Hybrid Pixel-Unshuffled Network for Lightweight Image Super-Resolution
arxiv(2022)
摘要
Convolutional neural network (CNN) has achieved great success on image super-resolution (SR). However, most deep CNN-based SR models take massive computations to obtain high performance. Downsampling features for multi-resolution fusion is an efficient and effective way to improve the performance of visual recognition. Still, it is counter-intuitive in the SR task, which needs to project a low-resolution input to high-resolution. In this paper, we propose a novel Hybrid Pixel-Unshuffled Network (HPUN) by introducing an efficient and effective downsampling module into the SR task. The network contains pixel-unshuffled downsampling and Self-Residual Depthwise Separable Convolutions. Specifically, we utilize pixel-unshuffle operation to downsample the input features and use grouped convolution to reduce the channels. Besides, we enhance the depthwise convolution's performance by adding the input feature to its output. Experiments on benchmark datasets show that our HPUN achieves and surpasses the state-of-the-art reconstruction performance with fewer parameters and computation costs.
更多查看译文
关键词
network,image,pixel-unshuffled,super-resolution
AI 理解论文
溯源树
样例
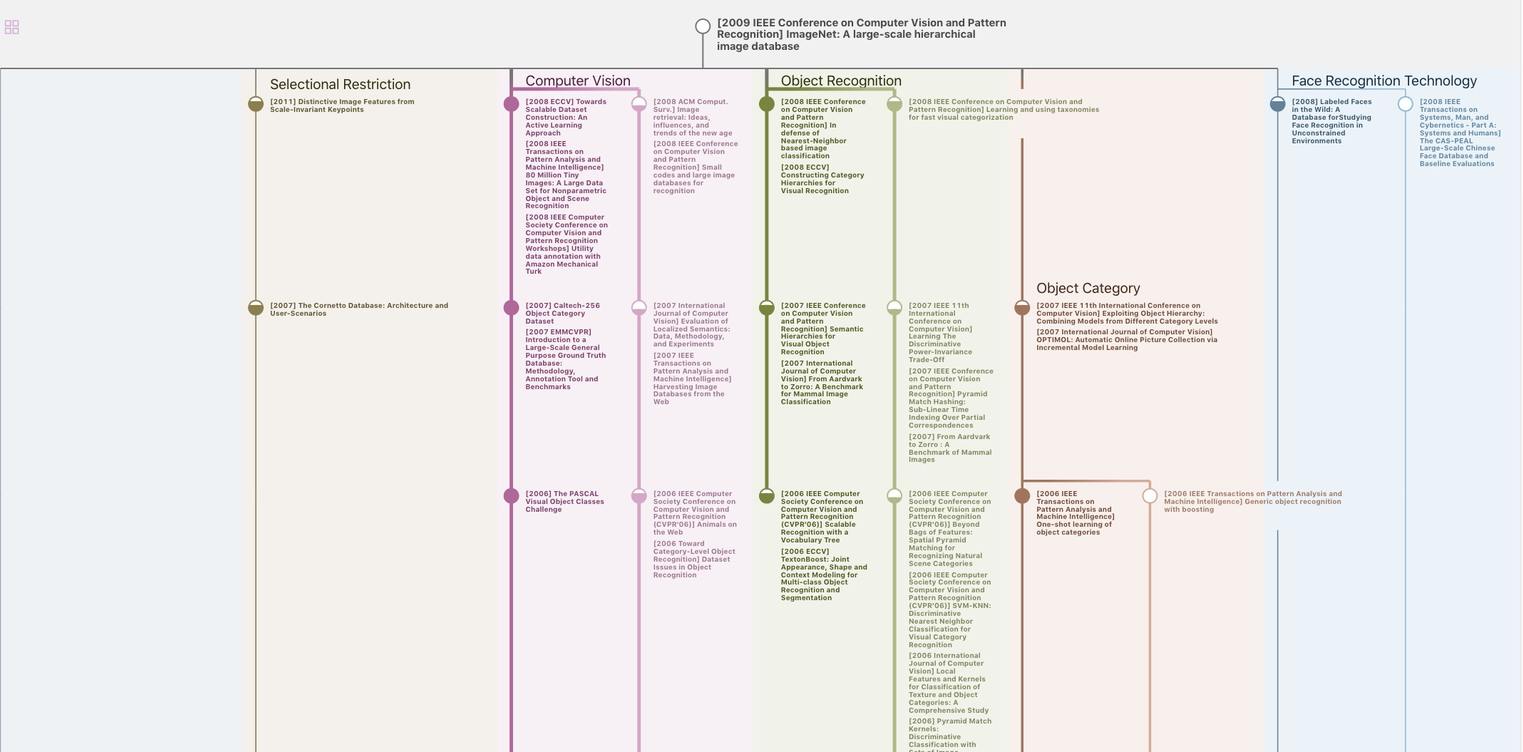
生成溯源树,研究论文发展脉络
Chat Paper
正在生成论文摘要