Animatable Implicit Neural Representations for Creating Realistic Avatars from Videos.
arxiv(2024)
摘要
This paper addresses the challenge of reconstructing an animatable human model from a multi-view video. Some recent works have proposed to decompose a non-rigidly deforming scene into a canonical neural radiance field and a set of deformation fields that map observation-space points to the canonical space, thereby enabling them to learn the dynamic scene from images. However, they represent the deformation field as translational vector field or SE(3) field, which makes the optimization highly under-constrained. Moreover, these representations cannot be explicitly controlled by input motions. Instead, we introduce blend weight fields to produce the deformation fields. Based on the skeleton-driven deformation, blend weight fields are used with 3D human skeletons to generate observation-to-canonical and canonical-to-observation correspondences. Since 3D human skeletons are more observable, they can regularize the learning of deformation fields. Moreover, the blend weight fields can be combined with input skeletal motions to generate new deformation fields to animate the human model. To improve the quality of human modeling, we further represent the human geometry as a signed distance field in the canonical space. Additionally, a neural point displacement field is introduced to enhance the capability of the blend weight field on modeling detailed human motions. Experiments show that our approach significantly outperforms recent human modeling methods. The code is available at https://zju3dv.github.io/animatable nerf/.
更多查看译文
关键词
Human Modeling,Implicit Neural Representations,View Synthesis
AI 理解论文
溯源树
样例
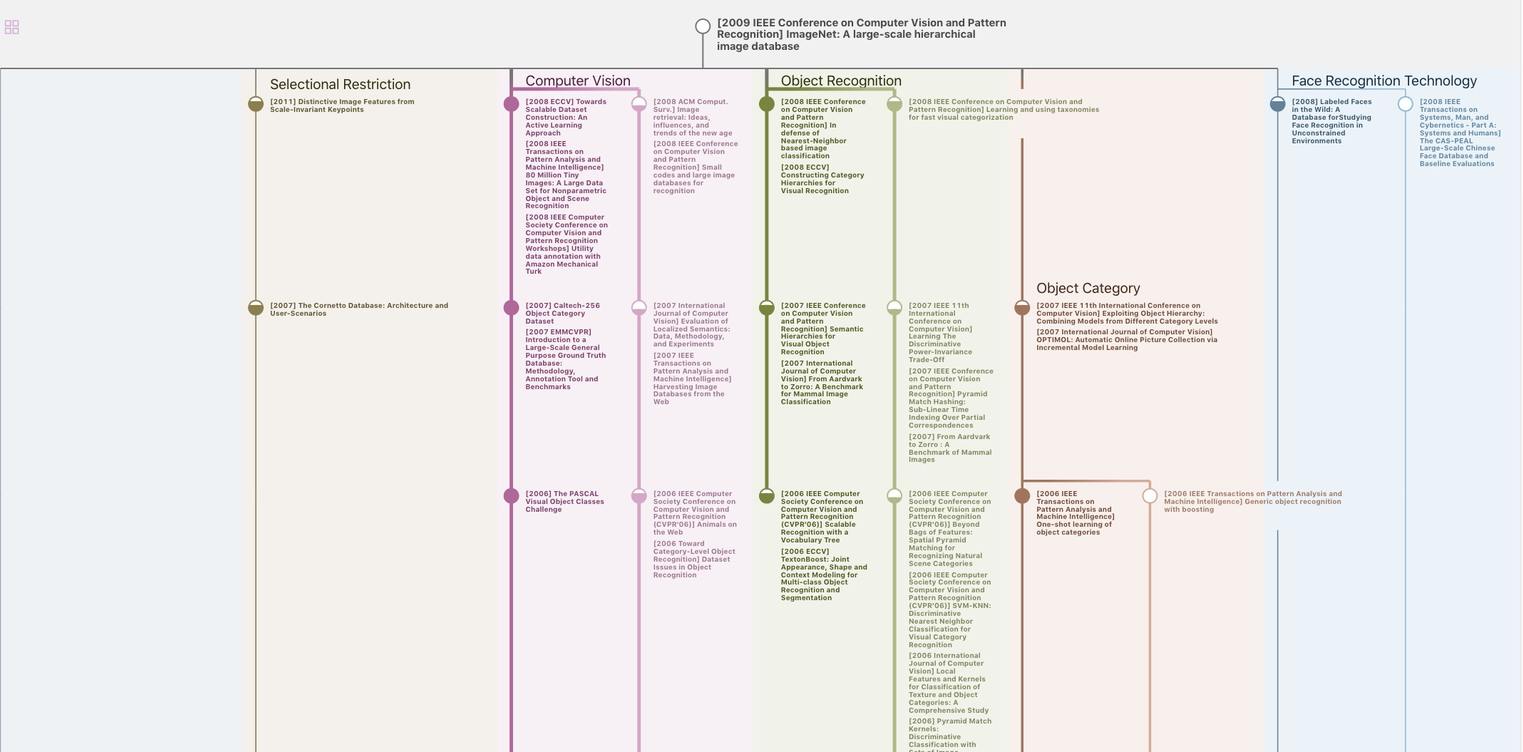
生成溯源树,研究论文发展脉络
Chat Paper
正在生成论文摘要