Learning deep representations of enzyme thermal adaptation.
Protein science : a publication of the Protein Society(2022)
摘要
Temperature is a fundamental environmental factor that shapes the evolution of organisms. Learning thermal determinants of protein sequences in evolution thus has profound significance for basic biology, drug discovery, and protein engineering. Here, we use a data set of over 3 million BRENDA enzymes labeled with optimal growth temperatures (OGTs) of their source organisms to train a deep neural network model (DeepET). The protein-temperature representations learned by DeepET provide a temperature-related statistical summary of protein sequences and capture structural properties that affect thermal stability. For prediction of enzyme optimal catalytic temperatures and protein melting temperatures via a transfer learning approach, our DeepET model outperforms classical regression models trained on rationally designed features and other deep-learning-based representations. DeepET thus holds promise for understanding enzyme thermal adaptation and guiding the engineering of thermostable enzymes.
更多查看译文
关键词
bioinformatics,deep neural networks,enzyme catalytic temperatures,optimal growth temperatures,protein thermostability,transfer learning
AI 理解论文
溯源树
样例
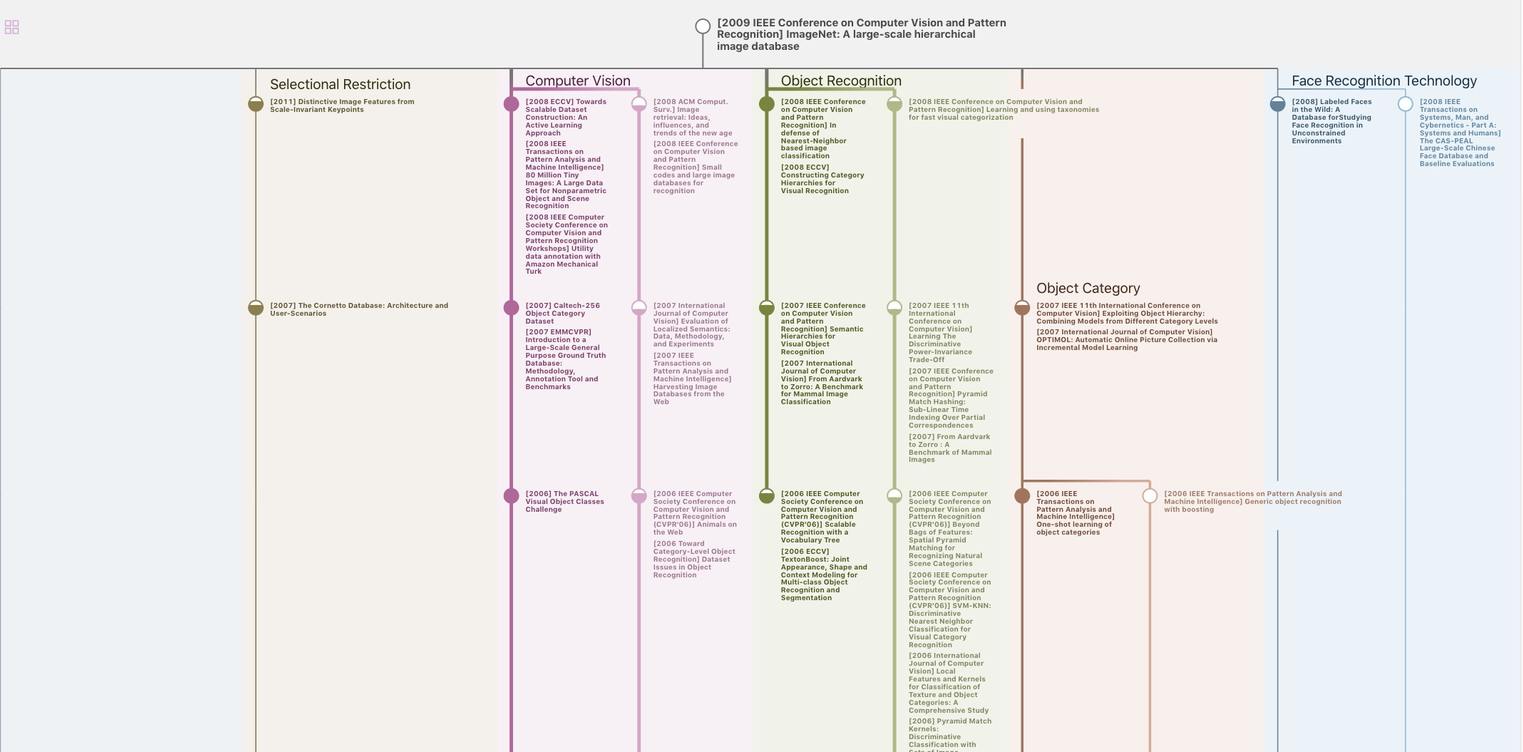
生成溯源树,研究论文发展脉络
Chat Paper
正在生成论文摘要