A reduced-memory multi-layer perceptron with systematic network weights generated and trained through distribution hyper-parameters
biorxiv(2022)
摘要
A multi-layer perceptron (MLP) consists of a number of forward-connected weights ( W ijk ) from each feeding layer node ( n ij ) to the many initially equivalent nodes ( n i +1, k ) in the next layer. Exact a priori order and search space of these weights ( W ijk ) is random and prone to redundancy, irreproducibility and non-optimality. We demonstrate that a weight subspace ( W ijk for each i and j ), generated systematically using a statistical distribution with predefined breakpoints and Genetic algorithm-trained hyper-parameters substantially reduces the computational complexity of an MLP and produces comparable or better performance than similarly trained equivalent models with fully defined weights. This distribution based neural network (DBNN) provides a novel framework to create very large neural network models with currently prohibitive memory requirements.
### Competing Interest Statement
The authors have declared no competing interest.
更多查看译文
关键词
systematic network weights,reduced-memory,multi-layer,hyper-parameters
AI 理解论文
溯源树
样例
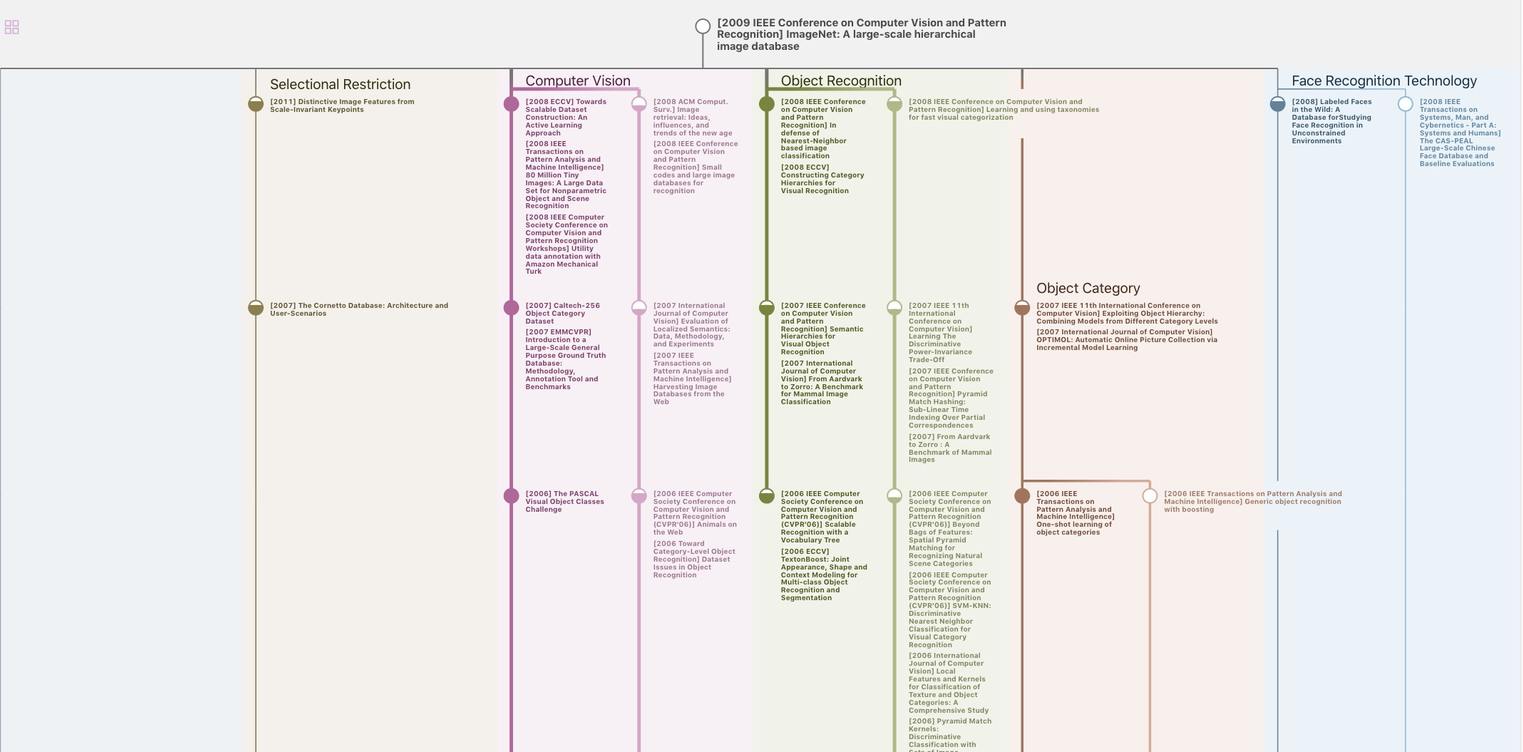
生成溯源树,研究论文发展脉络
Chat Paper
正在生成论文摘要