Potential of multiparametric characterization of foodstuffs by nuclear magnetic resonance to better predict microbial behavior
MAGNETIC RESONANCE IN CHEMISTRY(2022)
摘要
Numerous predictive microbiology models have been proposed to describe bacterial population behaviors in foodstuffs. These models depict the growth kinetics of particular bacterial strains based on key physico-chemical parameters of food matrices and their storage temperature. In this context, there is a prominent issue to accurately characterize these parameters, notably pH, water activity (a(w)), and NaCl and organic acid concentrations. Usually, all these product features are determined using one destructive analysis per parameter at macroscale (>5 g). Such approach prevents an overall view of these characteristics on a single sample. Besides, it does not take into account the intra-product microlocal variability of these parameters within foods. Nuclear magnetic resonance (NMR) is a versatile non-invasive spectroscopic technique. Experiments can be recorded successively on a same collected sample without damaging it. In this work, we designed a dedicated NMR approach to characterize the microenvironment of foods using 10-mg samples. The multiparametric mesoscopic-scale approach was validated on four food matrices: a smear soft cheese, cooked peeled shrimps, cold-smoked salmon, and smoked ham. Its implementation in situ on salmon fillets enabled to observe the intra-product heterogeneity and to highlight the impact of process on the spatial distribution of pH, NaCl, and organic acids. This analytical development and its successful application can help address the shortcomings of monoparametric methods traditionally used for predictive microbiology purposes.
更多查看译文
关键词
cold-smoked salmon, individual-based modeling, intra-product physico-chemical variability, mesoscopic scale, non-destructive analysis, spatial distribution
AI 理解论文
溯源树
样例
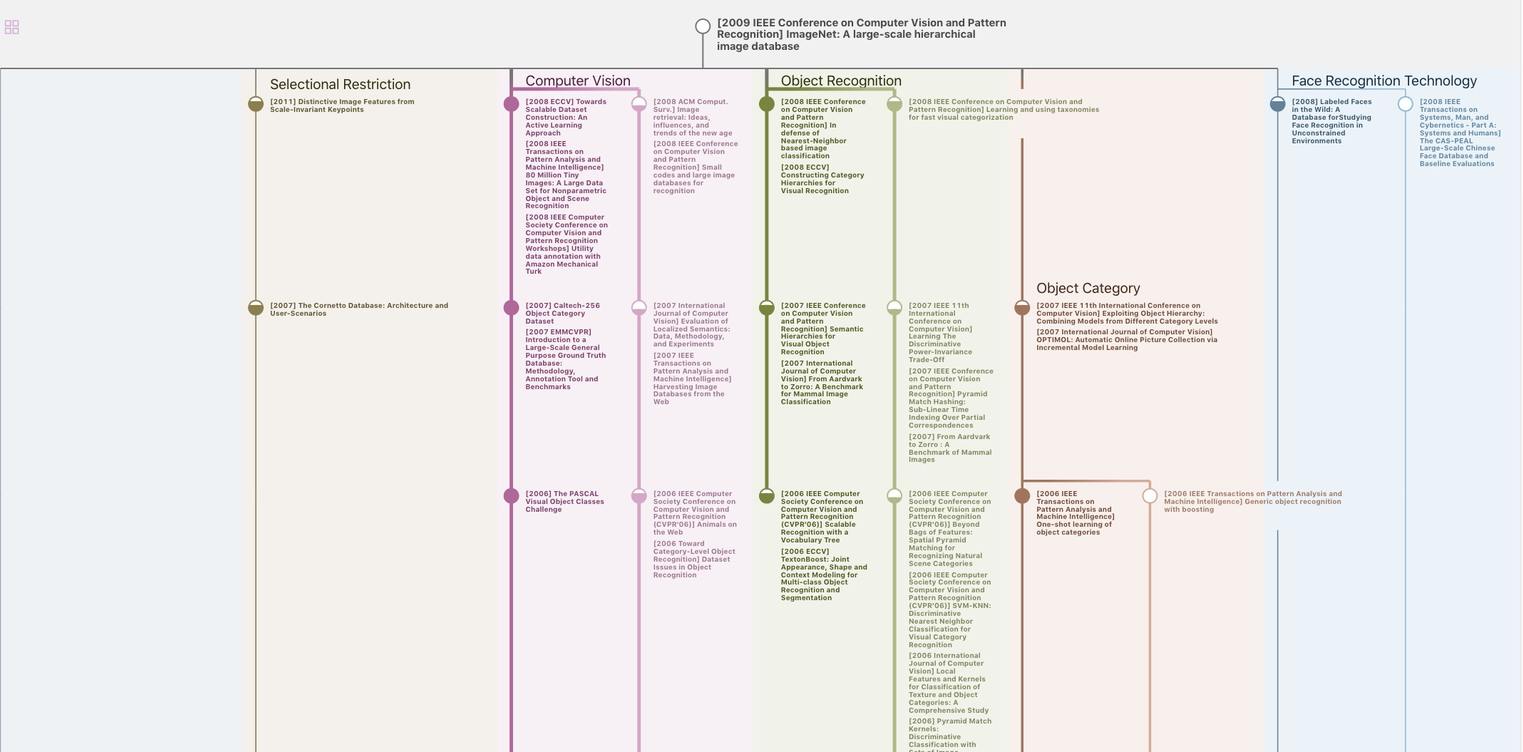
生成溯源树,研究论文发展脉络
Chat Paper
正在生成论文摘要