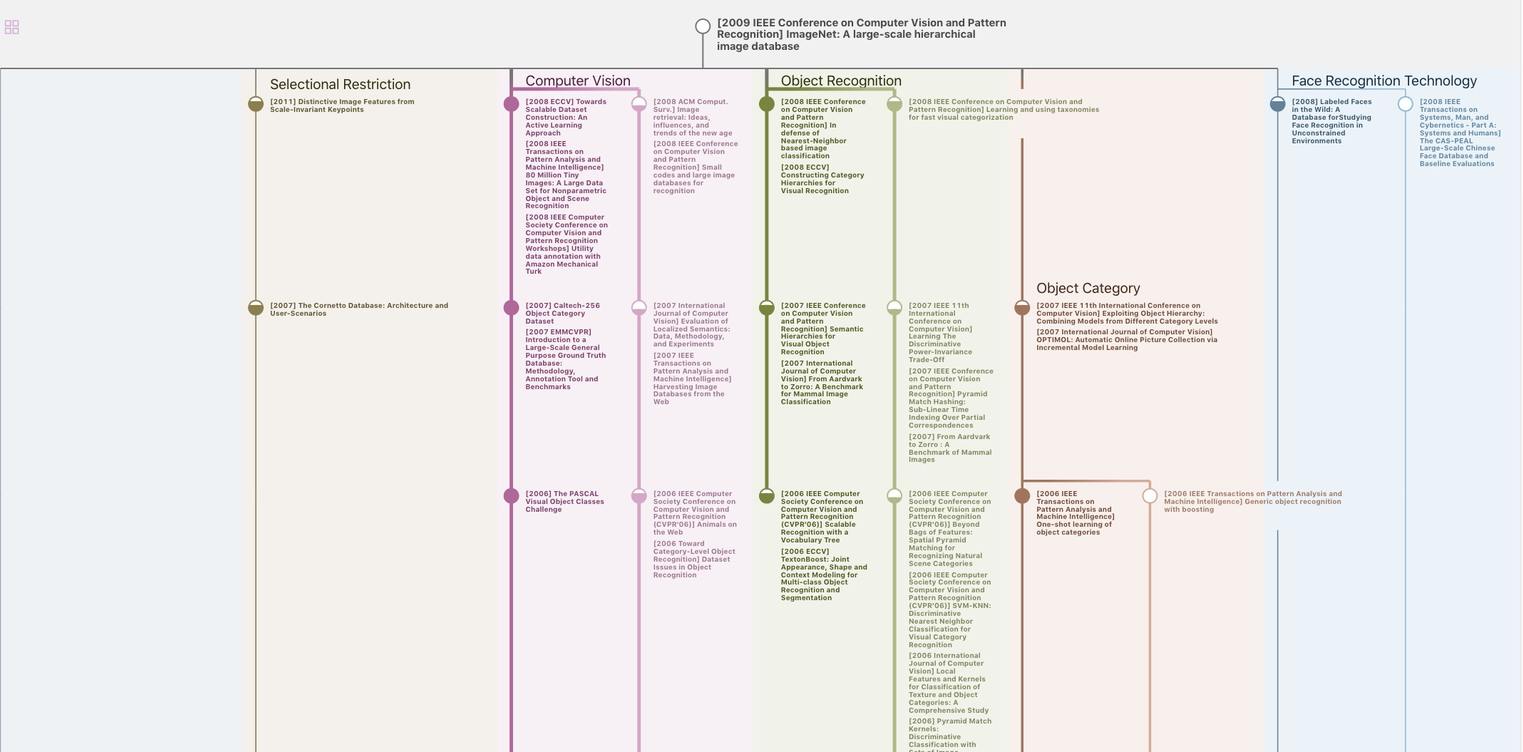
生成溯源树,研究论文发展脉络
Chat Paper
正在生成论文摘要