Style Transformer for Image Inversion and Editing
IEEE Conference on Computer Vision and Pattern Recognition(2022)
摘要
Existing GAN inversion methods fail to provide latent codes for reliable reconstruction and flexible editing simultaneously. This paper presents a transformer-based image inversion and editing model for pretrained StyleGAN which is not only with less distortions, but also of high quality and flexibility for editing. The proposed model employs a CNN encoder to provide multi-scale image features as keys and values. Meanwhile it regards the style code to be determined for different layers of the generator as queries. It first initializes query tokens as learnable parameters and maps them into W+ space. Then the multi-stage alternate self-and cross-attention are utilized, updating queries with the purpose of inverting the input by the generator. Moreover, based on the inverted code, we investigate the reference-and label-based attribute editing through a pretrained latent classifier, and achieve flexible image-to-image translation with high quality results. Extensive experiments are carried out, showing better performances on both inversion and editing tasks within StyleGAN. Codes are available at https://github.com/sapphire497/style-transformer.
更多查看译文
关键词
Image and video synthesis and generation, Deep learning architectures and techniques, Face and gestures
AI 理解论文
溯源树
样例
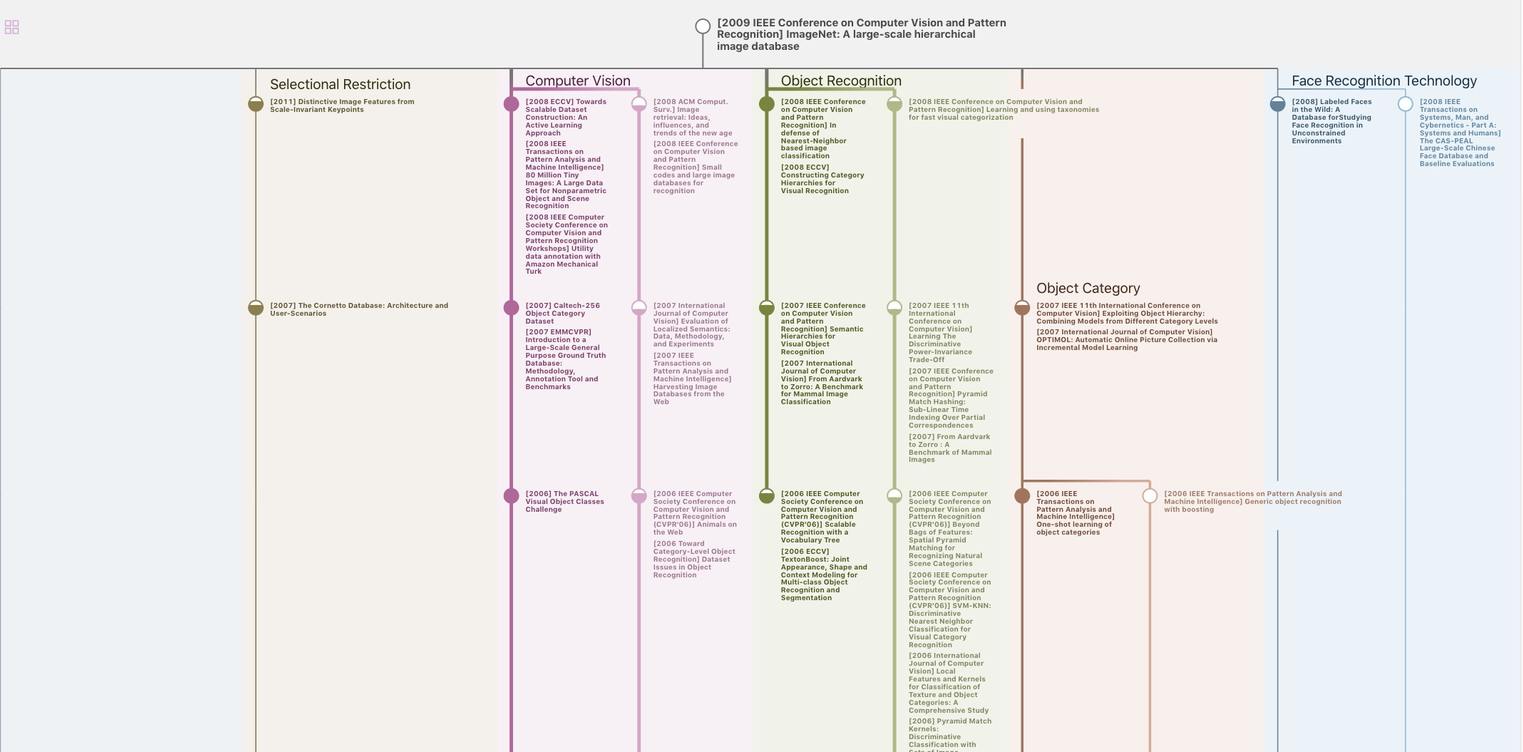
生成溯源树,研究论文发展脉络
Chat Paper
正在生成论文摘要