Protein-Ligand Interaction Graphs: Learning from Ligand-Shaped 3D Interaction Graphs to Improve Binding Affinity Prediction
biorxiv(2022)
摘要
Graph Neural Networks (GNNs) have recently gained in popularity, challenging molecular fingerprints or SMILES-based representations as the predominant way to represent molecules for binding affinity prediction. Although simple ligand-based graphs alone are already useful for affinity prediction, better performance on multi-target datasets has been achieved with models that incorporate 3D structural information. Most recent advances utilize complex GNN architectures to capture 3D protein-ligand information by incorporating ligand-interacting protein atoms as additional nodes in the graphs; or by building a second protein-based graph in parallel. This expands the graph considerably while obfuscating the shape of the underlying ligand, diminishing the advantage that GNNs have when encoding molecular structures. There is therefore a need for a simple and elegant molecular graph representation that retains the topology of the ligand while simultaneously encoding 3D protein-ligand interactions.
We present Protein-Ligand Interaction Graphs (PLIGs): a simple way of representing atom-atom contacts of 3D protein-ligand complexes as node features for GNNs. PLIGs featurize an atom node in the molecular graph by describing each atom’s properties as well as all atom-atom contacts made with protein atoms within a distance threshold. The edges of the graph are therefore identical to ligand-based graphs, but the nodes encode the 3D protein-ligand contacts. Since PLIGs are applicable to any GNN architecture, we have benchmarked their performance with six different GNN architectures, and compared them to conventional ligand-based graphs and fingerprint-based multi-layer perceptron (MLP) models using the CASF-2016 benchmark set where we found PLIG-based Graph Attention Networks (GATNet) to be the best performing model ( ρ =0.84, RMSE=1.22 pK). In summary, we created a novel graph-based representation that incorporates 3D structural information into the node features of ligand-shaped molecular graphs. The PLIG representation is simple, elegant, flexible and easily customizable, opening up many possibilities of incorporating other 2D and 3D properties into the graph.
Access The code and implementation for PLIGs and all models can be found at [github.com/MarcMoesser/Protein-Ligand-Interaction-Graphs][1].
### Competing Interest Statement
C.M.D. is employed by Excientia PLC.
[1]: http://github.com/MarcMoesser/Protein-Ligand-Interaction-Graphs
更多查看译文
关键词
binding affinity prediction,3d interaction graphs,protein-ligand,ligand-shaped
AI 理解论文
溯源树
样例
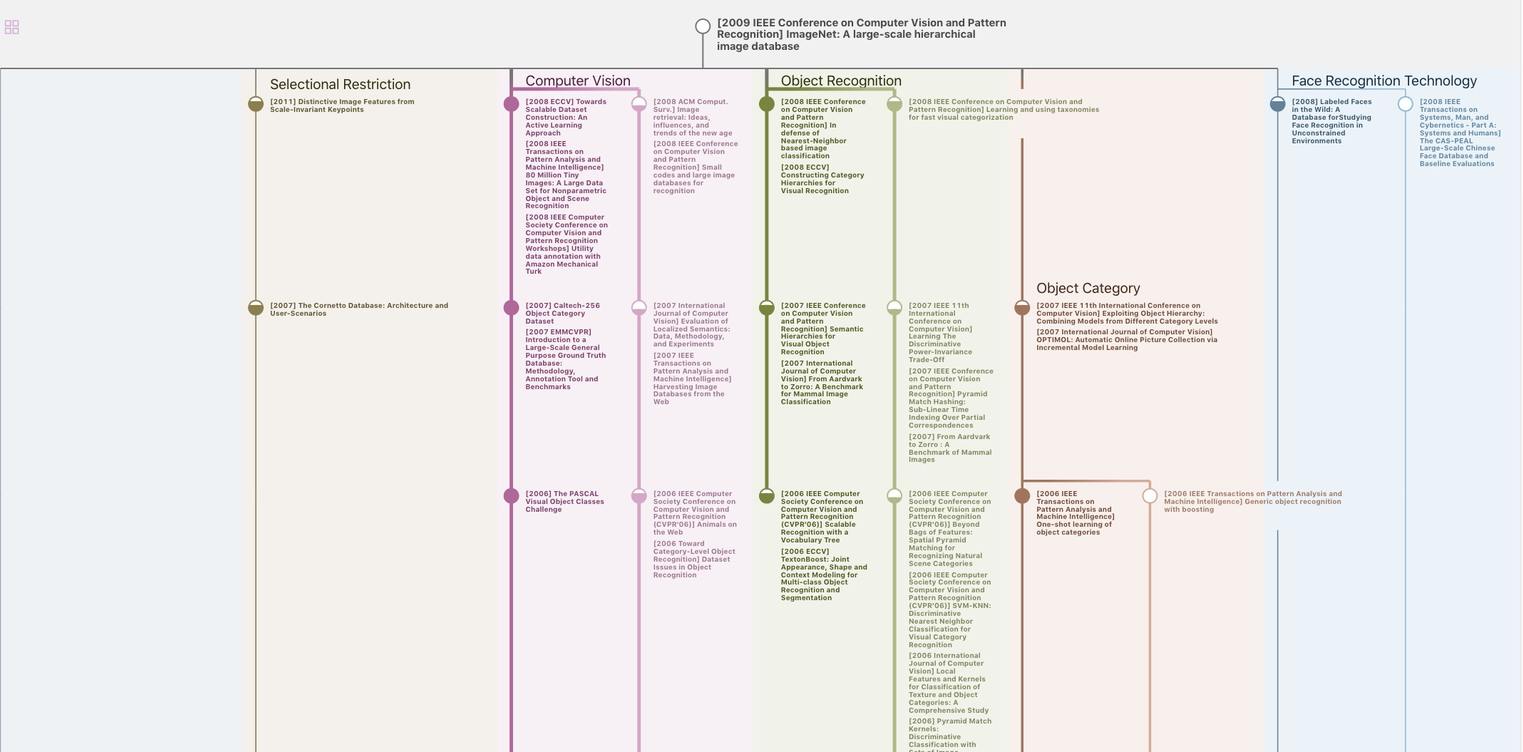
生成溯源树,研究论文发展脉络
Chat Paper
正在生成论文摘要