Improving Event Representation via Simultaneous Weakly Supervised Contrastive Learning and Clustering
PROCEEDINGS OF THE 60TH ANNUAL MEETING OF THE ASSOCIATION FOR COMPUTATIONAL LINGUISTICS (ACL 2022), VOL 1: (LONG PAPERS)(2022)
摘要
Representations of events described in text are important for various tasks. In this work, we present SWCC: a Simultaneous Weakly supervised Contrastive learning and Clustering framework for event representation learning. SWCC learns event representations by making better use of co-occurrence information of events. Specifically, we introduce a weakly supervised contrastive learning method that allows us to consider multiple positives and multiple negatives, and a prototype-based clustering method that avoids semantically related events being pulled apart. For model training, SWCC learns representations by simultaneously performing weakly supervised contrastive learning and prototype-based clustering. Experimental results show that SWCC outperforms other baselines on Hard Similarity and Transitive Sentence Similarity tasks. In addition, a thorough analysis of the prototypebased clustering method demonstrates that the learned prototype vectors are able to implicitly capture various relations between events.
更多查看译文
关键词
simultaneous weakly supervised
AI 理解论文
溯源树
样例
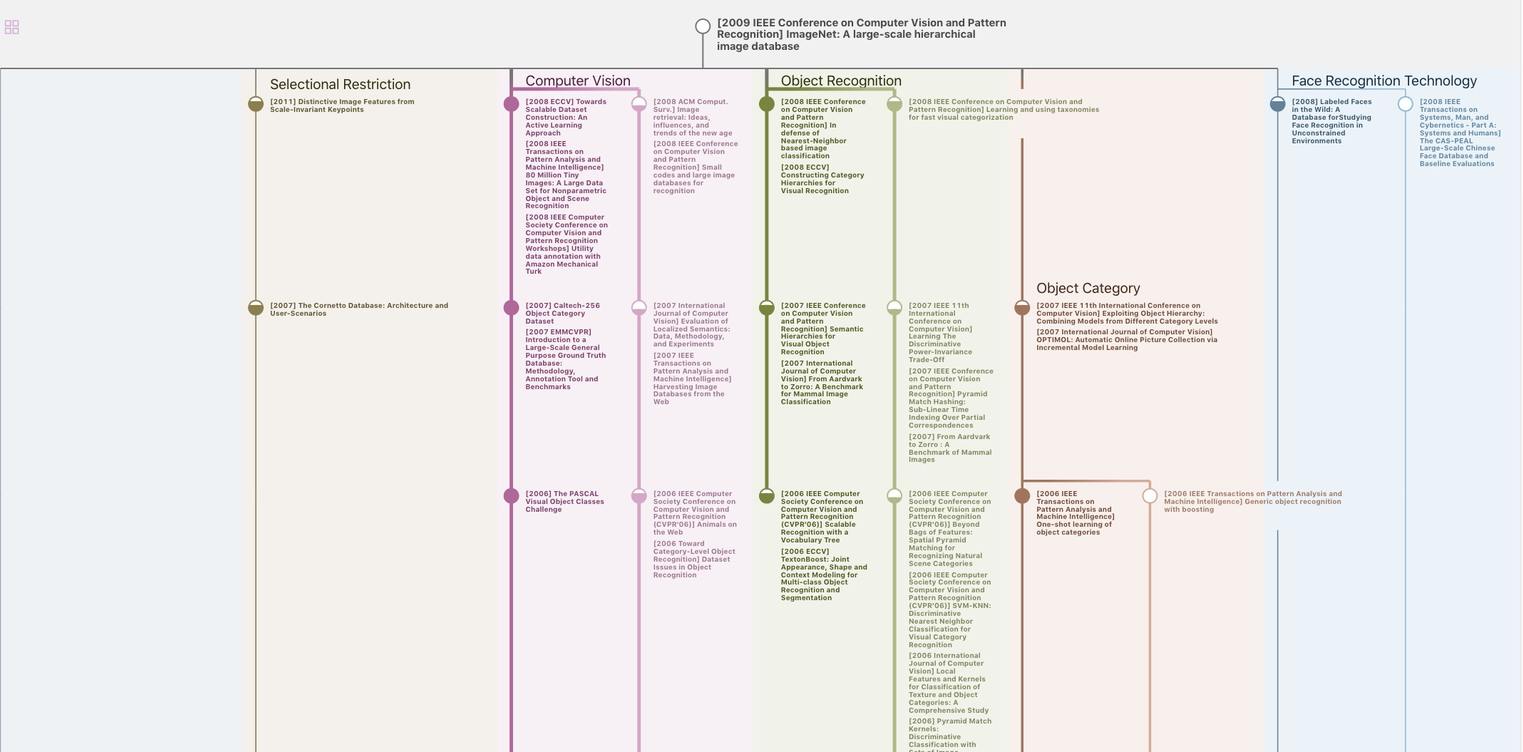
生成溯源树,研究论文发展脉络
Chat Paper
正在生成论文摘要