Object Discovery and Representation Networks.
European Conference on Computer Vision(2022)
摘要
The promise of self-supervised learning (SSL) is to leverage large amounts of unlabeled data to solve complex tasks. While there has been excellent progress with simple, image-level learning, recent methods have shown the advantage of including knowledge of image structure. However, by introducing hand-crafted image segmentations to define regions of interest, or specialized augmentation strategies, these methods sacrifice the simplicity and generality that makes SSL so powerful. Instead, we propose a self-supervised learning paradigm that discovers this image structure by itself. Our method, Odin, couples object discovery and representation networks to discover meaningful image segmentations without any supervision. The resulting learning paradigm is simpler, less brittle, and more general, and achieves state-of-the-art transfer learning results for object detection and instance segmentation on COCO, and semantic segmentation on PASCAL and Cityscapes, while strongly surpassing supervised pre-training for video segmentation on DAVIS.
更多查看译文
关键词
Self-supervised learning,Object discovery,Transfer learning
AI 理解论文
溯源树
样例
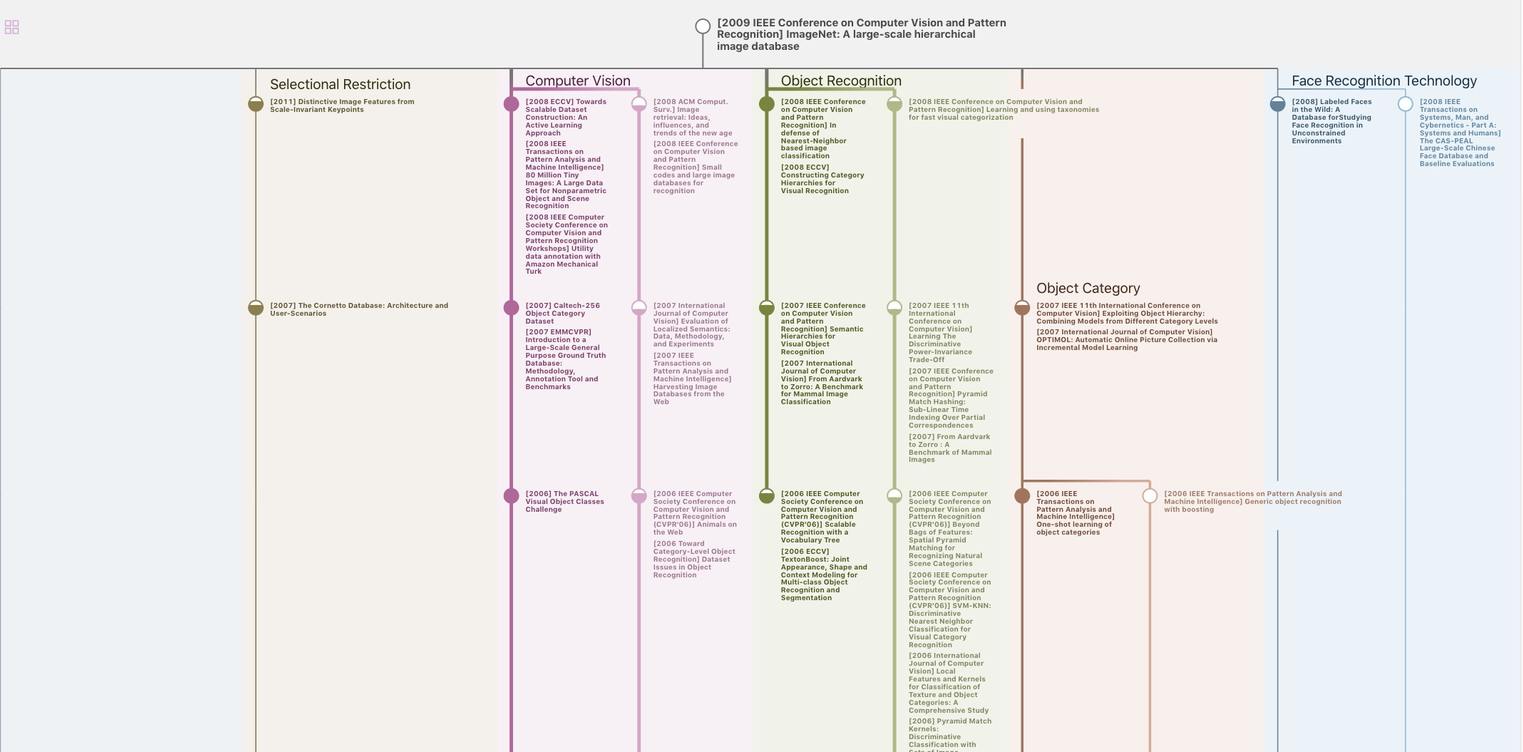
生成溯源树,研究论文发展脉络
Chat Paper
正在生成论文摘要