Dual-domain Attention-based Deep Network for Sparse-view CT Artifact Reduction
arxiv(2022)
摘要
Due to the wide applications of X-ray computed tomography (CT) in medical imaging activities, radiation exposure has become a major concern for public health. Sparse-view CT is a promising approach to reduce the radiation dose by down-sampling the total number of acquired projections. However, the CT images reconstructed by this sparse-view imaging approach suffer from severe streaking artifacts and structural information loss. In this work, an end-to-end dual-domain attention-based deep network (DDANet) is proposed to solve such an ill-posed CT image reconstruction problem. The image-domain CT image and the projection-domain sinogram are put into the two parallel sub-networks of the DDANet to independently extract the distinct high-level feature maps. In addition, a specified attention module is introduced to fuse the aforementioned dual-domain feature maps to allow complementary optimizations of removing the streaking artifacts and mitigating the loss of structure. Numerical simulations, anthropomorphic thorax phantom and in vivo pre-clinical experiments are conducted to verify the sparse-view CT imaging performance of the DDANet. Results demonstrate that this newly developed approach is able to robustly remove the streaking artifacts while maintaining the fine structures. As a result, the DDANet provides a promising solution in achieving high quality sparse-view CT imaging.
更多查看译文
AI 理解论文
溯源树
样例
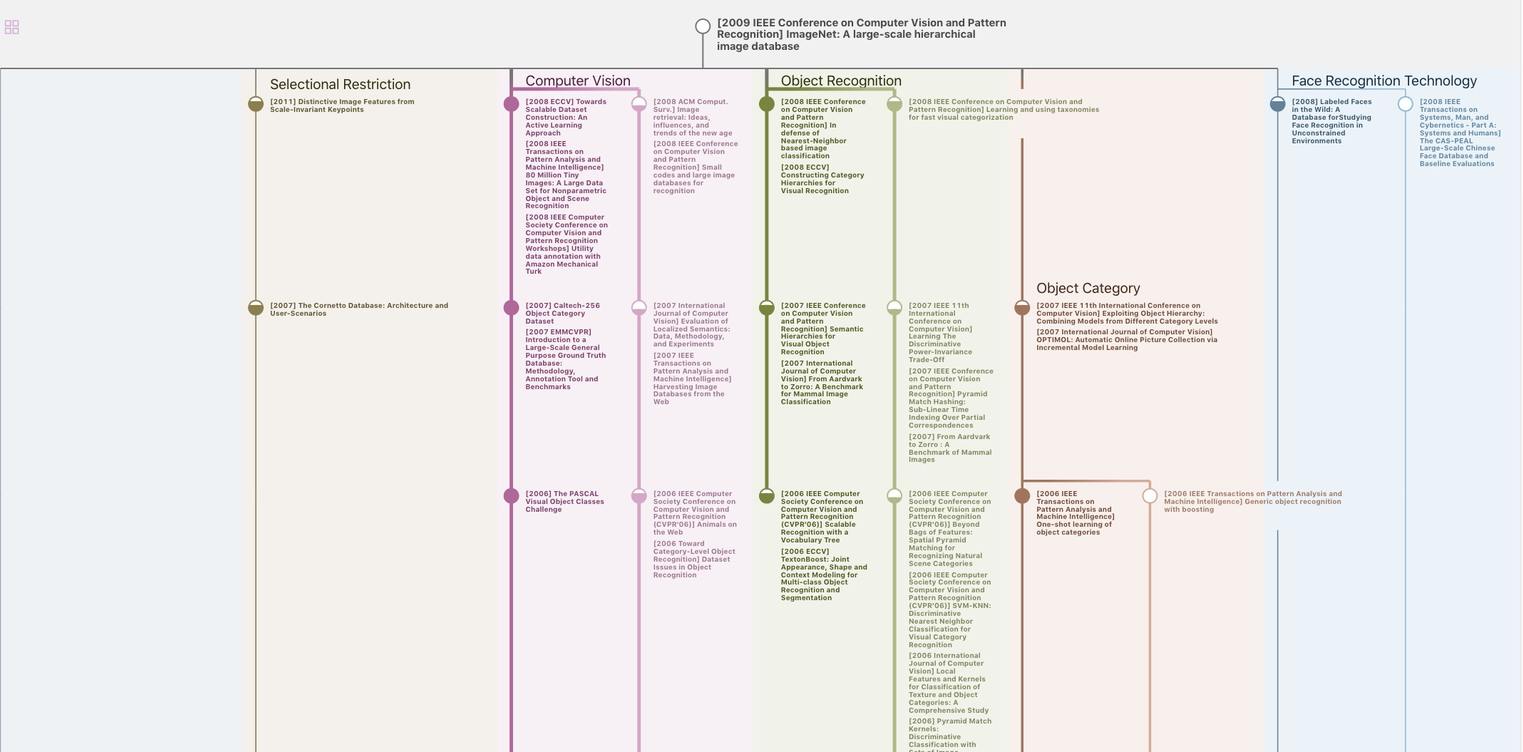
生成溯源树,研究论文发展脉络
Chat Paper
正在生成论文摘要