Complexity Reduction of Learned In-Loop Filtering in Video Coding.
ISCAS(2022)
摘要
In video coding, in-loop filters are applied on reconstructed video frames to enhance their perceptual quality, before storing the frames for output. Conventional in? loop filters are obtained by hand-crafted methods. Recently, learned filters based on convolutional neural networks that utilize attention mechanisms have been shown to improve upon traditional techniques. However, these solutions are typically significantly more computationally expensive, limiting their potential for practical applications. The proposed method uses a novel combination of sparsity and structured pruning for complexity reduction of learned in-loop filters. This is done through a three-step training process of magnitude-guidedweight pruning, insignificant neuron identification and removal, and fine-tuning. Through initial tests we find that network parameters can be significantly reduced with a minimal impact on network performance.
更多查看译文
关键词
neural networks,video coding,in-loop filtering,quality enhancement,pruning,sparsity
AI 理解论文
溯源树
样例
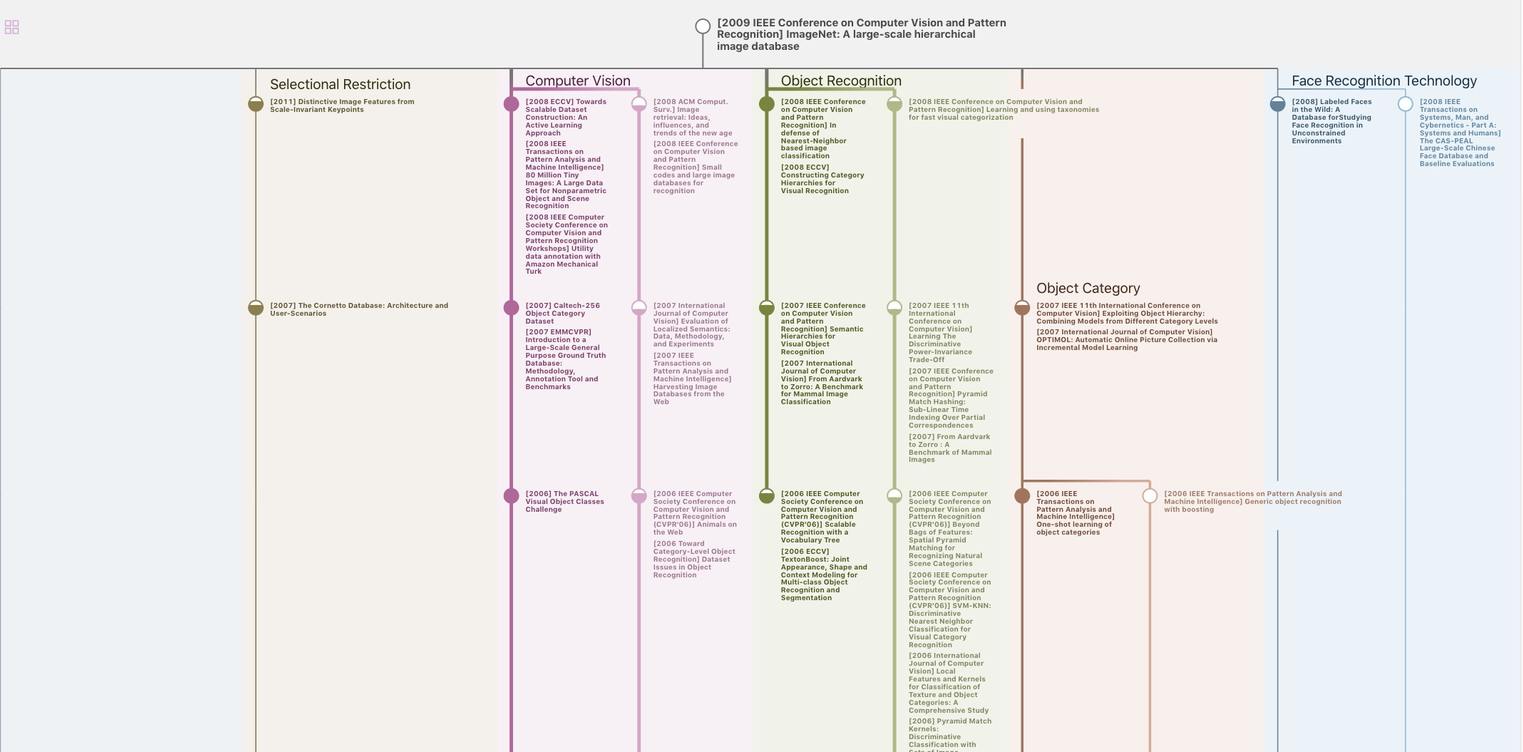
生成溯源树,研究论文发展脉络
Chat Paper
正在生成论文摘要