DU-VLG: Unifying Vision-and-Language Generation via Dual Sequence-to-Sequence Pre-training
FINDINGS OF THE ASSOCIATION FOR COMPUTATIONAL LINGUISTICS (ACL 2022)(2022)
摘要
Due to the limitations of the model structure and pre-training objectives, existing vision-and-language generation models cannot utilize pairwise images and text through bi-directional generation. In this paper, we propose DU-VLG, a framework which unifies vision-and-language generation as sequence generation problems. DU-VLG is trained with novel dual pre-training tasks: multi-modal denoising autoencoder tasks and modality translation tasks. To bridge the gap between image understanding and generation, we further design a novel commitment loss. We compare pre-training objectives on image captioning and text-to-image generation datasets. Results show that DU-VLG yields better performance than variants trained with uni-directional generation objectives or the variant without the commitment loss. On the image captioning task, our model reaches better performance than other pre-trained systems. On text-to-image generation datasets, our model achieves better or comparable results than previous state-of-the-art models. In addition, human judges further confirm that our model generates real and relevant images as well as faithful and informative captions.
更多查看译文
关键词
generation,du-vlg,vision-and-language,sequence-to-sequence,pre-training
AI 理解论文
溯源树
样例
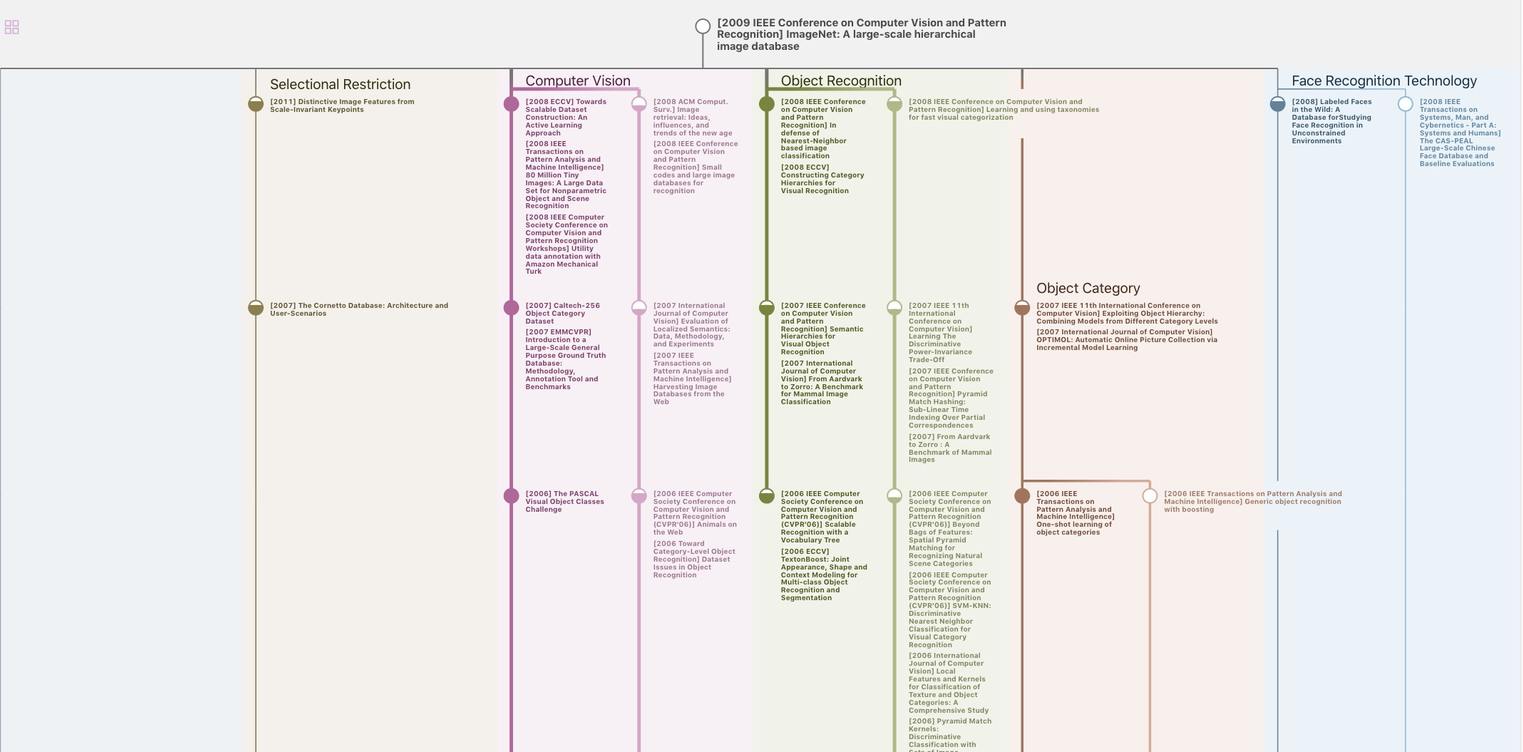
生成溯源树,研究论文发展脉络
Chat Paper
正在生成论文摘要