Don’t fear the unlabelled: safe semi-supervised learning via debiasing
arxiv(2023)
摘要
Semi-supervised learning (SSL) provides an effective means of leveraging unlabelled data to improve a model’s performance. Even though the domain has received a considerable amount of attention in the past years, most methods present the common drawback of lacking theoretical guarantees. Our starting point is to notice that the estimate of the risk that most discriminative SSL methods minimise is biased, even asymptotically. This bias impedes the use of standard statistical learning theory and can hurt empirical performance. We propose a simple way of removing the bias. Our debiasing approach is straightforward to implement and applicable to most deep SSL methods. We provide simple theoretical guarantees on the trustworthiness of these modified methods, without having to rely on the strong assumptions on the data distribution that SSL theory usually requires. In particular, we provide generalisation error bounds for the proposed methods. We evaluate debiased versions of different existing SSL methods, such as the Pseudo-label method and Fixmatch, and show that debiasing can compete with classic deep SSL techniques in various settings by providing better calibrated models. Additionally, we provide a theoretical explanation of the intuition of the popular SSL methods.
更多查看译文
关键词
Semi-supervised learning,deep learning,empirical risk minimisation,control variate,variance reduction,asymptotic statistics
AI 理解论文
溯源树
样例
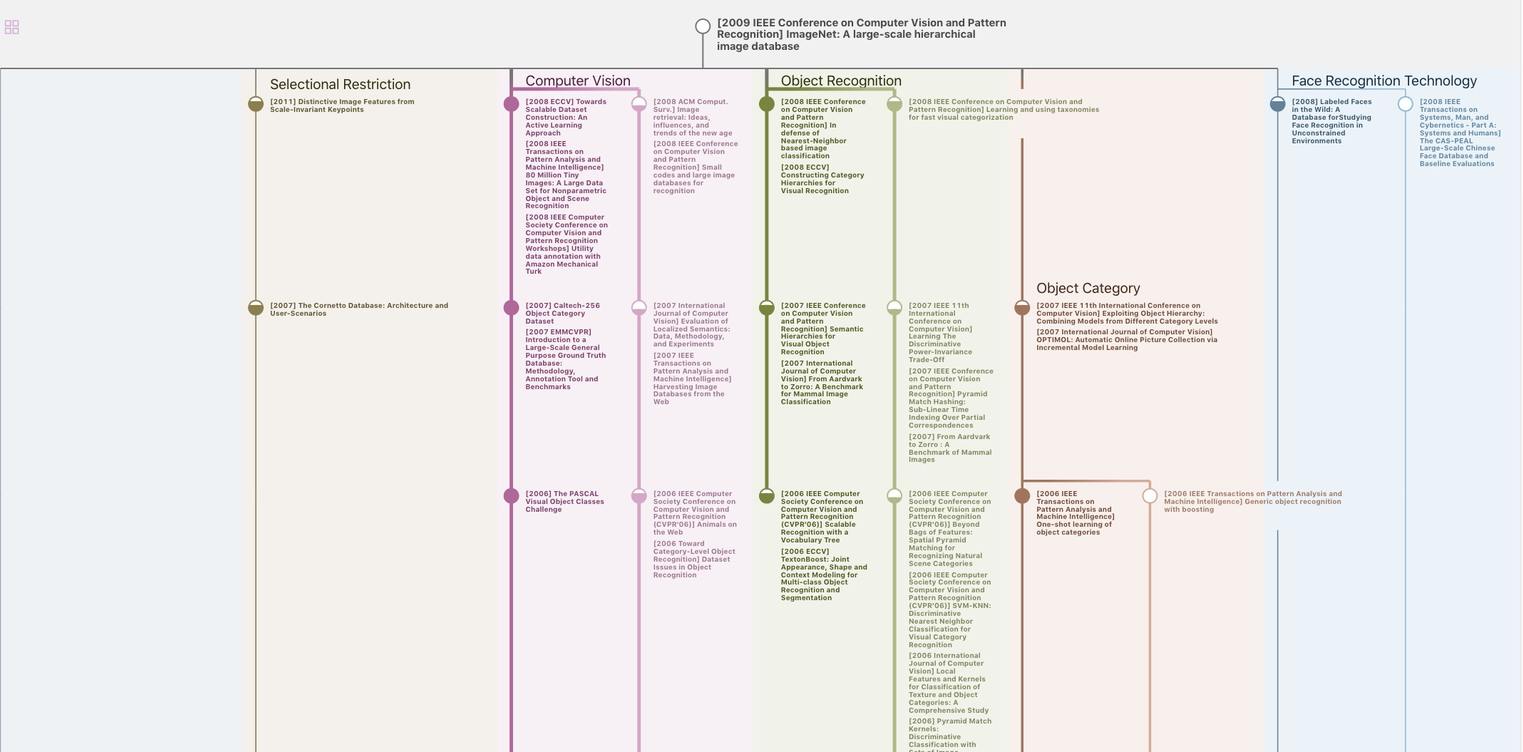
生成溯源树,研究论文发展脉络
Chat Paper
正在生成论文摘要