Predicting Value at Risk for Cryptocurrencies With Generalized Random Forests
arxiv(2022)
摘要
We study the prediction of Value at Risk (VaR) for cryptocurrencies. In contrast to classic assets, returns of cryptocurrencies are often highly volatile and characterized by large fluctuations around single events. Analyzing a comprehensive set of 105 major cryptocurrencies, we show that Generalized Random Forests (GRF) (Athey et al., 2019) adapted to quantile prediction have superior performance over other established methods such as quantile regression, GARCH-type and CAViaR models. This advantage is especially pronounced in unstable times and for classes of highly-volatile cryptocurrencies. Furthermore, we identify important predictors during such times and show their influence on forecasting over time. Moreover, a comprehensive simulation study also indicates that the GRF methodology is at least on par with existing methods in VaR predictions for standard types of financial returns and clearly superior in the cryptocurrency setup.
更多查看译文
关键词
cryptocurrencies,predicting,risk,generalized random
AI 理解论文
溯源树
样例
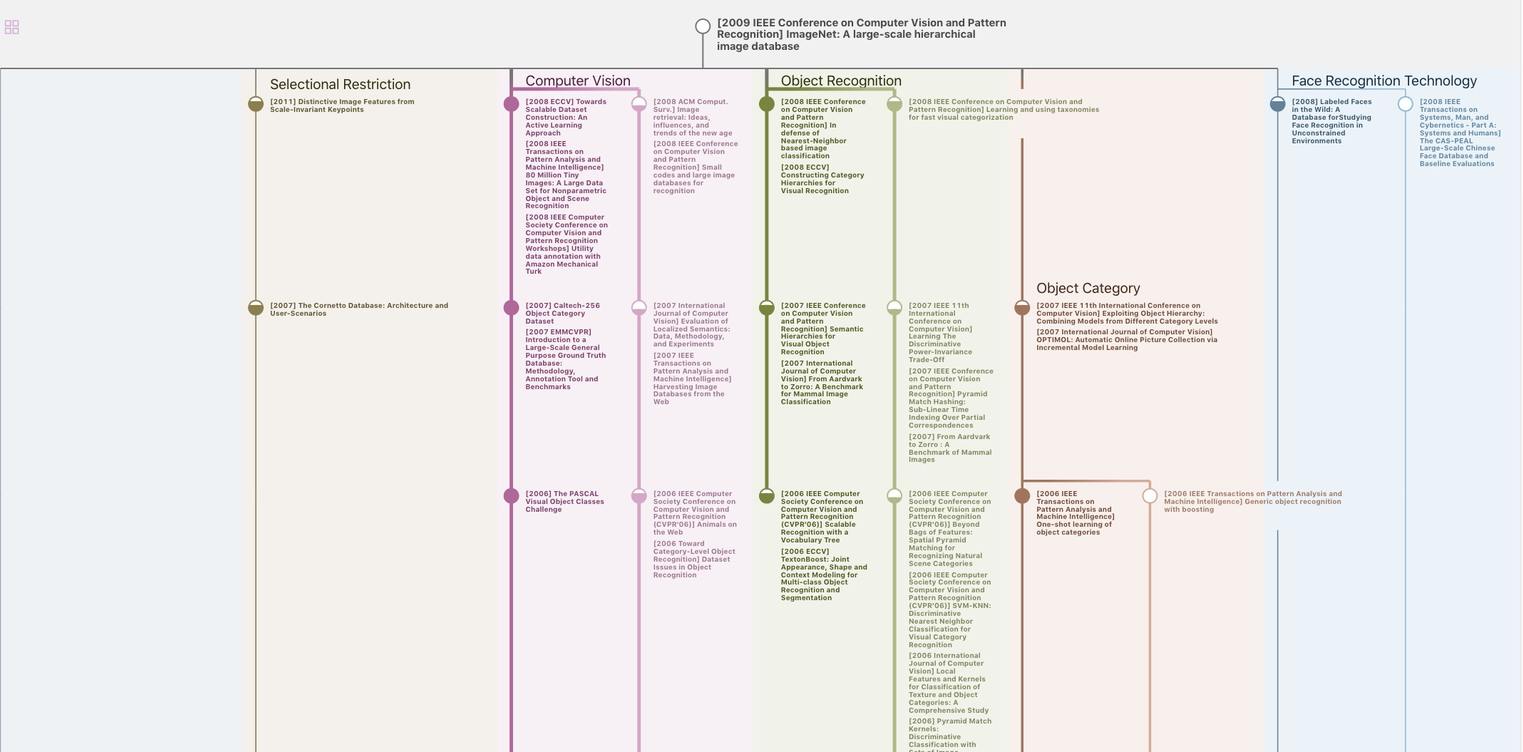
生成溯源树,研究论文发展脉络
Chat Paper
正在生成论文摘要