Graph Pre-training for AMR Parsing and Generation
PROCEEDINGS OF THE 60TH ANNUAL MEETING OF THE ASSOCIATION FOR COMPUTATIONAL LINGUISTICS (ACL 2022), VOL 1: (LONG PAPERS)(2022)
摘要
. Abstract meaning representation (AMR) highlights the core semantic information of text in a graph structure. Recently, pre-trained language models (PLMs) have advanced tasks of AMR parsing and AMR-to-text generation, respectively. However, PLMs are typically pre-trained on textual data, thus are sub-optimal for modeling structural knowledge. To this end, we investigate graph self-supervised training to improve the structure awareness of PLMs over AMR graphs. In particular, we introduce two graph auto-encoding strategies for graph-to-graph pre-training and four tasks to integrate text and graph information during pre-training. We further design a unified framework to bridge the gap between pre-training and fine-tuning tasks. Experiments on both AMR parsing and AMR-to-text generation show the superiority of our model. To our knowledge, we are the first to consider pre-training on semantic graphs.
更多查看译文
关键词
amr parsing,pre-training
AI 理解论文
溯源树
样例
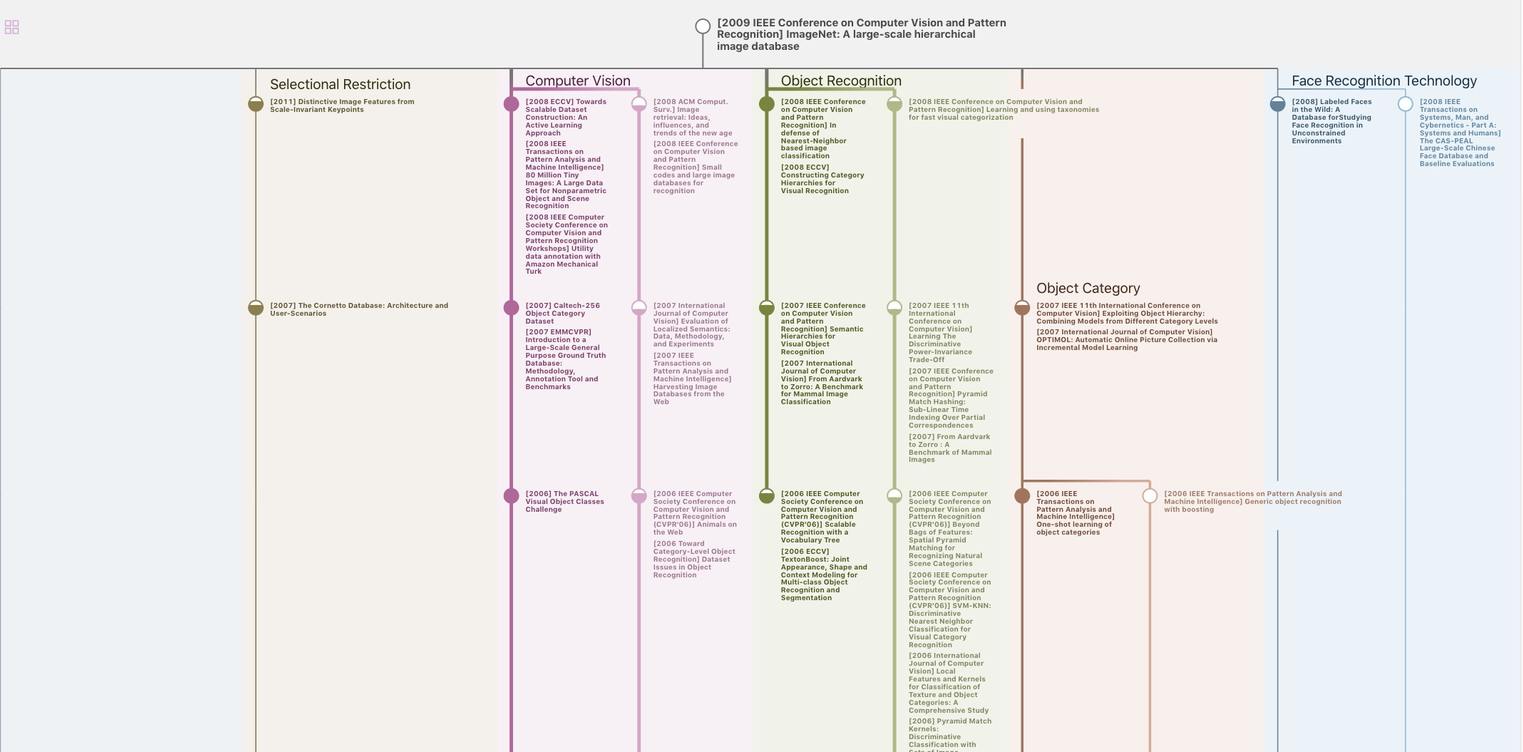
生成溯源树,研究论文发展脉络
Chat Paper
正在生成论文摘要