Capturing Humans in Motion: Temporal-Attentive 3D Human Pose and Shape Estimation from Monocular Video
IEEE Conference on Computer Vision and Pattern Recognition(2022)
摘要
Learning to capture human motion is essential to 3D human pose and shape estimation from monocular video. However, the existing methods mainly rely on recurrent or convolutional operation to model such temporal information, which limits the ability to capture non-local context relations of human motion. To address this problem, we propose a motion pose and shape network (MPS-Net) to effectively capture humans in motion to estimate accurate and temporally coherent 3D human pose and shape from a video. Specifically, we first propose a motion continuity attention (MoCA) module that leverages visual cues observed from human motion to adaptively recalibrate the range that needs attention in the sequence to better capture the motion continuity dependencies. Then, we develop a hierarchical attentive feature integration (HAFI) module to effectively combine adjacent past and future feature represen-tations to strengthen temporal correlation and refine the feature representation of the current frame. By coupling the MoCA and HAFI modules, the proposed MPS-Net excels in estimating 3D human pose and shape in the video. Though conceptually simple, our MPS-Net not only outperforms the state-of-the-art methods on the 3DPW, MPI-INF-3DHP, and Human3.6M benchmark datasets, but also uses fewer network parameters. The video demos can be found at https://mps-net.github.io/MPS-Net/.
更多查看译文
关键词
Pose estimation and tracking, 3D from single images, Motion and tracking
AI 理解论文
溯源树
样例
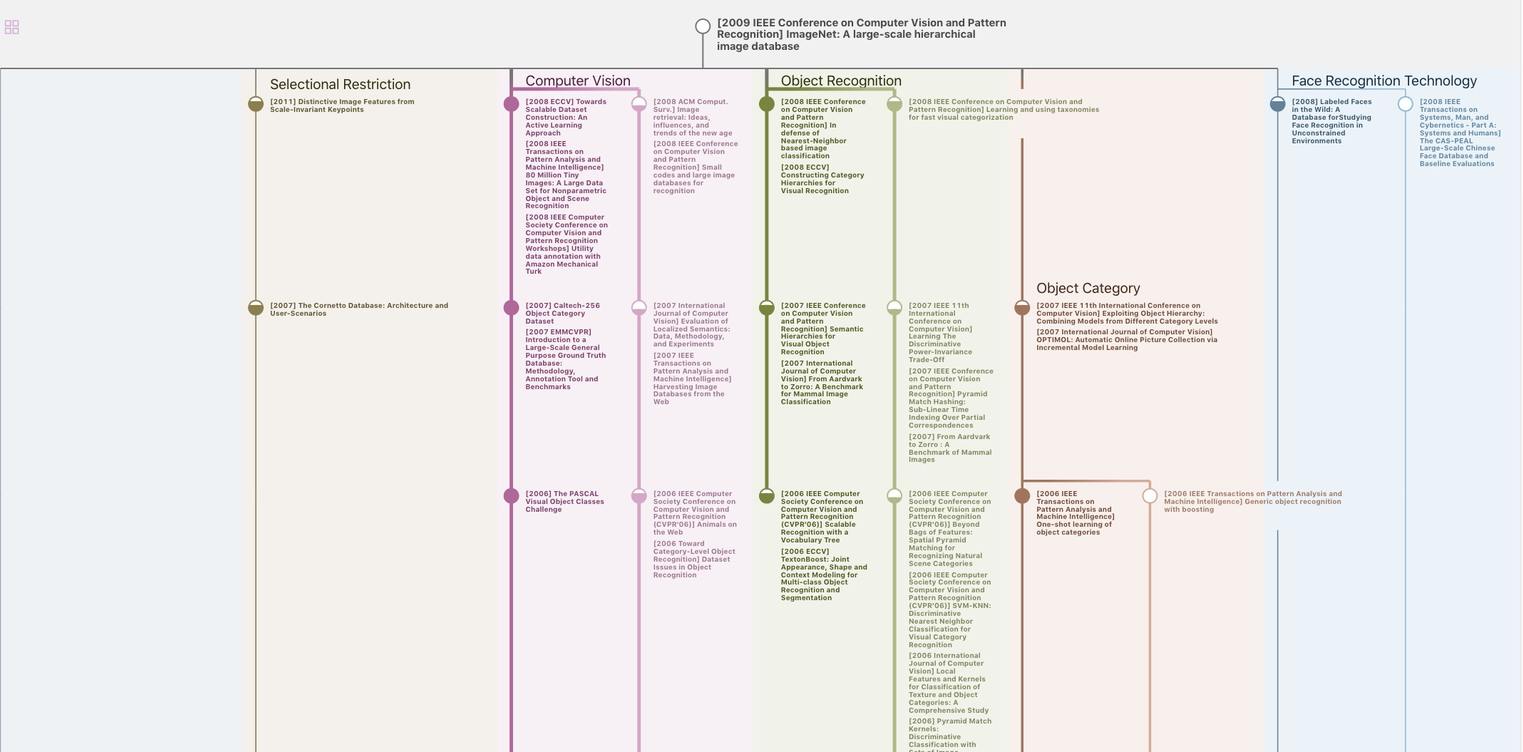
生成溯源树,研究论文发展脉络
Chat Paper
正在生成论文摘要