Adversarial Robustness of Neural-Statistical Features in Detection of Generative Transformers
IEEE International Joint Conference on Neural Network (IJCNN)(2022)
摘要
The detection of computer-generated text is an area of rapidly increasing significance as nascent generative models allow for efficient creation of compelling human-like text, which may be abused for the purposes of spam, disinformation, phishing, or online influence campaigns. Past work has studied detection of current state-of-the-art models, but despite a developing threat landscape, there has been minimal analysis of the robustness of detection methods to adversarial attacks. To this end, we evaluate neural and non-neural approaches on their ability to detect computer-generated text, their robustness against text adversarial attacks, and the impact that successful adversarial attacks have on human judgement of text quality. We find that while statistical features underperform neural features, statistical features provide additional adversarial robustness that can be leveraged in ensemble detection models. In the process, we find that previously effective complex phrasal features for detection of computer-generated text hold little predictive power against contemporary generative models, and identify promising statistical features to use instead. Finally, we pioneer the usage of $\Delta$MAUVE as a proxy measure for human judgement of adversarial text quality.
更多查看译文
关键词
transformer,neural networks,cybersecurity,natural language processing,phishing,adversarial attacks
AI 理解论文
溯源树
样例
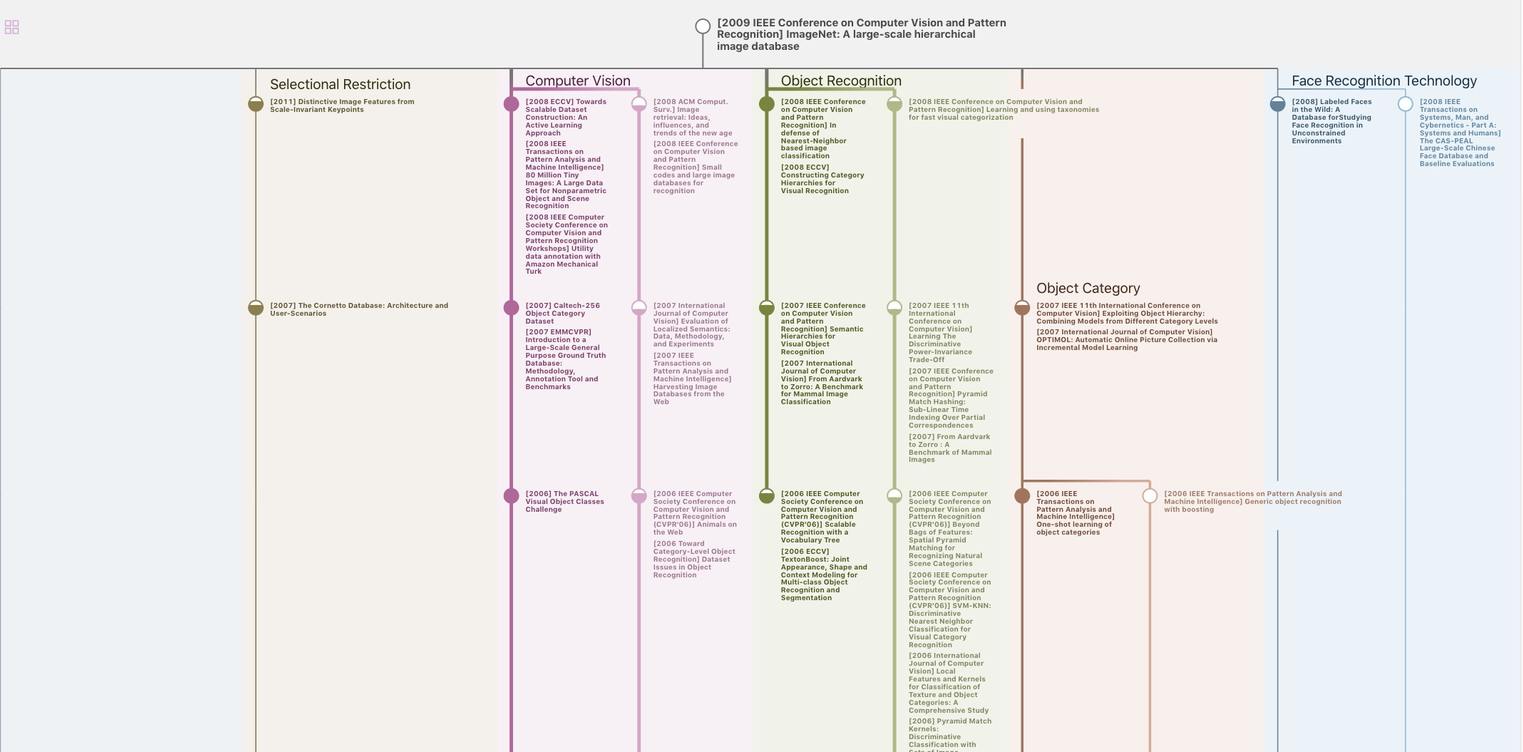
生成溯源树,研究论文发展脉络
Chat Paper
正在生成论文摘要